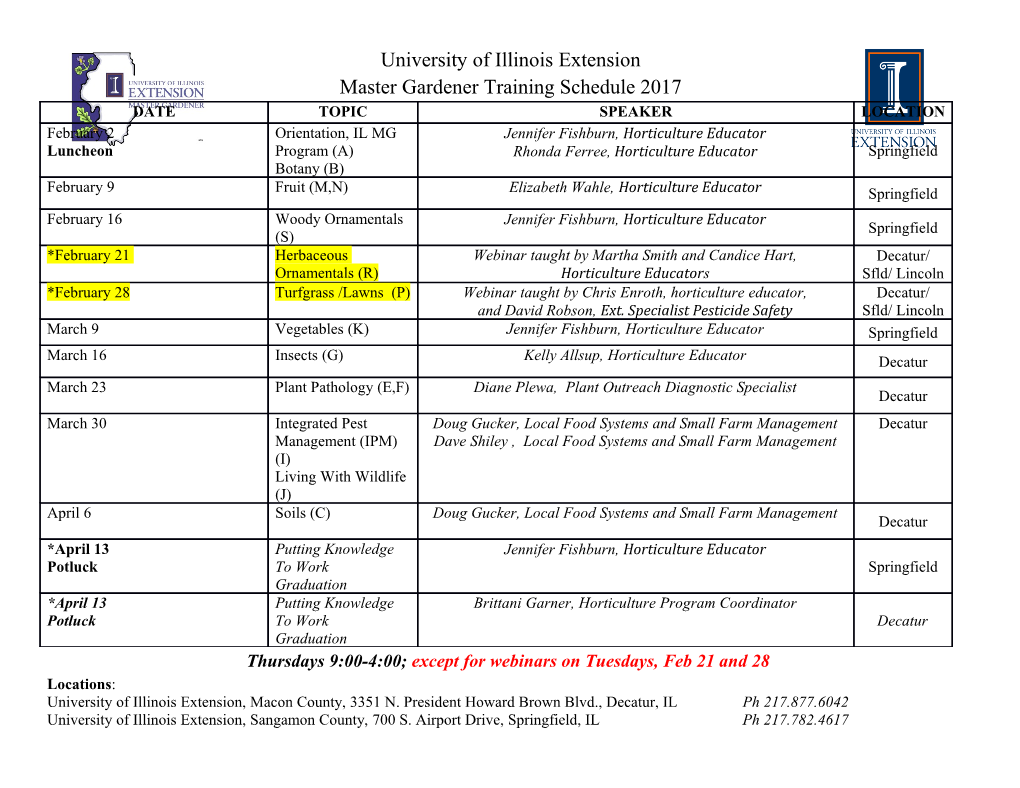
Algebraic statistics in Design of Experiments Maria Piera Rogantin DIMA – Universit`adi Genova – [email protected] Torino, September 2004 3. Indicator function and orthogo- Table of contents nality (a) Orthogonality 1. General aspects (b) Complex coding for full factorial (a) What is the Design of experiments? designs (b) Design & Ideal of the design (c) Indicator function of a fraction (c) The full factorial design (d) Results about orthogonality 2. Fractions and confounding (e) Generation of fractions with a given orthogonal structure (a) Fraction of a full factorial design (f) Regular fractions (b) Space of the responses on the fraction 4. Models on a fraction and term- orders (c) Identifiability of a model (a) Identifiable sub-models and initial (d) Fractions and identifiable linear orders models (b) Model curvature (e) Confounding of subspaces (c) Block term-order and factor screening 1 PART I: General aspects Pistone G., Wynn H. P.(1996). Generalized confounding with Gr¨obner bases, Biometrika, 83(1): 653–666. Robbiano L. (1998). Gr¨obner Bases and Statistics, Gr¨obner Bases and Applications (Proc. of the Conf. 33 Years of Gr¨obner Bases), Buchberg- er, B. & Winkler, F. ed., Cambridge University Press, 179–204. Pistone G., Riccomagno E. and Wynn H. P. (2001). Algebraic Statistics: Computational Commutative Algebra in Statistics, Chapman&Hall. 2 1.1 What is the Design of experiments? ∗ An example: the factorial design Push-off force of a spark control valve. (Wu & Hamada. 2000. Experiments. Whiley & Son, p.247) Influence of three factors on the response: - weld time (0.3 - 0.5 - 0.7 seconds) - pressure (15 - 20 - 25 psi) - moisture (0.8 - 1.2 - 1.8 percent) Each factor has three levels. We consider them as ordinal levels: low - medium - high; The levels are coded by integer numbers. full factorial design Each treatment corresponds to a point in Z3. ∗Full Design 3 In particular, the experiment is realized on fewer treatments ∗ (because of problems with costs, times, practical constraints, setting the factors and measuring the responses . ) How to choose the treatments? Which fraction for “the best” study of the responses? time pressure moisture -1 -1 -1 -1 0 0 -1 1 1 0 -1 0 0 0 1 0 1 -1 1 -1 1 1 0 -1 1 1 0 fractional factorial design or fraction ∗Fraction 4 Design and functions on the design∗ Response Factors Push-off force (lb) Time Pressure Moisture 111.1 -1 -1 -1 131.0 -1 0 0 65.4 -1 1 1 125.5 0 -1 0 46.9 0 0 1 113.7 0 1 -1 72.5 1 -1 1 141.1 1 0 -1 134.2 1 1 0 Linear influence of the factors and their interactions: Force = θ0 + θ1 T + θ2 P + θ3 M + θ12 T · P + θ13 T · M + ... the θ’s signify the “importance” of the terms w.r.t. the response. ∗General design 5 Full factorial designs and fractions: notations • Ai = {aij : j = 1,...,ni} factors