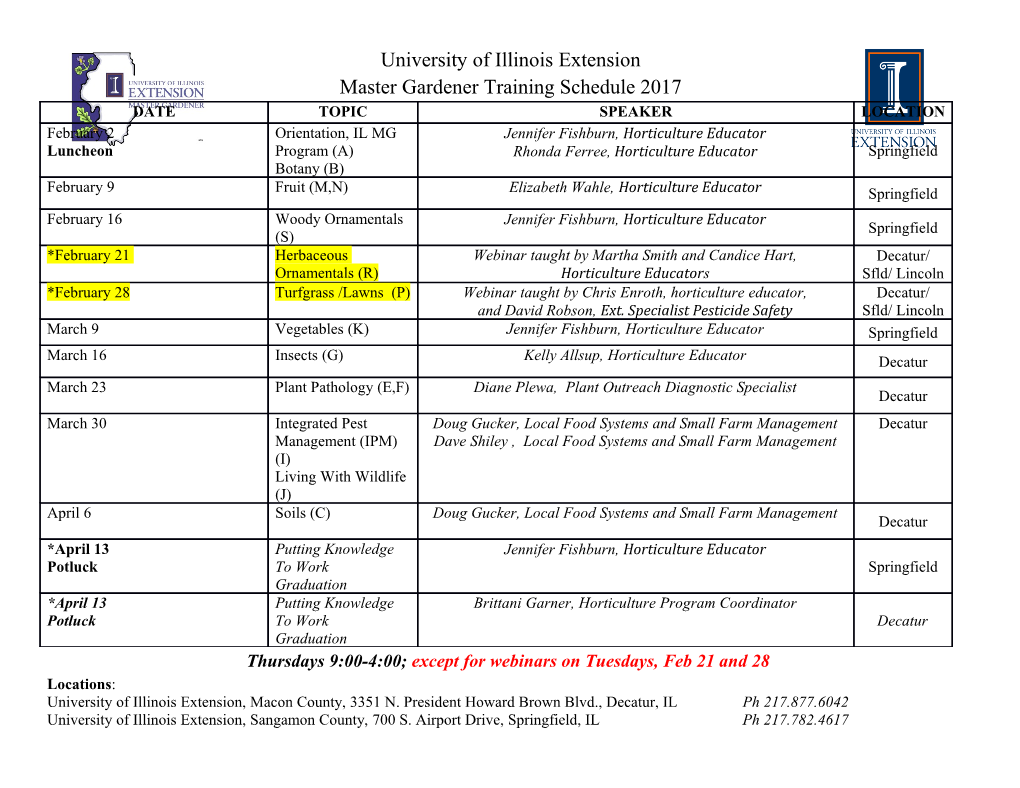
Proceedings of the Twenty-Sixth International Joint Conference on Artificial Intelligence (IJCAI-17) Student-t Process Regression with Student-t Likelihood Qingtao Tangy, Li Niuz, Yisen Wangy, Tao Daiy, Wangpeng Any, Jianfei Caiz, Shu-Tao Xiay y Department of Computer Science and Technology, Tsinghua University, China z School of Computer Science and Engineering, Nanyang Technological University, Singapore tqt15,dait14,wangys14,awp15 @mails.tsinghua.edu.cn; [email protected] f [email protected];g [email protected] Abstract distribution, GPR performs poorly on the data from heavy- tailed distributions or with outliers. However, real-world Gaussian Process Regression (GPR) is a powerful data often exhibit heavy-tailed phenomena [Nair et al., 2013] Bayesian method. However, the performance of and contain outliers [Bendre et al., 1994; Niu et al., 2015; GPR can be significantly degraded when the train- 2016]. ing data are contaminated by outliers, including In order to handle the outliers, heavy-tailed distribu- target outliers and input outliers. Although there tions (e.g., Laplace distribution, mixtures of Gaussians, and are some variants of GPR (e.g., GPR with Student-t Student-t distribution) have been introduced into GPR. In likelihood (GPRT)) aiming to handle outliers, most particular, Laplace noise is used in [Kuss, 2006] while mixed of the variants focus on handling the target outliers two forms of Gaussian corruption are used in [Naish-Guzman while little effort has been done to deal with the and Holden, 2008]. In [Neal, 1997; Vanhatalo et al., 2009; input outliers. In contrast, in this work, we aim to Jylanki¨ et al., 2011], the noise is assumed to follow the handle both the target outliers and the input outliers Student-t distribution (GPRT). However, all these methods at the same time. Specifically, we replace the Gaus- are only robust to the target outliers, but not robust to the sian noise in GPR with independent Student-t noise outliers in the inputs X, since the latent variables f(X) are to cope with the target outliers. Moreover, to en- still assumed to follow the Gaussian distribution. hance the robustness w.r.t. the input outliers, we use Related to the robustness w.r.t. the outliers in the inputs a Student-t Process prior instead of the common X, some works [Shah et al., 2014; Solin and Sarkk¨ a,¨ 2015; Gaussian Process prior, leading to Student-t Pro- Tang et al., 2016] rely on Student-t Process to handle the cess Regression with Student-t Likelihood (TPRT). input outliers. Particularly, the method in [Shah et al., 2014; We theoretically show that TPRT is more robust to Solin and Sarkk¨ a,¨ 2015] replaces the Gaussian Process with both input and target outliers than GPR and GPRT, the Student-t Process and incorporates the noise term into the and prove that both GPR and GPRT are special kernel function (TPRK) for computational simplicity. Fol- cases of TPRT. Various experiments demonstrate lowing [Shah et al., 2014; Solin and Sarkk¨ a,¨ 2015], an input that