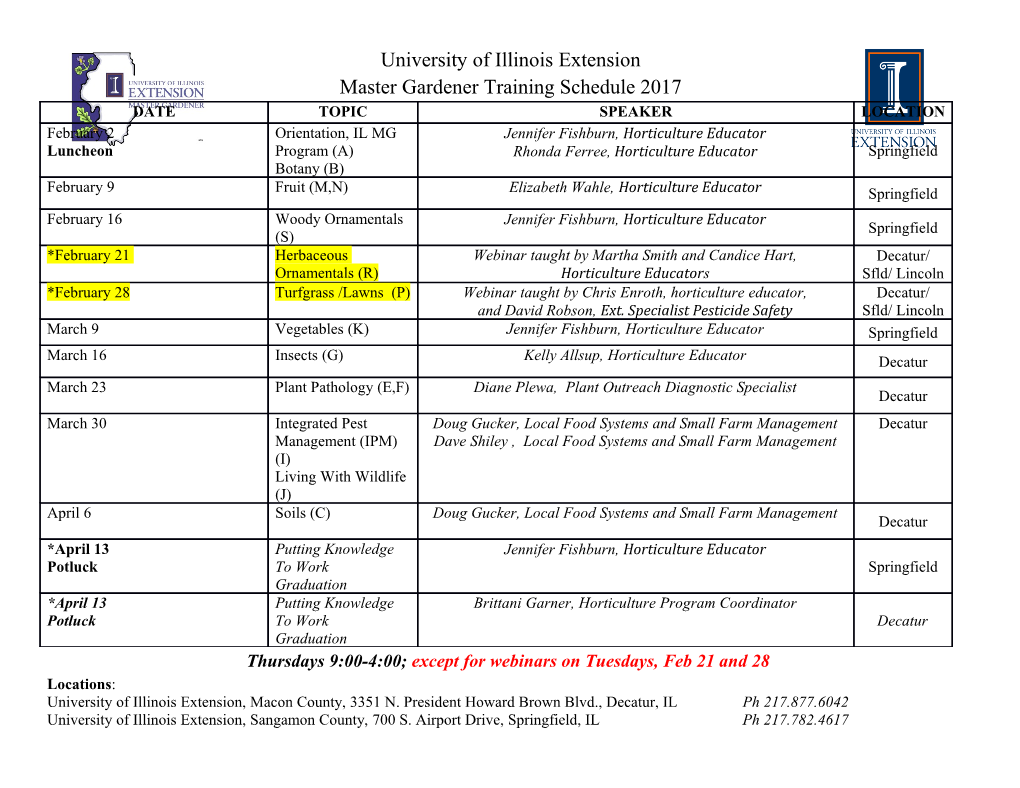
Research Collection Educational Material Software for numerical linear algebra Author(s): Arbenz, Peter; Chinellato, Oscar; Gutknecht, Martin H.; Sala, M. Publication Date: 2006 Permanent Link: https://doi.org/10.3929/ethz-a-005195943 Rights / License: In Copyright - Non-Commercial Use Permitted This page was generated automatically upon download from the ETH Zurich Research Collection. For more information please consult the Terms of use. ETH Library Contents 1 Introduction 1 I Dense Linear Algebra 3 2 Basics 4 2.1 Notation . 4 2.2 Statement of the problem . 5 2.3 Similarity transformations . 7 2.4 Schur decomposition . 8 2.5 The real Schur decomposition . 9 2.6 Hermitian matrices . 10 2.7 Cholesky factorization . 13 2.8 The singular value decomposition (SVD)F . 14 3 The QR Algorithm 17 3.1 The basic QR algorithm . 17 3.1.1 Numerical experiments . 18 3.2 The Hessenberg QR algorithm . 20 3.2.1 A numerical experiment . 22 3.2.2 Complexity . 23 3.3 The Householder reduction to Hessenberg form . 24 3.3.1 Householder reflectors . 24 3.3.2 Reduction to Hessenberg form . 24 3.4 Improving the convergence of the QR algorithms . 26 3.4.1 A numerical example . 27 3.4.2 QR algorithm with shifts . 28 3.4.3 A numerical example . 29 3.5 The double shift QR algorithm . 30 3.5.1 A numerical example . 33 3.5.2 The complexity . 34 3.6 The symmetric tridiagonal QR algorithm . 35 3.6.1 Reduction to tridiagonal form . 35 3.6.2 The tridiagonal QR algorithm . 36 3.7 Summary . 36 4 Cuppen’s Divide and Conquer Algorithm 40 4.1 The divide and conquer idea . 40 4.2 Partitioning the tridiagonal matrix . 41 4.3 Solving the small systems . 41 4.4 Deflation . 42 4.4.1 Numerical examples . 43 ii 4.5 The eigenvalue problem for D + ρvvT . 44 4.6 Solving the secular equation . 47 4.7 A first algorithm . 48 4.7.1 A numerical example . 48 4.8 The algorithm of Gu and Eisenstat . 51 4.8.1 A numerical example [continued] . 52 5 LAPACK and the BLAS 57 5.1 LAPACK . 57 5.2 BLAS . 58 5.2.1 Typical performance numbers for the BLAS . 58 5.3 Blocking . 59 5.4 LAPACK solvers for the symmetric eigenproblems . 61 5.5 Generalized Symmetric Definite Eigenproblems (GSEP) . 63 5.6 An example of a LAPACK routines . 63 II Sparse Linear Algebra 71 6 Finite Element Discretisations of Elliptic PDEs 72 6.1 Model Problem . 72 6.1.1 Derivation of a Weak Form . 73 6.1.2 Of Meshes, Element Functions and Discrete Operators . 