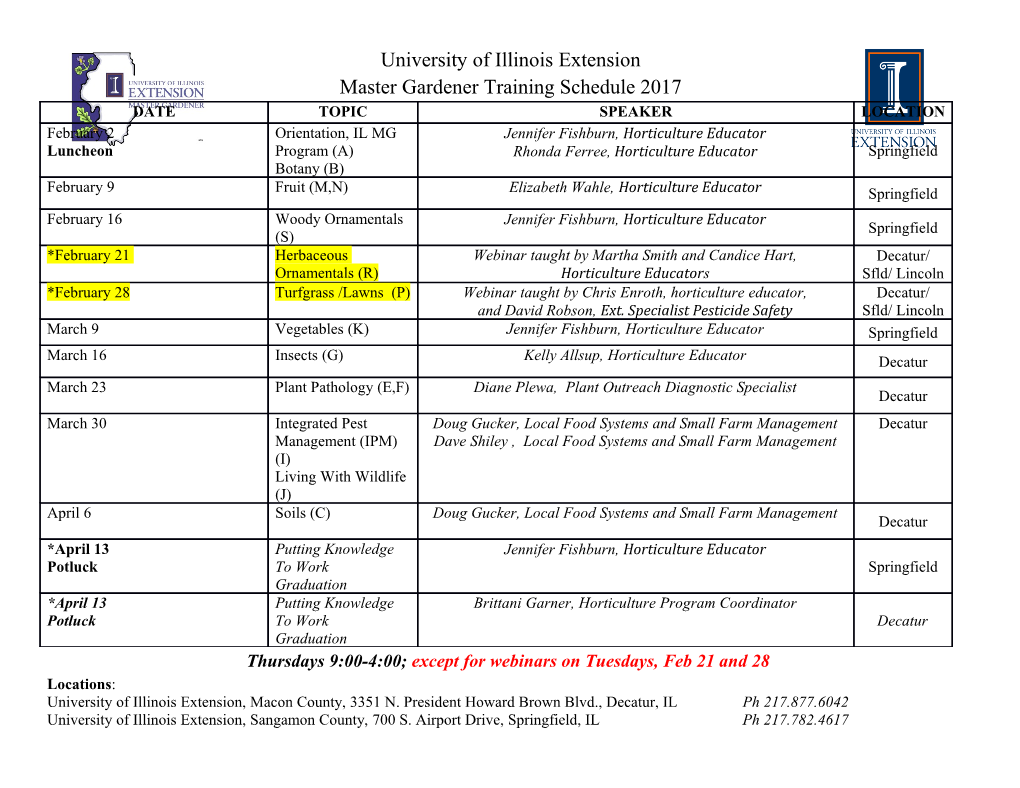
Herfort et al. A typology and analysis of geographic information crowdsourcing Being specific about geographic information crowdsourcing: a typology and analysis of the Missing Maps project in South Kivu Benjamin Herfort Melanie Eckle GIScience Chair, Heidelberg University, GIScience Chair, Heidelberg University, Germany Germany [email protected] [email protected] João Porto de Albuquerque Centre for Interdisciplinary Methodologies, University of Warwick, UK | Dept. of Computer Systems/ICMC, University of Sao Paulo, Brazil | GIScience Chair, Heidelberg University, Germany [email protected] ABSTRACT Recent development in disaster management and humanitarian aid is shaped by the rise of new information sources such as social media or volunteered geographic information. As these show great potential, making sense out of the new geographical datasets is a field of important scientific research. Therefore, this paper attempts to develop a typology of geographical information crowdsourcing. Furthermore, we use this typology to frame existing crowdsourcing projects and to further point out the potential of different kinds of crowdsourcing for disaster management and humanitarian aid. In order to exemplify its practical usage and value, we apply the typology to analyze the crowdsourcing methods utilized by the members of the Missing Maps project developed in South Kivu. Keywords Crowdsourcing, Classification, Digitization, Conflation, OpenStreetMap INTRODUCTION Crowdsourced geographic information has become a new information source for disaster risk management and humanitarian aid in recent years. The efforts during the earthquake that hit Haiti in 2010 have shown that information from Social Media, via SMS and from OpenStreetMap can complement official information. In some cases, when official information is missing at all, these new sources become even more relevant (Horita, Degrossi, Assis, Zipf, & de Albuquerque, 2013). Since the Haitian earthquake new organizations and groups such as the Humanitarian OpenStreetMap Team (HOT), the Standby Task Force, the GEOCAN consortium or the Digital Humanitarian Network break new grounds on how to organize and incorporate the work force of volunteers for disaster management and humanitarian aid. Their work shows, that crowdsourcing geographical information also demands tools that allow the collaborative and coordinated work of thousands of people. Nevertheless, recent research often focuses on crowdsourcing as a data source and equates it with terms like Short Paper – Geospatial Data and Geographical Information Science Proceedings of the ISCRAM 2016 Conference – Rio de Janeiro, Brazil, May 2016 Tapia, Antunes, Bañuls, Moore and Porto de Albuquerque, eds. Herfort et al. A typology and analysis of geographic information crowdsourcing volunteered geographic information or user generated geographical content. As a result, crowdsourcing activities are commonly distinguished with respect to their different platforms or data sources such as Twitter, Flickr, OpenStreetMap or Wikimapia. In contrast, this paper utilizes a definition of crowdsourcing as a method and not as a data source. We therefore understand that crowdsourcing is, as defined by Quinn & Bederson (2011), the act of taking a job traditionally performed by a designated agent (usually an employee) and outsource it to an undefined, generally large group of people in the form of an open call. There is some recent work that attempts to classify the different roles that can be played by the crowd in disaster management (Liu, 2014) and how they fit in the different phases of the disaster management cycle (Horita et al., 2013). In scientific research there is a strong link between the fields of crowdsourcing geographic information and citizen science. Haklay (2013) proposes four different levels of participation and engagement in citizen science projects: crowdsourcing, distributed intelligence, participatory sensing and extreme citizen science. However, what is currently missing is an overview about the specific tasks related to the creation of geographic information by the crowd, i.e. what are the tasks related to the production or enrichment of geographic information that can be ‘outsourced’ to the crowd. To fill this gap, this paper attempts to develop a typology of geographical information crowdsourcing. Furthermore, we use this typology to frame existing crowdsourcing projects and to further point out the potential of different kinds of crowdsourcing for disaster management and humanitarian aid. Finally, in order to exemplify its practical usage and value, we apply the typology to analyze the crowdsourcing methods utilized by the members of the Missing Maps project developed in South Kivu. TYPOLOGY In this section, we present a typology of geographical information