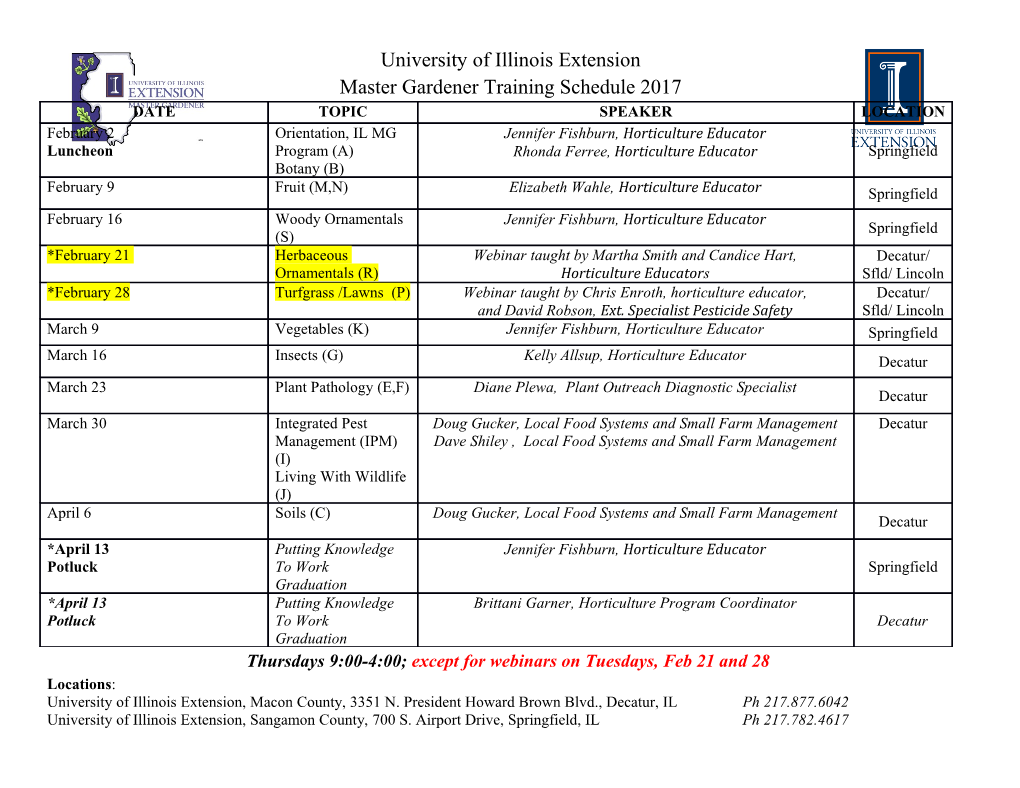
Genes & Immunity https://doi.org/10.1038/s41435-018-0051-y ARTICLE Unfolding of hidden white blood cell count phenotypes for gene discovery using latent class mixed modeling 1 1 2 3 3 4 Taryn O. Hall ● Ian B. Stanaway ● David S. Carrell ● Robert J. Carroll ● Joshua C. Denny ● Hakon Hakonarson ● 2 4 5 6 7 Eric B. Larson ● Frank D. Mentch ● Peggy L. Peissig ● Sarah A. Pendergrass ● Elisabeth A. Rosenthal ● 7 1 Gail P. Jarvik ● David R. Crosslin Received: 17 September 2018 / Revised: 24 September 2018 / Accepted: 24 October 2018 © Springer Nature Limited 2018 Abstract Resting-state white blood cell (WBC) count is a marker of inflammation and immune system health. There is evidence that WBC count is not fixed over time and there is heterogeneity in WBC trajectory that is associated with morbidity and mortality. Latent class mixed modeling (LCMM) is a method that can identify unobserved heterogeneity in longitudinal data and attempts to classify individuals into groups based on a linear model of repeated measurements. We applied LCMM to repeated WBC count measures derived from electronic medical records of participants of the National Human Genetics 1234567890();,: 1234567890();,: Research Institute (NHRGI) electronic MEdical Record and GEnomics (eMERGE) network study, revealing two WBC count trajectory phenotypes. Advancing these phenotypes to GWAS, we found genetic associations between trajectory class membership and regions on chromosome 1p34.3 and chromosome 11q13.4. The chromosome 1 region contains CSF3R, which encodes the granulocyte colony-stimulating factor receptor. This protein is a major factor in neutrophil stimulation and proliferation. The association on chromosome 11 contain genes RNF169 and XRRA1; both involved in the regulation of double-strand break DNA repair. Introduction Electronic supplementary material The online version of this article White blood cell count (WBC) count is a marker of sys- (https://doi.org/10.1038/s41435-018-0051-y) contains supplementary temic inflammation and immune system health. WBC count material, which is available to authorized users. varies acutely in response to infection and other environ- — * Taryn O. Hall mental exposures. However, resting-state WBC count the [email protected] WBC level when the immune system is neither challenged * David R. Crosslin nor suppressed—may be an indicator of chronic disease [email protected] risk. Elevated resting WBC count has been associated with metabolic syndrome [1–4], cardiovascular disease [5, 6] and 1 Department of Biomedical Informatics Medical Education, School mortality [7–11]. This may reflect excess inflammation as of Medicine, University of Washington, Seattle, WA 98109, USA evidenced by WBC count, or leukocytes may contribute 2 Kaiser Permanente Washington Health Research Institute directly to disease [12]. (Formerly Group Health Cooperative-Seattle), Kaiser Permanente, While WBC count is impacted by modifiable factors Seattle, WA 98109, USA such as smoking [13–15] and body composition [16–18], 3 Departments of Biomedical Informatics and Medicine, Vanderbilt resting-state WBC count is also influenced by ancestry, and University, Nashville, TN 37235, USA has been found to be partly under genetic control, with 4 Children’s Hospital of Philadelphia, Philadelphia, PA 19104, USA heritability estimated at around 40% [19]. Individuals with 5 Center for