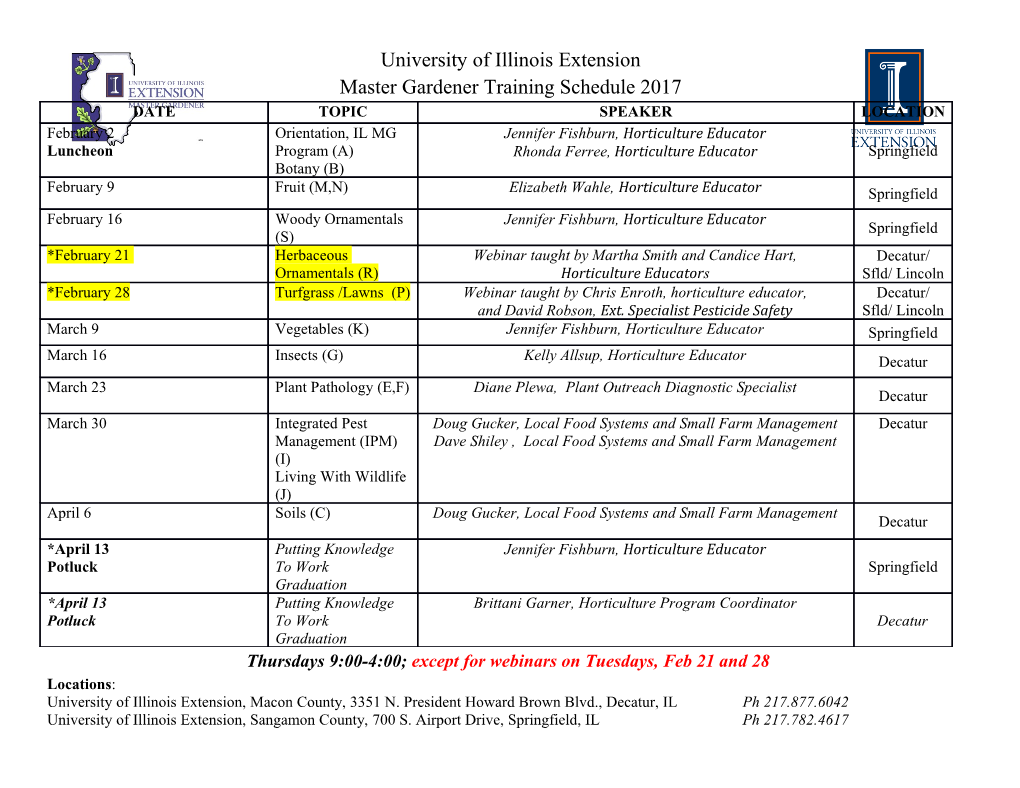
INBREEDING ESTIMATION FROM POPULATION DATA: MODELS, PROCEDURES AND IMPIJCATIONS1 RICHARD S. SPIELMAN,V JAMES V. NEEL,Z and FRANCIS H. F. LIZ 2Depariment of Human Genetics, Uniuersiiy of Michigan Medical School, Ann Arbor, Michigan 48109 SDepariment of Human Genetics, University of Pennsyluania School of Medicine, Philadelphia, Pennsyluania 191744 Manuscript received November 6, 1975 ABSTRACT Four different estimation procedures for niodels of population structure are compared. The parameters of the models are shown to be equivalent and, in most cases, easily expressed in terms of the parameters WRIGHTcalls “F- statistics.” We have estimated the parameters of each of these models with data on nine codominant allele pairs in 47 Yanomama villages, and we find that the different estimators for a given parameter all yield more or less equivalent results. P-statistics are often equated to inbreeding coefficients that are defined as the probability of identity by descent from alleles taken to be unique in some founding population. However, we are led to infer from computer simulation and general historical considerations that all estimates from genotype Ire- quencies greatly underestimate the inbreeding coefficient ior alleles in the founding population of American Indians in the western hemisphere. We sur- mise that in the highly subdivided tribal populations which prevailed until the recent advent of civilization, the probability of identity by descent for homo- logous allelcls was roughly 0.5. We consider some consequences of working with the customary, much lower, estimates-0.005 to 0.01-if, on the time scale of human evolution, these represent only a very recent departure from the inbreeding intensity that prevailed before civilization. RIGHT ( 1943, 195 1,1965) described the genetic properties of a subdivided or “structured” population by a model that, under certain assumptions, leads to estimates of average inbreeding of individuals. Recent years have witnessed the development of a number of alternative models and a proliferation of estimation procedures, described below. In the present communication, we first show how the models and estimators are related, by rewriting them in a uniform notation which reveals the underlying similarities. Then we compare the results obtained by these various approaches with a well-defined body of data. Finally, we attempt to infer how much these procedures err in estimating inbreeding (i.e.,frequency of identity by descent of a pair of alleles) and illirstrate some consequences. 1 Supported by the Energy Research Development Agency E(11-1)-1152 and the National Science Foundation BMS-74-11823. Present address. Genetics 85: 355-371 February, 1977 356 R. S. SPIELMAN, J. V. NEEL AND F. H. F. LI For our test data we use snme of the extensive infomalion now available on the South American Indian. The unusual advantages of such populations for genetic inference were pointed out some time ago (NEELand SALZANO1964; NEEL 1970). Although the conclusions are derived from Indian populations, we believe that with suitable caution they apply to tribal man generally. It will be shown that all estimation procedures based on gene and genotype frequencies yield more or less comparable results, which, however, substantially underestimate the true coefficient of inbreeding in such