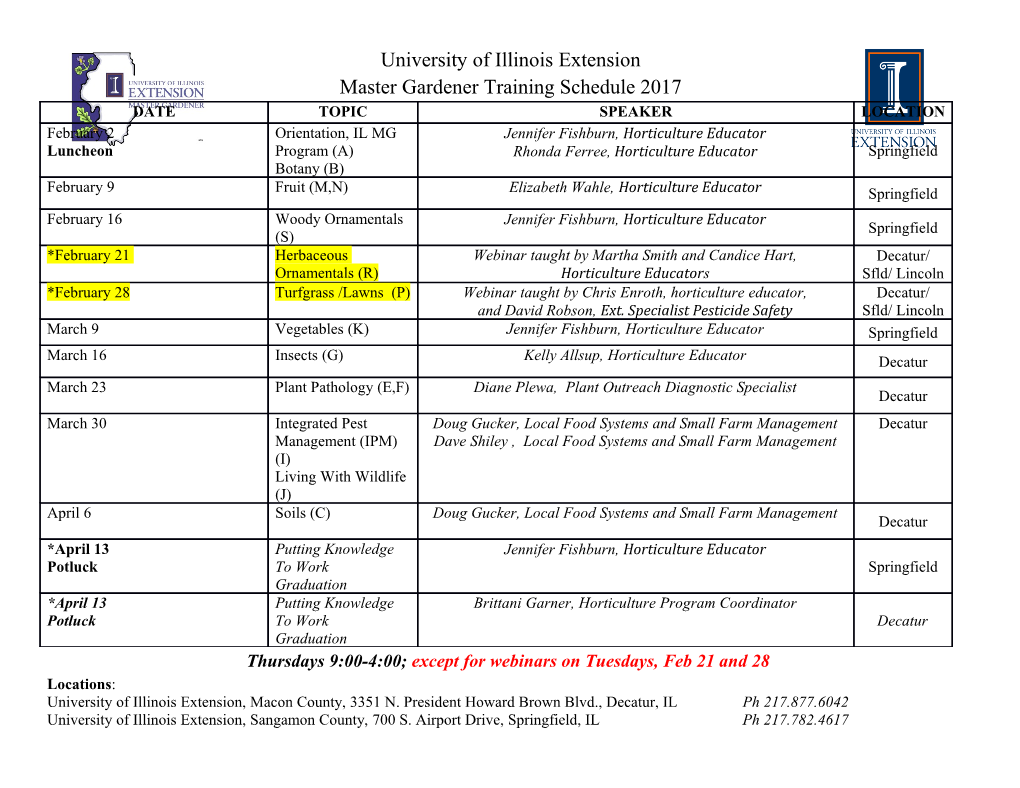
Algebraic Methods for Evaluating Integrals in Bayesian Statistics by Shaowei Lin A dissertation submitted in partial satisfaction of the requirements for the degree of Doctor of Philosophy in Mathematics in the Graduate Division of the University of California, Berkeley Committee in charge: Professor Bernd Sturmfels, Chair Professor Lior Pachter Associate Professor Yun S. Song Spring 2011 Algebraic Methods for Evaluating Integrals in Bayesian Statistics Copyright 2011 by Shaowei Lin 1 Abstract Algebraic Methods for Evaluating Integrals in Bayesian Statistics by Shaowei Lin Doctor of Philosophy in Mathematics University of California, Berkeley Professor Bernd Sturmfels, Chair The accurate evaluation of marginal likelihood integrals is a difficult fundamental problem in Bayesian inference that has important applications in machine learning and computational biology. Following the recent success of algebraic statistics [16,41,43] in frequentist inference and inspired by Watanabe’s foundational approach to singular learning theory [58], the goal of this dissertation is to study algebraic, geometric and combinatorial methods for computing Bayesian integrals effectively, and to explore the rich mathematical theories that arise in this connection between statistics and algebraic geometry. For these integrals, we investigate their exact evaluation for small samples and their asymptotics for large samples. According to Watanabe, the key to understanding singular models lies in desingularizing the Kullback-Leibler function K(ω) of the model at the true distribution. This step puts the model in a standard form so that various central limit theorems can be applied. While general algorithms exist for desingularizing any analytic function, applying them to non-polynomial functions such as K(ω) can be computationally expensive. Many singular models are however represented as regular models whose parameters are polynomial functions of new parameters. Discrete models and multivariate Gaussian models are all examples. We call them regularly parametrized models. One of our main contributions is showing how this polynomiality can be exploited by defining fiber ideals for singular models and relating the properties of these algebraic objects to the statistics. In particular, we prove that a model is put in standard form if we monomialize the corresponding fiber ideal. As a corollary, the learning coefficient of a model is equal to the real log canonical threshold (RLCT) of the fiber ideal. While complex log canonical thresholds are well-studied in algebraic geometry, little is known about their real analogs. In Chapter 4, we prove their fundamental properties and simple rules of computation. We also extend Varchenko’s notion [54] of Newton polyhedra and nondegeneracy for functions to ideals. Using these methods, we discover a formula for the RLCT of a monomial ideal with respect to a monomial amplitude. For all other ideals, this formula is an upper bound for their RLCT. Our tools are then applied to a difficult statistical example involving a na¨ıve Bayesian network with two ternary random variables. 