Human Genetics, Marshfield Clinic Research Institute, African ancestry, on average, have lower WBC count Marshfield, WI 54449, USA compared to individuals with European ancestry, attributed 6 Geisinger Research, Rockville, MD 20850, USA to lower neutrophil count [20, 21]. Among those with 7 Division of Medical Genetics, School of Medicine, University of African ancestry, total WBC and neutrophil count has been Washington, Seattle, WA 98105, USA associated with SNP rs2814778 in the ACKR1/DARC gene, T. O. Hall et al. Table 1 LCMM fit statistics Model Link Classes BIC Entropy Average posterior probability Sample size (%) 1a Linear 1 339342.22 –– 9742 (100) 1b Beta 1 301289.38 ––– 9742 (100) 2 Beta 2 299820.82 0.27 0.73 0.75 3349 (35) 6292 (65) 3 Beta 3 299145.93 0.44 0.75 0.71 0.70 209 (2) 6725 (70) 2702 (28) 4 Beta 4 298777.82 0.52 0.77 0.73 0.65 0.70 151 (1) 7639 (78) 1595 (16) 357 (4) via admixture mapping [22, 23]. This association was behavior, attention-deficit hyperactivity disorder and Aut- replicated in several genome-wide association studies, ism) [37–44]. including our own, and a meta-analysis [24–27]. Here, we applied a trajectory analysis, using latent class There is evidence that resting-state WBC count is not mixed modeling (LCMM) [45], to longitudinal WBC count fixed over time. Longitudinal analysis has shown a U- data obtained from the EMR from the electronic MEdical shaped pattern in WBC counts over the lifespan, dipping Record and GEnomics (eMERGE) Network study. We then around age 60 and then increasing [9]. Similarly, cross- conducted a GWAS and identified genetic variants asso- sectional data has shown higher WBC count in individuals ciated with the trajectory classes derived in the deep phe- older than 65 years old [10]. Heterogeneity in WBC count notyping step. trajectory also exists and some trajectories are associated with morbidity and mortality [8]. Because WBC count is also influenced by adiposity, changes in resting-state WBC Results count may reflect age-related change in body composition. However, in a mouse model, different strains exhibited Resting-state WBC count data was identified for 14,018 different WBC count trajectories, indicating these trajec- participants. LCMM requires a minimum number of repe- tories may be under genetic control [28]. ated measurements to appropriately model trajectory (here a Deep phenotyping aims to increase the granularity of a minimum of three data points for a quadratic model). In our phenotype in hopes that a more precise phenotype will sample, 4762 participants were excluded due to insufficient increase the power of a genome-wide association study data. Excluded participants were younger than included (GWAS) and lead to larger effect size estimates [29]. participants (56.6 vs. 64.1 year, respectively). There was Extending a phenotype over time by harnessing the infor- also a higher proportion of participants of genetically mation contained in longitudinal data instead of simple determined African Ancestry (AA) among those excluded. aggregation is one strategy to deepen phenotype [30, 31]. A higher proportion of participants from the Vanderbilt Different trajectories of WBC count over the lifespan may University site and a lower proportion of participants from be a fruitful deep phenotype to use in GWAS. the Marshfield Clinic site were excluded. Trajectory heterogeneity may be difficult to discern in large, observational