populations. METHODS We have estimated the structure parameters for each of nine codominant allele pairs (listed in Table 2) using data from 47 Yanomama villages (GERSHOWITZet al. 1972; WEITKAMPand NEEL 1972; WEITKAMPet al. 1972; WARDet al. 1975). The four estimation procedures we have used are desrribed eponymously below, and may be taken to represent four slightly different models. All models assume no selection and envisage a population subdlvided into N smaller units. The estimated allele frequency in the $11 subdivision (size ni) is pz and the mean of pi across subdivisions is 3. Following WRIGHT’Sdefinitions (1943, especially 1965), all models intro- duce one or more parameters anomalously called “F-statistics,” to measure the nonrandomness which results from this structure. In the first three models, F,, is the correlation between two gametes randomly selected from individuals in the same subdivision, relative to gametes of the total population. F,, is the correlation between two gametes uniting to form a zygote, ignoring subdivisicn( i.e., “relative to gametes of the total population”). If generations are discrete and mating is at random within each subdivision (as “random” is specified in the definition of F,,), FIT is exactly equal (ignonng sampling error) to F,, of the previous generation (WRIGHT1965, p. 409-410). If mating is not random, a third correlation, F,,, defined as the correlation between uniting gametes “relative to those of their own subdivision,” reflects departures from random mating within subdivisions. The three F-statistics are related by: These correlations have been estimated as follows: A. W/N: (WORKMANand NISWANDER1970; NEELand WARD1972): Method of moments To estimate the F values, let H, be the frequency of heterozygotes in the total population and SL be the weighted mean squared deviation of pi and i.e., N 6 s2 = .B - a 1=1 Wi(Pi 3)Z. Weighting is proportional to subdivision size; s wi= ni/ I: ni i =I Then INBREEDING POPULATION DATA 357 where & represents “is estimated by.” Rewriting equation (1) we have F, = (FIT - F,,)/ (1 - FST). In the W/N procedure, F,, has been estimated either by substituting estimates for F,, and F,, in this relationship, or as the weighted mean of the within-subdivision analogce of F,y7: AY Fix= %=l.E ~,[1 -Hi/2pi (l-pi)] . (2c) These alternate estimators for F,, are in general not equivalent. B. COCKERHAM(1969,1973): Least squares The model interprets the F values as intraclass correlations, thus implying a nested analysis of variance. The two alleles at a locus are assigned values (say 0, I), and cach individual pro- vides two such allelic measurements for a total score of 0, 1, or 2. The total variance of allelic measurements is the sum of three contributions:G, variance among group means; U;, variance a among individuals (within groups) ; and uz variance between the two alleles (within individuals, W within groups). The equivalence of such variances among units to covariances within units leads naturally to the definition of their ratios as intraclass correlations. Thus COCKERHAMdefines F,, (which he calls T) as and F,,, which he does not usually subscript: These definitions give meaning to WRIGHT’S(1965) phrase “relative to gametes of thc total population” quoted above. For F,, the numerator is the covariance within groups (variance among groups) and for F,,, the covariance within individuals (total variance among individuals). Continuing the analogy, the intraclass correlation interpretation of WRIGHT’Sdefinition, which is algebraically exactly (FIT - F8,)/(1 -- F,,). COCKERAMthus achieves a system of correlations which give statistical meaning to WRIGHT’Sdefinitions and form a coherent set in the idiom of analysis of variance. One attractive consequence is a familiar and natural means of estimation (least squares). For an unblamed design (unequal nL),we may define a conventional weighted analysis. Let ri = N N 2 n,/N, and define no = ri- Z (n,- ri)Z/N(N - 1)ri (SNEDECORand COCHRAN1967; SING, 2.=1 ,=1 CHAMBERLAINand EGGLESTON1973) Table 1 gives the expectation of the mean squares among subdivisions, among individuals within subdivisions. and within individuals. Since observations are restricted to 0 and 1, the sums of squares may be expressed simply in terms of numbers of genotypes or alleles. Setting the expectations equal to observed mean squares, we obtain the usual least squares estimators: U: A (MS, - MSb)/2n0, U: (MSb - MS,,)/2, and U: A MS,. C. RST: (ROTHMAN,SING and TEMPLETON1974): Maximum likelihood The first two characterizations of population structure require no assumptions about prob- ability distributions underlying the observations. In contrast, the RST model makes explicit assumptions about the probability of collections of genotypes in an array of subdivisions. Imagine a population in which genotypes AA, Aa, and an have frequencies P,, P, and P, respectively (PI+ P, + P, = 1). Let the numbers of these three genotypes in the ith subdivision be nil, 358 R. S. SPIELMAN, J. V. NEEL AND F. H. F. LI TABLE 1 Nested analysis of uariance-unbalanced design * ~~ Expectation of Mean squares mean squares v N Among individuals is12niqi(~-qi) - U;+ 20: (within subdivisions) [ 2 rs 1 A’ Within individuals U2 izlni2/2 &ni w (between alleles) 1 1/ * One method of estimation for COCKERHAM’S(least squares) model. From SING,CHAMBERLAIN and EGGLESTON1973. n Estimate frequency qi of A by qi = (2ni1+ ni2)/2ni. For additional notation, see text. ni2,ni3 where nil + ni2 fni, = ni. While the basic parameter of structure in WRIGHT’Smodel is correlation between allele values, the RST model is more convemently expressed in terms of the correlation (p) between the total allelic measurements (0,1, 2) of individuals. The funda- mental equivalence tc WRIGHT’Sform of the model is shown by the relationship D = 2FsT/ (1 f FIT);i.e., p is WRIGHT’S(1922) coefficient of relationship. If a sample of ni individuals is drawn without replacement from the total to form a subpopulation, the distribution of the three genotypes as a function of P,, P,, P,, and pis: Estimation fortunately does not require evaluation of the combinations in (4). ROTHMAN, SINGand TEMPLETON(1974) give the log-likelihood for N subdivisions under this model: N 3 n,,-l N ni-l InL(Pl,P,,p) =,z z In[(l-p)Pj+pk] --.Z I: ln(1-p+pk). (5) 1=1 3=1 k=O z=1 k=o A computer program is available to search for the values of P,, P,, and p which maximize this likelihood, given the genotype numbers (nij)in each subdivision. Since a single biological model underlies all the procedures, P,, P,, and p in RST may be considered a reparameterization of WRIGHT’SFIT and FsT.
Details
-
File Typepdf
-
Upload Time-
-
Content LanguagesEnglish
-
Upload UserAnonymous/Not logged-in
-
File Pages17 Page
-
File Size-