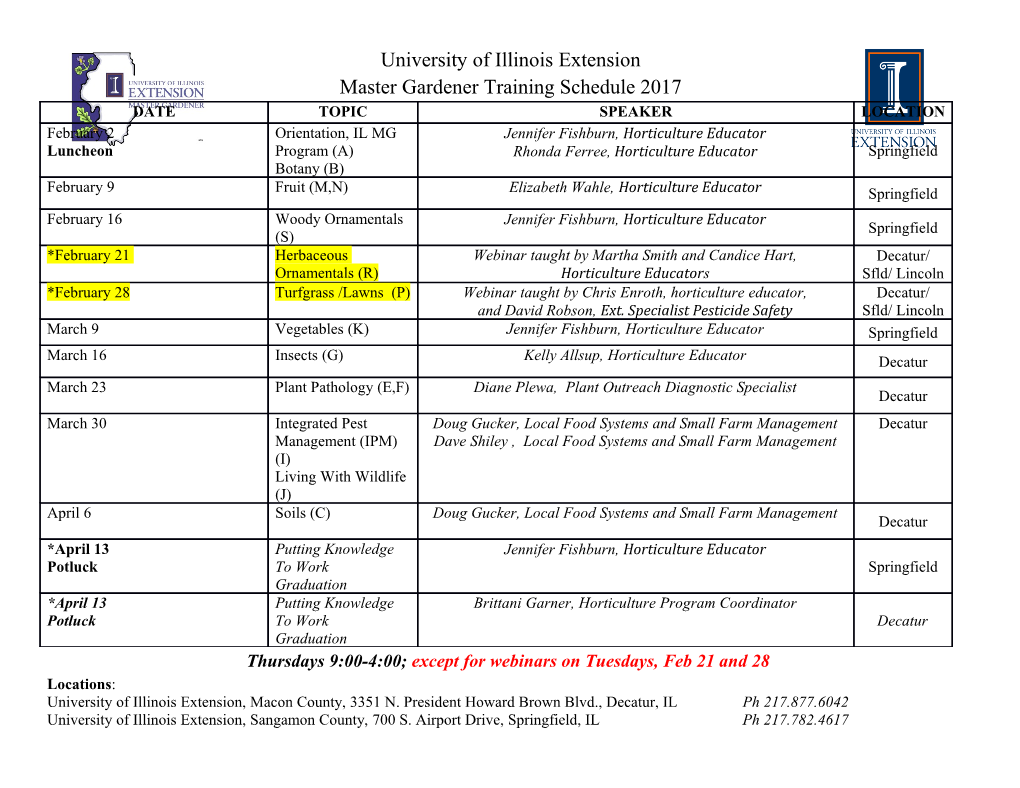
© 2020 Qi Wang SECURING EMERGING IOT SYSTEMS THROUGH SYSTEMATIC ANALYSIS AND DESIGN BY QI WANG DISSERTATION Submitted in partial fulfillment of the requirements for the degree of Doctor of Philosophy in Computer Science in the Graduate College of the University of Illinois at Urbana-Champaign, 2020 Urbana, Illinois Doctoral Committee: Professor Carl A. Gunter, Chair Professor Klara Nahrstedt Assistant Professor Adam Bates Assistant Professor Kangkook Jee, University of Texas at Dallas ABSTRACT The Internet of Things (IoT) is growing very rapidly. A variety of IoT systems have been developed and employed in many domains such as smart home, smart city and industrial control, providing great benefits to our everyday lives. However, as IoT becomes increasingly prevalent and complicated, it is also introducing new attack surfaces and security challenges. We are seeing numerous IoT attacks exploiting the vulnerabilities in IoT systems everyday. Security vulnerabilities may manifest at different layers of the IoT stack. There is no single security solution that can work for the whole ecosystem. In this dissertation, we explore the limitations of emerging IoT systems at different layers and develop techniques and systems to make them more secure. More specifically, we focus on three of the most important layers: the user rule layer, the application layer and the device layer. First, on the user rule layer, we characterize the potential vulnerabilities introduced by the interaction of user-defined automation rules. We introduce iRuler, a static analysis system that uses model checking to detect inter-rule vulnerabilities that exist within trigger-action platforms such as IFTTT in an IoT deployment. Second, on the application layer, we design and build ProvThings, a system that instruments IoT apps to generate data provenance that provides a holistic explanation of system activities, including malicious behaviors. Lastly, on the device layer, we develop ProvDetector and SplitBrain to detect malicious processes using kernel-level provenance tracking and analysis. ProvDetector is a centralized approach which collects all the audit data from the clients and performs detection on the server. SplitBrain extends ProvDetector with collaborative learning, where the clients collaboratively builds the detection model and performs detection on the client device. ii To my parents, for their love and support. & To my mother, I will love you forever. iii ACKNOWLEDGMENTS I would like to thank my advisor Professor Carl Gunter for his great supervision and guidance. Professor Gunter has provided me with enormous support and insightful advice during my Ph.D. study. He gave me a lot of freedom to explore the research problems that I find interesting. I am always feeling extremely fortunate and thankful to work with and learn from him. His kindness and wisdom have motivated and inspired me a lot through my Ph.D. study, and will continue to motivate me in the future. I would also like to extend my appreciation to the rest of my dissertation committee members, Professor Klara Nahrstedt, Assistant Professor Adam Bates and Assistant Pro- fessor Kangkook Jee, for their service and support. They have provided insightful feedback, suggestions and guidance to this dissertation. I would like to thank Adam Bates, one