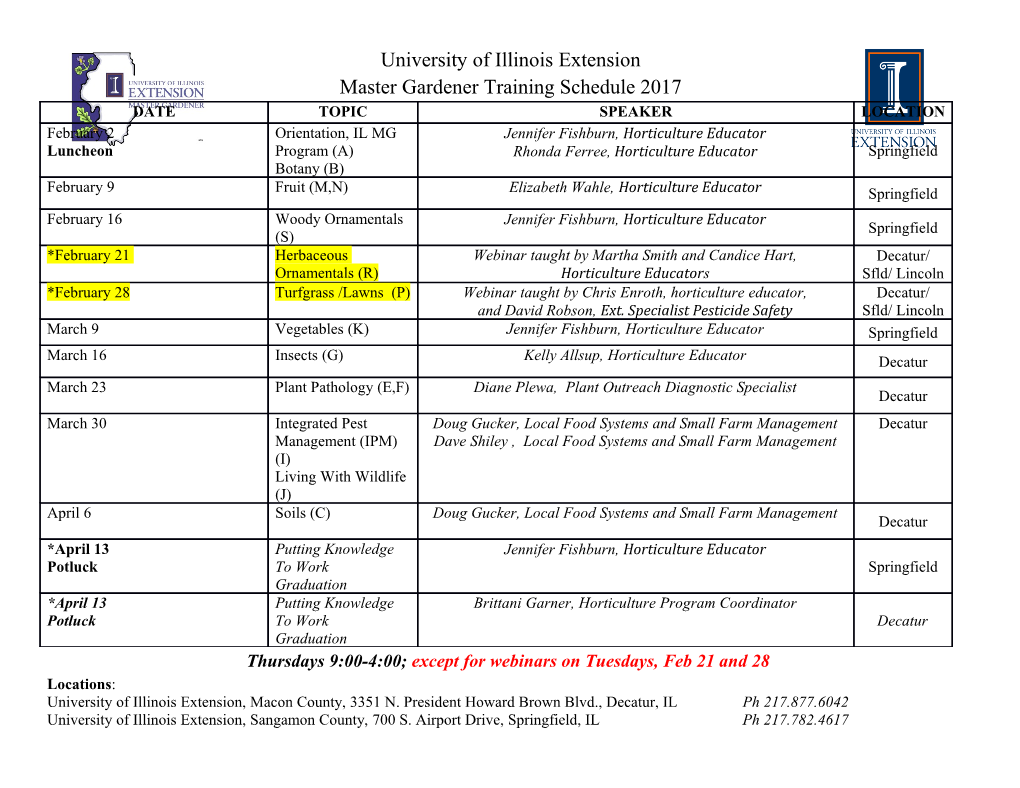
A Bayesian Reanalysis of Results from the Enhanced Services for the Hard-to-Employ Demonstration and Evaluation Project OPRE Report 2012-36 June 2012 Office of Planning, Research and Evaluation (OPRE) Administration for Children and Families U.S. Department of Health and Human Services Office of the Assistant Secretary for Planning and Evaluation U.S. Department of Health and Human Services A Bayesian Reanalysis of Results from the Hard-to-Employ Demonstration and Evaluation Project OPRE Report 2012-36 June 2012 Author: Charles Michalopoulos, MDRC Submitted to: Girley Wright, Project Officer Office of Planning, Research and Evaluation Administration for Children and Familiees U.S. Department of Health and Human Services Kristen Joyce and Laura Radell, Project Officers Assistant Secretary for Planning and Evaluation U.S. Department of Health and Human Services Project Director: Davvid Butler MDRC 16 East 34 Street New York, NY 10016 Contract Number: HHS-233-01-0012 This report is in the public domain. Permission to reproduce is not necessary. Suggested citation: Charles Michalopoulos (2012). A Bayesian Reanalysis of Results from the Hard-to-Employ Demonstration and Evaluation Project. OPRE Report 2012-3 6. Washington, DC: Office of Planning, Research and Evaluation, Administration for Children and Families, U.S. Department of Health and Human Services. Disclaimer: The views expressed in this publication do not necessarily reflect the views oor policies of the Office of Planning, Research and Evaluaation, the Admministration for Children and Families, or the U.S. Department of Health and Human Services. This report and other reports sponsored by the Office of Planning, Research and Evaluation are available at http://acf.gov.programs/opre/index.html. MDRC is conducting the Enhanced Services for the Hard-to-Employ Demonstration and Evalua- tion Project under a contract with the Administration for Children and Families (ACF) and the Office of the Assistant Secretary for Planning and Evaluation (ASPE) in the U.S. Department of Health and Human Services (HHS), funded by HHS under a competitive award, Contract No. HHS-233-01-0012. The project officers are Girley Wright (ACF) and Kristen Joyce and Laura Radel (ASPE). Additional funding has been provided by the U.S. Department of Labor (DOL). DataStat, a subcontractor, fielded the six-month client survey for the Rhode Island site. The findings and conclusions in this report do not necessarily represent the official positions or policies of HHS. Dissemination of MDRC publications is supported by the following funders that help finance MDRC’s public policy outreach and expanding efforts to communicate the results and implica- tions of our work to policymakers, practitioners, and others: The Annie E. Casey Foundation, The George Gund Foundation, Sandler Foundation, and The Starr Foundation. In addition, earnings from the MDRC Endowment help sustain our dissemination efforts. Con- tributors to the MDRC Endowment include Alcoa Foundation, The Ambrose Monell Foundation, Anheuser-Busch Foundation, Bristol-Myers Squibb Foundation, Charles Stewart Mott Founda- tion, Ford Foundation, The George Gund Foundation, The Grable Foundation, The Lizabeth and Frank Newman Charitable Foundation, The New York Times Company Foundation, Jan Nichol- son, Paul H. O’Neill Charitable Foundation, John S. Reed, Sandler Foundation, and The Stupski Family Fund, as well as other individual contributors. For information about MDRC and copies of our publications, see our Web site: www.mdrc.org. This page intentionally left blank. Abstract Social policy evaluations usually use classical statistical methods, which may, for example, compare outcomes for program and comparison groups and determine whether the estimat- ed differences (or impacts) are statistically significant — meaning they are unlikely to have been generated by a program with no effect. This approach has two important shortcom- ings. First, it is geared toward testing hypotheses regarding specific possible program ef- fects — most commonly, whether a program has zero effect. It is difficult with this frame- work to test a hypothesis that, say, the program’s estimated impact is larger than 10 (whether 10 percentage points, $10, or some other measure). Second, readers often view results through the lens of