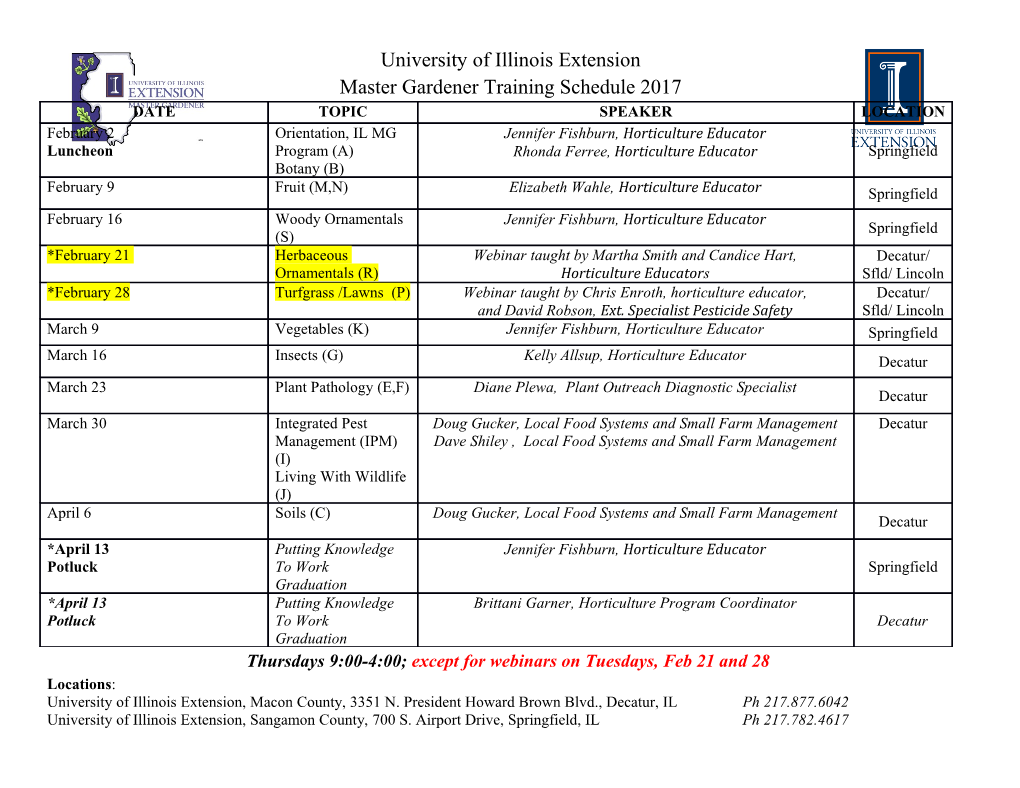
Penalized maximum likelihood estimation of finite mixture models∗ Sofya Budanovay November 12, 2016 Abstract Economic models often resort to finite mixtures to accommodate unobserved heterogeneity. In practice, the number of components in the mixture is rarely known. If too many components are included in the estimation, then the parameters of the estimated model are not point- identified and lie on the boundary of the parameter space. This invalidates the classic results on maximum likelihood estimation. Nonetheless, the parsimonious model, which corresponds to a particular subset of the identified set, can be point-identified. I propose a method to estimate finite mixture models with an unknown number of components by maximizing a penalized likelihood function, where the penalty is applied to the mixing coefficients. The resulting Order- Selection-Consistent Estimator (OSCE) consistently estimates the true number of components in the mixture, and achieves the oracle efficiency for the parameters of the parsimonious model. This paper extends the literature on penalized estimation to the case of non-identified model parameters. Further, numerical simulations illustrate the performance of the proposed method in practice. Finally, the method is applied to the experimental data from Cornand and Heinemann [2014] to determine the composition of subjects’ types associated with their level of rationality in a coordination game. Keywords: finite mixtures, penalized maximum likelihood, SCAD, MCP, non-identification. JEL Classification: C13, C18, C52. ∗The latest version can be found at http://sites.northwestern.edu/sbg354/ yDepartment of Economics, Northwestern University (e-mail: [email protected]). I would like to thank Ivan Canay and Elie Tamer for being great mentors and their support. This paper has benefited a lot from the comments of Joel Horowitz, Gaston Illanes, Charles Manski, Alexander Torgovitsky, and many participants of the econometrics workshop at Northwestern University. I am also very grateful to Davide Cianciaruso, Sergey Gitlin, Eric Mbakop, Shruti Sinha, Yi Sun, and the other members of the EMG. 1 1 Introduction Finite mixtures are used to model situations where data comes from a distribution that can be represented as a convex combination of finitely many other densities. That is, the density of the data generating process in a finite mixture model can be written as h(x; ') ≡ π1f(x; θ1) + π2f(x; θ2) + ::: + πK f(x; θK ); where the densities f(x; θ) belong to some family of distributions which can be indexed by a p- dimensional parameter θ (p is assumed to be finite), K is the order of the mixture and denotes the number of distinct components, and πk are the mixing coefficients that are strictly positive and sum PK up to 1, i.e. k=1 πk = 1. Finite mixtures are an element of many economic models. They help to model heterogeneity of agents, workers, consumers, economic regimes, etc. If the order of the mixture is known, ways to estimate the model parameters are well studied. In particular, the theory of efficient likelihood estimation (see Lehmann and Casella[1998]) can be used to show the existence of a consistent estimator of the model parameters and establish its asymptotic normality. For an extensive survey of the literature on finite mixtures see McLachlan and Peel[2004]. However, in many applications in practice there is no ex-ante information on what the number of components is. This gives rise to one of the two issues: if the estimated model has fewer components than the truth, the model will be misspecified, whilst if the estimated model contains more components than the the true one, then the parameters of the estimated model will no longer be point-identified. This paper considers the latter situation; in particular, it is assumed that although the researcher does not know the value of K, he has an idea on its upper bound, K¯ (see Assumption (M1) in the Section 4.1.1), and estimates a K¯ -component mixture: π1f(x; θ1) + π2f(x; θ2) + ::: + πK¯ f(x; θK¯ ): In this large model some of the mixing coefficients πk are zero, and hence, this is a situation with parameters being on the boundary of the parameter space. As this usually leads to the nonstandard asymptotic distribution of the maximum likelihood estimator (MLE), a bigger issue 2 in this situation is the loss of point-identification of the vector of this model parameters, which is due to the multiplicity of ways in which the true K-component mixture can be written as a K¯ - component mixture (see Example1). It is a problem because point-identification of the parameters is the crucial assumption for establishing consistency and asymptotic normality of the maximum likelihood estimator (see Section 6.3 in Lehmann and Casella[1998]). Redner[1981] showed how a generalized version of consistency of the MLE can be established in such a situation by proving its convergence to the identified set (see Definition1). Inference in this context is discussed, for example, in Liu and Shao[2003] and Chen et al.