major collaborator for my IoT security research. It was fantastic to have the opportunity to work with him. I am thankful for his aspir- ing professional guidance, invaluable constructive criticism and positive attitude during my research work. With special mention to Kangkook Jee, my two-times NEC Laboratories America, Inc. (NEC Labs) internship mentor. I'm grateful that I had the opportunity of working with and learning from him. He helped me not only in research but also in life. I am fortunate to have such a friend. I would like to thank all my coauthors and collaborators during my Ph.D. study: pro- fessors Nikita Borisov, William H Sanders, Jos´eMeseguer, Indranil Gupta and Bo Li at University of Illinois at Urbana-Champaign (UIUC); researchers Haifeng Chen, Ding Li, Xiao Yu, Zhengzhang Chen, Wei Cheng and Junghwan Rhee from NEC labs; and my fellow students Xiaojun Xu, Huichen Li, Wajih Ul Hassan, Pubali Datta, Benjamin E Ujcich, Si Liu, Wei Yang and Karan Ganju. I apologize for any of the inevitable omissions. I am also very thankful to my lab mates and all my friends at UIUC and during internships for making my Ph.D. life enjoyable. Special thanks to Yi Zhang, Si Liu, Sihan Li, and Wei Yang. I am extremely grateful to my parents and sisters for supporting me through all these years. Their unconditional love and support helped me bring this adventure to its end. This dissertation is dedicated to them. iv TABLE OF CONTENTS CHAPTER 1 INTRODUCTION . 1 1.1 Thesis Statement . .2 1.2 Dissertation Contributions . .3 1.3 Dissertation Organization . .4 CHAPTER 2 PRELIMINARY CONCEPTS . 5 2.1 IoT Platforms and Smart Home Platforms . .5 2.2 Trigger-Action IoT Platforms . .7 2.3 The Growth and Risks of Highly Functional IoT Devices . .9 2.4 Data Provenance . .9 CHAPTER 3 UNDERSTANDING AND DISCOVERING INTER-RULE VUL- NERABILITIES . 11 3.1 Introduction . 11 3.2 Background . 13 3.3 Threat Model and Assumptions . 13 3.4 Characterization of Inter-Rule Vulnerabilities . 14 3.5 Approach: IRULER . 18 3.6 Evaluation . 23 3.7 Discussion and Limitations . 28 3.8 Related Work . 28 3.9 Conclusion . 30 CHAPTER 4 PROVIDING PROVENANCE TRACING TO IOT PLATFORMS . 31 4.1 Introduction . 31 4.2 Background . 33 4.3 Threat Model and Assumptions . 35 4.4 Approach: ProvThings . 36 4.5 Implementation . 43 4.6 Evaluation . 46 4.7 User Scenarios . 50 4.8 Discussion and Limitations . 57 4.9 Related Work . 58 4.10 Conclusion . 59 CHAPTER 5 DETECTING STEALTHY ATTACKS AGAINST DEVICES VIA DATA PROVENANCE ANALYSIS . 61 5.1 Introduction . 61 5.2 Background . 64 5.3 Threat Model and Assumptions . 69 v 5.4 Problem Definition . 69 5.5 Approach: ProvDetector ........................... 71 5.6 Evaluation . 77 5.7 Discussion and Limitations . 89 5.8 Related Work . 90 5.9 Conclusion . 93 CHAPTER 6 ENABLING ON-DEVICE ANOMALY DETECTION WITH FED- ERATED LEARNING . 94 6.1 Introduction . 94 6.2 Background . 97 6.3 Threat Model and Assumptions . 100 6.4 Approach: SplitBrain . 100 6.5 Evaluation . 107 6.6 Discussion . 111 6.7 Related Work . 112 6.8 Conclusion . 113 CHAPTER 7 FUTURE WORK AND CONCLUSION . 114 7.1 Future Work . 114 7.2 Concluding Remarks . 115 REFERENCES . 117 APPENDIX A EXAMPLE CODE IN IOT PLATFORMS . 138 A.1 IFTTT Applet Filter Code Example . 138 A.2 The Code Structure of an Example Device Handler . 138 APPENDIX B EXAMPLE DEVICE/SERVICE METADATA OF IRULER . 