crowdsourcing. In the following we distinguish three levels of crowdsourcing: classification, digitization and conflation. For each of these levels we will provide information on purpose, subtasks and complexity. Furthermore, we review and describe existing crowdsourcing projects to exemplify each category. An overview of these issues can be found in table 1. Levels Description Complex Examples in the extant literature ity 1: classification The process of assigning predefined low Chan, Munro, & Schnoebelen (2013), attributes (values /categories) to Vieweg, Castillo, & Imran (2014), Imran, existing geographical information Castillo, Lucas, Meier, & Rogstadius (2014), Ostermann (2015) 2: digitization The process of creating new medium Barrington et al. (2011), Hillen & Höfle, geographic objects based on (2015), Arcanjo, Luz, Fazenda, & Ramos existing geographic information. (2016) 3: conflation The process of integrating existing high Soden, Budhathoki, & Palen (2014) geographic information representing the same real-world object into a consistent scheme Table 1. Typology of geographical information crowdsourcing The typology proposes ‘classification’ as the first level of crowdsourcing geographical information, i.e. the assignment of an additional attribute to an existing piece of geographic information. This attribute (also refered to as ‘tag’ or ‘label’) usually indicates a category and/or property of the geographic object to which it is attached. This task has a low level of complexity and thus bears the potential to be executed by a large number of volunteers, since it does not demand too much in terms of experience or skills. Classification tasks are usually based on the content of a single information source. Furthermore, this first level of crowdsourcing geographical information has the potential to be combined and augment automated classification methods, such as those based on machine learning techniques. Several past projects used crowdsourcing to classify geographical information. In the aftermath of hurricane Sandy hitting the US east coast in 2012, for instance, CAP and FEMA used an online crowdsourcing system for damage assessment. Non-expert volunteers were asked to evaluate the level of damage present in aerial images Short Paper – Geospatial Data and Geographical Information Science Proceedings of the ISCRAM 2016 Conference – Rio de Janeiro, Brazil, May 2016 Tapia, Antunes, Bañuls, Moore and Porto de Albuquerque, eds. Herfort et al. A typology and analysis of geographic information crowdsourcing captured after the hurricane hit the coastline (Chan et al., 2013). Through the MicroMappers crowdsourcing platform, volunteers can support disaster management by reading and labeling tweets based on the information they contain (Vieweg et al., 2014). Imran, Castillo, Lucas, Meier, & Rogstadius (2014) use such crowdsourced classification of social media messages to train supervised classifiers that are applied to a message stream. A similar approach that combines automated and human classification is pursued by Ostermann (2015). The author uses crowdsourcing for the supervision of machine learning classification and regression tasks in order to process geo-social media streams. Digitization is the second level of crowdsourcing geographical information in our typology. Digitization describes the process of creating new geographic objects, based on existing geographical information. Such spatial objects, representing real-world objects or features, have a topology (which can be points, lines or areas) and must be located in space (e.g. using a reference coordinate system). This level requires some experience and knowledge in using mapping tools or GIS software. Crowdsourced digitization of geographical information is also usually based on the contents of a single information source. Although the automated extraction of geographic objects and features from geographical information is a field of vast research, the results of automated approaches often require detailed knowledge about the characteristics of the features and the given input datasets. Several past projects relied upon crowdsourcing techniques to digitize geographical information. After the 2010 Haiti catastrophe, crowdsourced digitization of buildings was used for the rapid analysis of damage caused by the earthquake based on satellite imagery (Barrington et al., 2011). Hillen & Höfle (2015) present a crowdsourcing approach to digitize buildings from earth observation data incorporating the reCAPTCHA concept. The ForestWatcher citizen science project asked users to draw polygons for regions that show deforestation (Arcanjo et al., 2016). The OpenStreetMap community and the Humanitarian
Details
-
File Typepdf
-
Upload Time-
-
Content LanguagesEnglish
-
Upload UserAnonymous/Not logged-in
-
File Pages8 Page
-
File Size-