m m aij levels coded by rational numbers Q or complex numbers C m m m • D = A1 × . × Am ⊂ Q (or D ⊂ C ) with N = j=1 nj points full factorial design Q • A fraction is a subset F ⊂D; 6 Responses on a design f : D 7→ R (functions defined on D) ∗ “Design” indicates either “Full factorial design” or “Fractional factorial design” or ... • Xi : D ∋ (d1,...,dm) 7→ di projection, frequently called factor α α1 αm • X = X1 · · · Xm , αi <ni, i = 1,...,m α = (α1,...,αm) monomial responses or terms or interactions The term Xα has order k if in α there are k non-null values: Xα is an interaction of order k (binary case: order = degree) Definitions: 1 • Mean value of f on D, ED(f): ED(f) = #D d∈D f(d) P • A contrast is a response f such that ED(f) = 0. • Two responses f and g are orthogonal on D if ED(f g) = 0. ∗General design 7 The polynomial complete regression model∗ • L = {(α1,...,αm) : αi <ni, i =1,...,m} exponents (or logarithms) of all the interactions • complete regression model: For all di ∈ D and for the observed value yi α yi = θα X (di) αX∈L whit θα ∈ R or θα ∈ C. In vector notation, Y =(y1,...,yn) response measured on the points of D: α Y = θα X αX∈L α • Z = [X (d)]d∈D,α∈L matrix of the complete regression model • θ =(θα)α∈L vector of the coefficients Matrix notation of the complete regression model Y = Zθ ∗General design 8 On the full factorial design: the complete regression model is identifiable, i.e. there is a unique solution w.r.t. θ: θˆ = Z−1 Y (the matrix Z is a square full rank matrix) On a fraction: there is not a unique solution w.r.t. θ α (Z = [X (d)]d∈F,α∈L has less rows than columns) θˆ = (Z′Z)−Z′ Y 9 In general, for linear regression models of the form: Y = W θ + R where W is a matrix of “explicative variables” and R is a vector of residuals Even if θˆ = (W ′W )−W ′ Y is not unique, the approximation of the response Y through the “explicative variables” is always unique: Yˆ = W (W ′W )−W ′ Y Y R Yˆ is the orthogonal projec- tion of Y in the linear space Y=^ W ^θ = W (W'W)- W' Y = P Y generate by the columns of W . Wθ Moreover, if Y is a multivariate random variable with normal distribution Y ∼ N (W θ, σ2I) then θˆ = (W ′W )−W ′ Y is a solution of maximum likelihood. 10 Example: 2 × 3 full factorial design∗ -1 -1 -1 0 A = {−1, 1}, n =2 -1 1 1 1 D = A2 = {−1, 0, 1}, n2 =3 1 -1 1 0 1 1 2 2 monomial responses: 1, X1, X2,X2 , X1X2,X1X2 L = {(0, 0), (1, 0), (0, 1), (0, 2), (1, 1), (1, 2)} complete regression model: 2 2 Y = θ00 + θ10X1 + θ01X2 + θ02X2 + θ11X1X2 + θ12X1X2 2 2 1 X1 X2 X2 X1X2 X1X2 1 −1 −11 1 −1 1 −100 0 0 matrix of the complete regression model Z = 1 −1 1 1 −1 −1 1 1 −1 1 −1 1 1100 0 0 1111 1 1 ∗Full Design 12 1.2 Design and design ideal∗ The application of computational commutative algebra to the study of estimability, confounding on the fractions of factorial designs has been proposed by Pistone & Wynn (Biometrika 1996). 