TPRT outperforms GPR and its variants on dependent Student-t noise (TPRD) is proposed in [Tang et both synthetic and real datasets. al., 2016]. Note that Tang et al. [2016] prove that TPRK, TPRD, and GPR have the same predictive mean if the kernel has a certain property named β property, which is actually 1 Introduction satisfied by most kernels. Taking the frequently used kernels Gaussian Process Regression (GPR) is a powerful Bayesian implemented by GPML [Rasmussen and Nickisch, 2010] (the method with good interpretability, non-parametric flexibil- most popular toolbox in Gaussian Process community) as ity, and simple hyper-parameter learning [Rasmussen, 2006]. examples, 24 out of 28 kernels have β property, for which Due to its nice properties, GPR has been successfully applied the above Student-t Process based methods (i.e., TPRK and to many fields, such as reinforcement learning [Rasmussen TPRD) have the same predictive value as GPR and thus fail et al., 2003], computer vision [Liu and Vasconcelos, 2015], to deal with the input outliers effectively. spatio-temporal modeling [Senanayake et al., 2016]. In this paper, with the aim to handle both the input outliers In GPR, the basic model is y = f(X) + , where y = and the target outliers at the same time, we propose Student- n n yi i=1 is the target vector, X = xi i=1 is the collection t Process Regression with Student-t Likelihood (TPRT). In off inputg vectors, and is the noise.f g The latent function our model, the latent function f is assumed to be a Student-t f is given a Gaussian Process prior and is assumed to Process prior while the noise is assumed to be an independent be independent and identically distributed (i.i.d.) Gaussian Student-t noise, instead of the noise incorporated into kernel noise. In practice, as the number of input vectors is finite, as in [Shah et al., 2014; Solin and Sarkk¨ a,¨ 2015] or dependent the latent variables f(X) follow a multivariate Gaussian noise as in [Tang et al., 2016]. In addition to owning all distribution. Due to the thin-tailed property of the Gaussian the advantages of GPR, such as good interpretability, non- 2822 Proceedings of the Twenty-Sixth International Joint Conference on Artificial Intelligence (IJCAI-17) parametric flexibility, and simple hyper-parameter learning, 2.2 Student-t Distribution and Student-t Process our proposed TPRT method is robust to both input and tar- get outliers, because the Student-t Process prior contributes The Student-t distribution [McNeil, 2006] we use in this to robustness to the input outliers while the independent paper is defined as follows. Definition 1. An n-dim random vector x Rn follows the Student-t noise assumption can cope with the target outliers. 