datasets using standard statistical LCMM selection methods. Trajectory analysis is a method that can identify unobserved heterogeneity in longitudinal data and attempts We evaluated model fit based on Bayesian Information to classify individuals into groups based on a linear model Criteria (BIC), average posterior probability of class mem- of repeated measurements over time [32]. As such, this bership ≥ 70%, and minor class sample size ≥ 10%. A method is particularly suited to the type of data gathered in summary of the models fit are presented in Table 1. Details the electronic medical records (EMR), which contains for all models tested are available in the Supplementary information about multiple traits, gathered repeatedly over Materials. Based on these criteria, we determined that the time. Trajectory analysis, applied to EMR data, has been two-class solution was the best fitting model tested. used to characterize and identify risk factors for multi- Participants were assigned to a trajectory class based on morbidity [33], depression [34], dementia-related cognitive the class for which they had a higher posterior probability of decline [35], and adverse birth weight outcomes [36]. membership, given their data and the model fit. Fig. 1a Trajectory-based phenotypes have been shown to be heri- shows the mean predicted trajectory based on the LCMM table, used in candidate gene studies, linkage analysis and for each class. Class 1 is modeled by the equation GWAS, and have been associated with genetic risk −0.10137*age_at_event + 0.00043*age_at_event2. The scores for a number of complex traits (e.g., systolic blood equation for the Class 2 trajectory is −4.00651- pressure, BMI, schizophrenia, alcohol use and smoking 0.07115*age_at_event + 0.00082*age_at_event2. Figure Unfolding of hidden white blood cell count phenotypes for gene discovery using latent class mixed. Fig. 1 Predicted mean class-specific trajectory (a) and observed mean class-specific trajectory and 95% confidence interval (b) of WBC count by age from the LCMM Table 2 Descriptive characteristics of each trajectory class 1b shows the mean observed trajectory and 95% confidence interval for classed participant data. Class 2 was the major Class 1 (N = Class 2 (N = p-value 3349) 6292) trajectory identified, representing 65% of the participants, showed a stable resting-state WBC count trajectory and then Median [IQR] increased after age 60. The Class 1 WBC count trajectory Observations 8 [5–12] 7 [4–10] <0.0001 decreased steadily across the lifespan and accounted for Age at event 62.5 [52.3– 64.6 [55.1– <0.0001 35% of sample participants. The trajectories cross at about 71.8] 72.9] age 70 and the 95% confidence intervals overlap from ages Number of years of 11.7 [6.5– 10.9 [5.9– <0.0001 68 to 72. The average posterior probability of Class 1 and follow-up 17.5] 15.9] Class 2 membership was moderately high at 73% and 75%, WBC count 7.4 [6.2–8.6] 6.2 [5.4–7.1] <0.0001 respectively, but the entropy (a measure of confidence, BMI 28.9 [25.1– 27.9 [24.9– <0.0001 bounded by 0 and 1) of classification was low (0.27). 33.3] 31.3] % The median number of observations, age-at-event, years of follow-up, WBC counts, and BMI were similar in Male 41 45 <0.0001 magnitude between classes (Table 2).
Details
-
File Typepdf
-
Upload Time-
-
Content LanguagesEnglish
-
Upload UserAnonymous/Not logged-in
-
File Pages11 Page
-
File Size-