74 6.1.3 Assembling the Parts . 77 6.2 A MATLAB implementation . 78 6.3 The Solution — Assessing Correctness . 81 7 Storage Schemes for Sparse Matrices 86 7.1 Compressed Sparse Row (CSR), Compressed Sparse Column (CSC) . 86 7.2 Modified Sparse Row (MSR), Modified Sparse Column (MSC) . 88 7.3 Coordinate Format (AIJ) . 88 7.4 Linked List Format . 89 7.5 H –MatricesF . 89 8 Reorderings and Sparse Direct Solvers 92 8.1 Fill-in Reducing (and other) Reorderings . 92 8.2 Direct Solvers . 94 8.3 Available Software . 94 9 Iterative Solvers 98 9.1 Fixed Point and Jacobi Iteration . 98 9.2 Iterations Based on Matrix Splittings . 101 9.3 Krylov Subspaces and Krylov Space Solvers . 104 9.4 Chebyshev IterationF . 108 9.5 Preconditioning . 112 9.6 The Conjugate Gradient Method . 113 9.6.1 Energy Norm Minimization . 113 9.6.2 Steepest Descent . 114 9.6.3 Conjugate Direction Methods . 115 9.6.4 The Conjugate Gradient (CG) method . 117 9.6.5 The Conjugate Residual (CR) Method . 122 9.6.6 A Bound for the Convergence . 124 9.6.7 Preconditioned CG Algorithms . 124 9.6.8 CG and CR for Complex SystemsF . 126 iii 9.6.9 CG for Least Squares ProblemsF . 126 9.7 The Symmetric Lanczos ProcessF . 129 9.7.1 The Lanczos Process and Its Relation to the CG MethodF . 129 9.7.2 Eigenvalue Computations With the Symmetric Lanczos ProcessF . 133 9.7.3 Solving the System in Coordinate SpaceF . 134 9.7.4 Further Topics Related to the CG Method and the Lanczos ProcessF . 137 9.8 Solving the System in Coordinate Space . 137 9.8.1 The Arnoldi Process . 137 9.8.2 The Transformation to Coordinate Space . 141 9.8.3 GMRES . 142 9.8.4 MINRES . 145 9.8.5 FOMF . 147 F 9.8.6 SYMMLQ . 147 10 Preconditioning 153 10.1 Preconditioning based on classical matrix splittings . 154 10.2 Incomplete LU and Cholesky factorizations . 155 10.3 Polynomial preconditioning . 157 10.4 Inner-outer iteration . 157 10.5 Sparse Approximate Inverse Preconditioners (SPAI)F . 157 10.6 Domain Decomposition Preconditioners . 158 10.6.1 One-level Schwarz Preconditioners . 160 10.6.2 Two-level Schwarz Preconditioners . 162 10.7 Multigrid Preconditioners . 164 11 Finite Difference and Finite Element Discretisations of Eigenvalue ProblemsF 170 11.1 What makes eigenvalues interesting? . ..
Details
-
File Typepdf
-
Upload Time-
-
Content LanguagesEnglish
-
Upload UserAnonymous/Not logged-in
-
File Pages233 Page
-
File Size-