2 Because our statistical models are defined over compact semianalytic parameter spaces Ω, we need to extend standard asymptotic theory [3] of real analytic functions over neigh- borhoods of the origin to functions over domains like Ω. Chapter 3 summarizes these results which are critical for other proofs in this dissertation. We also give explicit formulas for the full asymptotic expansion of a Laplace integral over Ω in terms of the Laurent coefficients of the associated zeta function. In Chapter 5, we apply these formulas to Laplace integrals Z(n) with nondegenerate phase functions, and describe algorithms for computing the coeffi- cient C in the first term asymptotics Z(n) ≈ Cn−λ(log n)θ−1. Procedures for calculating all higher order coefficients are also developed and explained. Watanabe’s treatment of singular models assumes knowledge of the true distribution. In this dissertation, we also explore marginal likelihood integrals of exponential families given data where the true distribution is unknown. This is the context in which Schwarz [48], Haughton [27] and Geiger and Rusakov [45] studied the Bayesian Information Criterion (BIC). We find here that the log likelihood ratio of the data is equal to the Kullback-Leibler function of the model at the maximum likelihood distribution. Therefore, all the methods we developed for Kullback-Leibler functions apply, so we describe how to compute the full asymptotics of the marginal likelihood integral by monomializing the associated fiber ideal. Lastly, to complement developments in asymptotic estimation as well as in Markov Chain Monte Carlo (MCMC) estimation, we present, in Chapter 2, symbolic algorithms for comput- ing marginal likelihood integrals exactly for discrete data of small samples. The underlying statistical models are mixtures of independent distributions, or, in geometric language, secant varieties of Segre-Veronese varieties. For these models, the numerical value of the integral is a rational number, and exact evaluation means computing that rational number rather than a floating point approximation. These exact results provide a gold standard with which approximation methods can be compared. i To my Brother ii Contents Acknowledgments iv 1 Integrals in Bayesian Statistics 1 1.1 Model Selection . 2 1.1.1 Maximum Likelihood . 3 1.1.2 Marginal Likelihood . 3 1.1.3 Cross Validation . 4 1.2 Regular and Singular Models . 5 1.3 Model Asymptotics . 8 1.3.1 Bayesian Information Criterion . 8 1.3.2 Singular Learning Theory . 9 1.4 Important Classes of Models . 13 1.4.1 Discrete Models . 13 1.4.2 Multivariate Gaussian Models . 14 1.4.3 Exponential Families . 14 1.4.4 Graphical Models . 16 1.4.5 Mixtures of Independence Models . 17 1.5 Regularly Parametrized Models . 22 1.5.1 Fiber Ideals . 23 1.5.2 Real Log Canonical Thresholds . 25 1.5.3 Desingularizing the Kullback-Leibler Function . 26 1.6 Marginal Likelihood of Exponential Families . 30 2 Exact Evaluation 38 2.1 Independence Models and their Mixtures . 39 2.2 Summation over a Zonotope . 41 2.3 Algorithms . 46 2.3.1 Ignorance is Costly . 46 2.3.2 Symbolic Expansion of the Integrand . 47 2.3.3 Storage and Evaluation of φA(b, U)................... 48 2.3.4 Limitations and Applications . 49 iii 2.4 Back to Bayesian Statistics . 52 3 Asymptotic Theory 57 3.1 Resolution of Singularities . 58 3.2 Zeta Functions . 61 3.2.1 Monomialization . 61 3.2.2 Localization . 63 3.2.3 Comparability of Phase Functions . 64 3.2.4 Boundary of Domain of Integration . 65 3.2.5 Deepest Singularities . 66 3.3 Laplace and Mellin Transforms . 66 3.4 Asymptotic Expansion . 