1st idea Each set of points D ⊆ Qm is the set of the solutions of a system of polynomial equations; we assume that each solution is simple. 2nd idea Each real function defined on D is a polynomial function with coefficients into the field of real number R. It is a restriction to D of a real polynomial. ∗General Design 13 A design D is a finite set of distinct points in a m-dimensional field km ∗ The defining ideal of the design (or design ideal) I(D) is the set of all polynomials on k[x1, . , xm] that vanish on D. • The design D is a variety • The design ideal I(D) is radical • I(D) is the intersection of the design ideals of each point of D ∗General design 14 Operations with designs & Operations with ideals∗ • Product of designs. m m D1 ⊂ k 1 D2 ⊂ k 2 I (D1 ×D2) =< I1, I2 > m +m D1 ×D2 ⊂ k 1 2 τ term ordering on k[x1, . , xm1+m2] τ1, τ2 t.o. restricted to k[x1, . , xm1] and k[x1, . , xm2] G1,τ1, G2,τ2, G-bases of I (D1), I (D2) G = g1, g2 | g1 ∈ G1,τ1 g2 ∈ G2,τ2 n o is G-basis of I (D1 ×D2) ∗General design 15 • Restriction of a design∗ m D ⊂ k I + J ideal in k[x1, . , xm] I = I(D) I + J = {f + g | f ∈ I g ∈ J} J ideal in k[x1, . , xm] Variety(I + J) = D∩ Variety(J) ∗General design 16 • Union of designs∗ m D1, D2 ⊂ k τ term ordering on k[x1, . , xm], m D1 ∪D2 ⊂ k G1, G2 G-bases of I (D1), I (D2) A G-basis of I (D1 ∪D2) is G = {g1g2 | g1 ∈ G1 g2 ∈ G22} ∗General design 17 1.3 The full factorial design.∗ Polynomial representation The full factorial design D corresponds to the set of solutions of the system 1 n1 = n1− k (X1 − a11) ··· (X1 − a1n1 ) = 0 X1 k=0 ψ1k X1 . (X2 − a21) ··· (X2 − a2n2 ) = 0 . . or . P . . n −1 (Xm − am1) ··· (Xm − amn ) = 0 nm m k m Xm = k=0 ψmk Xm P In the previous examples: 2 (X1 − 1)(X1 +1) = 0 X1 = 1 rewriting or 3 X2 (X2 − 1)(X2 +1) = 0 X = X2 rules 2 3 X1 (X1 − 1)(X1 +1) = 0 X1 = X1 rewriting 3 X2 (X2 − 1)(X2 +1) = 0 or X2 = X2 rules ( 1)( +1) = 0 3 = X3 X3 − X3 X3 X3 ∗Full Design 18 Space of the real functions on the full factorial design R(D)∗ For a full factorial design each function is represented in a unique way by an identified complete regression model (i.e. as a linear combination of constant, simple terms and interactions): R(D) = θ Xα , θ ∈ R α α αX∈L In general, for full factorial designs or fraction: • R(D) is a Hilbert vector space (classical results derive from this structure) 1 The scalar product is: <f,g >= ED(f g) = N d∈D f(d)g(d) P • R(D) is a ring (algebraic statistical approach) The products are reduced with the rules derived by the polynomial representation of the full factorial design: ni−1 ni k Xi = ψik Xi , ψik ∈ R for i = 1, .
Details
-
File Typepdf
-
Upload Time-
-
Content LanguagesEnglish
-
Upload UserAnonymous/Not logged-in
-
File Pages53 Page
-
File Size-