2 One challenge of our TPRT method is that the inference is n-variate Student-t distribution with degrees of freedom ν n 2 R+, mean vector µ R , and correlation matrix R Π(n) analytically intractable. To solve the inference problem, we 2 2 utilize Laplace approximation for computing the posterior if its joint probability density function (PDF) is given by and marginal likelihood. The computational cost of TPRT Γ[(ν + n)=2] is roughly the same as that of GPRT, which also requires St (x ν; µ; R) = approximate inference. From the perspective of posterior and j Γ(ν=2)νn=2πn=2 R 1=2 j j marginal likelihood, we show that TPRT is more robust than − ν+n 1 2 GPR and GPRT. Besides, both GPR and GPRT are proved to 1 + (x µ)>R−1(x µ) : be special cases of TPRT. Finally, extensive experiments also · ν − − demonstrate the effectiveness of our TPRT method on both synthetic and real datasets. Given the definition of Student-t distribution, we can have the definition of Student-t Process [Shah et al., 2014]. 2 Background Definition 2. The process f is a Student-t Process (TP) on with degrees of freedom ν R+, mean function m: In this section, we will briefly introduce Gaussian Process RX, and kernel function k: 2 R if any finite subsetX! Regression (GPR), provide the definitions of Student-t dis- of function values have a multivariateX × X ! Student-t distribution, tribution and Student-t Process, and then compare Gaussian n i.e., f = f (xi) i=1 St(ν; µ; K) where K Π(n) with Process (GP) with Student-t Process (TP). f g ∼ n 2 Kij = k(xi; xj; θk) and µ R with µi = m(xi). We 2 2.1 Review of GPR denote that the process f is a Student-t Process with degrees of freedom ν, mean function m, and kernel function k as f In a regression problem, we have a training set = X; y ∼ n D f g TP (ν; m; k). of n instances, where X = xi and xi denotes a d-dim n f gi=1 input vector; y = yi i=1 and yi denotes a scalar output or target. In GPR, wef haveg 2.3 Comparison of GP and TP yi = f (xi) + i; i = 1; 2; :::; n; (1) In [Shah et al., 2014], it has been proved that GP is a special case of TP with degrees of freedom ν + . Among all the where i ; ; :::; n is assumed to be i.i.d. Gaussian i ( = 1 2 ) elliptical processes with an analytically! representable1 density, noise and the latent function f is given a GP prior, implying TP is the most general one, which implies its expressiveness that any finite subset of latent variables f f x n fol- = ( i) i=1 for nonparametric Bayesian modeling. The comparison of TP low a multivariate Gaussian distribution, i.e.,fp f Xg; K ( ) = and GP is illustrated in Figure 1 ([Shah et al., 2014]), from f µ; K , where µ is the mean vector andjK is the ( ) which we can see that TP allows the samples (blue solid) to covarianceN j matrix. Specifically, µ is an n-dim vector which be away from the mean (red dashed) while the samples of is usually assumed to be 0 for simplicity, and K is the GP gather around the mean. This indicates that the outliers covariance matrix with K k x ; x θ , in which k is i;j = ( i j; k) (usually away from the mean) will not have much effect on a kernel function and θ θ ; θ ; : : : ; θ is the set of k = ( k1 k2 kl) the mean of TP, but will affect the mean of GP severely as GP kernel parameters.
Details
-
File Typepdf
-
Upload Time-
-
Content LanguagesEnglish
-
Upload UserAnonymous/Not logged-in
-
File Pages7 Page
-
File Size-