[2014]. Despite the parameter vector of the large model not being identified as a whole, there exists an object, which is of a particular interest, and that is point-identified in this situation. This object is called the parsimonious model (see Definition2), and it is the mixture with the smallest number of distinct components that all have non-zero mixing coefficients. The parsimonious model has a tight connection with the true K-component mixture: the number of non-zero mixing coefficients in the parsimonious model is exactly K, and the parameters of the components that correspond to the non-zero weights coincide with the components parameters of the true K-component mixture. This paper focuses on the estimation of the parsimonious model. Note that once the parsimonious model becomes the object of interest the vector of the model parameters can be partitioned in two subvectors: 1) (θ1; π1), the parameters of the parsimonious model (this way π1 is a vector of length K and contains strictly positive elements, and (θ1; π1) = '), and 2) (π2; θ2), where π2 = 0, and θ2 is completely non-identified. It means that the parsimonious model is represented by a subset of the identified set (for an example, see Figure1). This paper proposes Order-Selection-Consistent Estimator (OSCE) – an estimator of the model parameters that converges to the parsimonious part of the identified set; and, moreover, with probability approaching one, it has exactly K non-zero elements in the subvector of estimators of the mixing coefficients (in other words, π^2 = 0). The OSCE is defined as the maximizer of the penalized log-likelihood function (see Equation4), where the penalty is put on the mixing coefficients.1 The reason for considering this particular penalization is as follows. Penalizing mixing 1The idea to use a penalty function applied to the mixing coefficients comes from the vast literature on the simultaneous selection of the relevant regressors and estimation of the model parameters in the linear regression models (e.g. Tibshirani[1996], Fan and Li[2001], Knight and Fu[2000], Zhang[2010a], Zou[2006], Huang et al. [2008]). Discussion of the connection of the current paper to this literature is provided in Section3. 3 coefficients guarantees that all the points in the parsimonious subset of the identified set yield the same value of the objective function, and that this value is larger than at any other point from the identified set (see Figure4). This allows to discriminate between different parts of the identified set and achieve convergence to the parsimonious subset. Theorem1 states the conditions that ensure existence of the OSCE and its convergence to the parsimonious part of the identified set (at n−1=2- rate), and Theorem2 proves that the OSCE consistently estimates K and achieves the so-called oracle efficiency for the identifiable parameters of the parsimonious model (namely, θ1 and π1). In other words, the fact that K is estimated consistently allows to estimate the parameters of the parsimonious model as efficiently as in the case with K being known. The main step of the proof of Theorem1 consists in showing that the objective function evaluated at any point at the boundary of a n−1=2-neighborhood of the parsimonious subset of the identified set is smaller than the objective function evaluated at any point in this subset. Since the parameters of the model are not point-identified, the standard proof of a similar statement from Lehmann and Casella[1998] (for MLE) or Fan and Li[2001] (for penalized maximum likelihood estimator with point-identified model parameters) cannot be applied directly. In fact, it is the penalty part of the objective function that plays a crucial role in restoring the validity of this step, by ensuring the aforementioned relation for those points on the boundary, for which the standard argument would fail (see Section4 for a more detailed discussion). To implement the OSCE in practice I propose a data-driven way to select the tuning parameter for the penalty function, and modify the standard EM algorithm, in order to accommodate the penalty term of the objective function (which depends on the mixing coefficients). To illustrate the applicability of my method, I use the data from Cornand and Heinemann’s experiment. They test a coordination game studied theoretically in Morris and Shin[2002]. Coor- dination games are important as their analysis have implication for social welfare and thus, might lead to policy recommendations. However, the results in Morris and Shin[2002] rely on the players using the equilibrium strategy. Playing the Nash equilibrium in this game requires an infinite level of reasoning, and the experimental literature that studied similar games (e.g. Nagel[1995]) discov- ered that subjects often exhibit bounded reasoning behaviour.
Details
-
File Typepdf
-
Upload Time-
-
Content LanguagesEnglish
-
Upload UserAnonymous/Not logged-in
-
File Pages73 Page
-
File Size-