139 APPENDIX C SOURCE CODE OF SMARTAPPS USED IN PROVTHINGS CASE STUDIES . 141 C.1 Source Code of the LockItWhenILeave SmartApp . 141 C.2 Source Code of the FaceDoor SmartApp . 142 vi CHAPTER 1: INTRODUCTION The Internet of Things (IoT) is growing rapidly. The number of IoT devices deployed worldwide is expected to reach 20.4 billion by 2020, forming a global market of 13 trillion dollars [1]. The rapid expansion of IoT is providing great benefits to our everyday lives. For example, smart homes now offer the ability to automatically manage household appliances, while smart health initiatives have made monitoring more effective and adaptive for each patient. With the increasing of user requirements, IoT devices are becoming more complex. Voice assistants, smart-home hubs, wearables, drones, and automobiles are just some examples. Recent development of inexpensive and highly functional hardware [2, 3] has introduced cost- effective ways to implement IoT devices running community-verified IoT operating systems (e.g., Android Things [4] and Ubuntu IoT [5]). Leveraging existing full-fledged IoT operating systems (OSes), it saves a lot of time and efforts to build highly functional IoT devices to meet the growing and diversified computational demands. On the other hand, in response to the increasing availability of smart devices, a variety of IoT platforms have emerged that are able to interoperate with devices from different manufactures. Samsung's SmartThings [6], Apple's HomeKit [7], and Google's Android Things [4] are just a few examples. IoT plat- forms offer appified software [8] for the management of smart devices, with many going so far as to provide programming frameworks for the design of third-party applications. To sup- port easier end-user customizations, many IoT platforms provide user-friendly programming frameworks for the design of simple automation logic that enable customized functionality. For example, IFTTT [9] and Zapier [10]. Currently, trigger-action programming (TAP) is the most commonly-used model to create automations in IoT. Studies have shown that about 80% of the automation requirements of typical users can be represented by TAP and that even non-programmers can easily learn this paradigm [11]. However, as long prophesied by our community, the expansion of IoT is also now bringing about new challenges in terms of security and privacy. Recently, there are numerous IoT attacks exploit the vulnerabilities in IoT devices [12, 13, 14, 15, 16, 17], protocols [18, 19], apps and platforms [20]. In some cases, IoT attacks could have chilling safety consequences { burglars can now attack a smart door lock to break into homes [14], and arsonists may even attack a smart oven to cause a fire [21]. There are considerable challenges to protect IoT. First, new IoT devices are released and deployed every day. Exploits targeting IoT devices are also being developed by ad- versaries at a similarly high pace, making the threats against IoT devices highly dynamic 1 and ever-increasing. Second, Most IoT devices have limited resource allocations. It is thus a challenging task to build an effective host-based data collection and detection solution that runs on minimal resources.
Details
-
File Typepdf
-
Upload Time-
-
Content LanguagesEnglish
-
Upload UserAnonymous/Not logged-in
-
File Pages149 Page
-
File Size-