67 4 Real Log Canonical Thresholds 69 4.1 Fundamental Formulas . 70 4.1.1 Equivalent definitions . 70 4.1.2 Choice of Generators . 71 4.1.3 Sum, Product and Chain Rules . 72 4.2 Newton Polyhedra . 74 4.2.1 Nondegeneracy . 74 4.2.2 Toric Resolutions . 78 4.2.3 Monomial Ideals . 79 4.3 Applications to Statistical Models . 81 5 Higher Order Asymptotics 91 5.1 Sum, Product and Chain Rules . 92 5.2 Leading Coefficient . 95 5.3 Higher Order Coefficients . 98 Bibliography 105 iv Acknowledgments First and foremost, I want to thank my advisor, Bernd Sturmfels, for inspiring me to pursue this research topic. Through the years, he taught by example what it means to be a successful researcher. I learned to always ask good questions and to always ask for help. I also want to thank his family for being like a family to me in Berlin and in Berkeley. Second, I am grateful to two great statisticians, Sumio Watanabe and Mathias Drton, who were very patient in imparting their insights on this subject area. Watanabe taught me to approximate Kullback-Leibler functions with sums of squares, while Drton inspired me to extend my results for discrete models to regularly parametrized models. Thank you for being so generous with your ideas, advice and encouragement. Third, I am thankful for Zhiqiang Xu, who was a co-author on one of my paper. I enjoyed the many hours we spent coding and discussing problems in Berlin and in Beijing. I want to thank my committee members Lior Pachter and Yun S. Song for organizing memorable classes, attending my qualifying exam, and taking time to read this thesis. Special thanks go to Christine Berkesch, Nero Budur, Anne Fr¨uhbis-Kr¨uger,Luis Garc´ıa- Puente, Anton Leykin, Robin Pemantle, Josef Schicho, Zach Teitler and Piotr Zwiernik for teaching me so much. I am also grateful to Morgan Brown, Dustin Cartwright, Mar´ıaCueto, Jes´usDe Loera, Daniel Erman, Alex Fink, Christopher Hillar, Edward Kim, Matthias K¨oppe, Jiawang Nie, Luke Oeding, Philipp Rostalski, Raman Sanyal, Caroline Uhler and Cynthia Vinzant for all the enlightening discussions. Of course, my PhD would not be possible without funding and guidance from the Agency for Science, Technology and Research (A*STAR), Singapore. I am also indebted to the UC Berkeley Mathematics Department and to MSRI for the many opportunities to grow. In my personal life, few people have given me as much encouragement and support as Diogo Oliveira e Silva, Dewen Soh, Jeremy Chan, my sister Qianwen and my loving parents. Thank you for believing in me! To my wonderful fianc´eeand best friend Cynthia Yu: nobody knows what goes on behind the scenes besides you. Thank you for holding my hands in this journey. To Father God, Jesus and Holy Spirit: thank you for never letting go. You have opened my eyes to see. Thank you for setting me free to play, to dream and to fly. 1 Chapter 1 Integrals in Bayesian Statistics Bayesian statistics is foundational in applications such as machine learning and compu- tational biology. A fundamental problem in Bayesian statistics is the accurate evaluation of integrals. This chapter is an introduction to some of the theory that has developed around this problem, and a summary of the major contributions in this dissertation.
Details
-
File Typepdf
-
Upload Time-
-
Content LanguagesEnglish
-
Upload UserAnonymous/Not logged-in
-
File Pages117 Page
-
File Size-