their own expectations. A program developer may interpret re- sults positively even if they are not statistically significant — that is, they do not confirm the program’s effectiveness — while a skeptic might interpret with caution statistically sig- nificant impact estimates that do not follow theoretical expectations. This paper uses Bayesian methods — an alternative to classical statistics — to reanalyze results from three studies in the Enhanced Services for the Hard-to-Employ (HtE) Demon- stration and Evaluation Project, which is testing interventions to increase employment and reduce welfare dependency for low-income adults with serious barriers to employment. In interpreting new data from a social policy evaluation, a Bayesian analysis formally incorpo- rates prior beliefs, or expectations (known as “priors”), about the social policy into the sta- tistical analysis and characterizes results in terms of the distribution of possible effects, in- stead of whether the effects are consistent with a true effect of zero. The main question addressed in the paper is whether a Bayesian approach tends to confirm or contradict published results. Results of the Bayesian analysis generally confirm the pub- lished findings that impacts from the three HtE programs examined here tend to be small. This is in part because results for the three sites are broadly consistent with findings from similar studies, but in part because each of the sites included a relatively large sample. The Bayesian framework may be more informative when applied to smaller studies that might not be expected to provide statistically significant impact estimates on their own. v This page intentionally left blank. Contents Abstract v List of Exhibits ix Introduction 1 An Introduction to Bayesian Ideas 2 Extending Bayes’ Rule to a Social Policy Evaluation 4 Why Use Bayesian Updating? 8 Examples of Bayesian Analysis in Random Assignment Studies 11 Estimating Mean Impacts 11 Comparing Subgroups or Sites 12 Overview of the Paper 13 Rhode Island: Working toward Wellness 14 Forming Priors for Working toward Wellness 15 Results of the Bayesian Analysis for Working toward Wellness 17 Full Sample Impacts 17 Subgroup Differences 21 Working toward Wellness: Discussion 25 New York and Philadelphia: Transitional Jobs 25 New York: Center for Employment Opportunities 26 Related Studies 27 Results of the Bayesian Analysis for CEO 31 Philadelphia: Transitional Work Corporation 34 Related Studies 35 Results of the Bayesian Analysis for TWC 38 Transitional Jobs: Discussion 40 Conclusion 41 References 43 Earlier Publications on the Enhanced Services for the Hard-to-Employ Demonstration and Evaluation Project 47 vii This page intentionally left blank. List of Exhibits Table 1 Probabilities of Women Age 40 Having Breast Cancer and Screening Positive on a Mammogram 3 2 Bayesian Analysis of Hypothetical Earnings Impacts with Different Priors 9 3 Estimated Effects of Working toward Wellness at Six Months: Summary of Posterior Distribution Using Bayesian Analysis 18 4 Estimated Effects of Working toward Wellness at Six Months for Hispanic and Non-Hispanic Subgroups: Summary of Posterior Distribution Using Bayesian Analysis 23 5 Impacts on Employment and Recidivism in Transitional Jobs Programs for Former Prisoners 29 6 Estimated Impacts on Employment, Center for Employment Opportunities: Summary of Classical and Bayesian Analyses 32 7 Estimated Impacts on Arrests, Convictions, and Incarceration, Center for Employment Opportunities: Summary of Posterior Distributions Using Bayesian Analysis 33 8 Impacts on Employment and Welfare Receipt, Subsidized Employment for Welfare Recipients 37 9 Estimated Effects on Employment and Welfare Receipt, Subsidized Employment for Welfare Recipients: Summary of Bayesian Posterior Distributions 39 Figure 1 Three Prior Distributions of the Effects on Earnings 6 ix This page intentionally left blank. Introduction Social policy evaluations almost always draw inferences using frequentist or classical statistical methods. An evaluator may, for example, compare outcomes for program and comparison groups and make statements about whether estimates are statistically significant, which means they are unlikely to have been generated by a program with a true effect of zero. This approach has two important shortcomings. First, it is geared toward testing hy­ potheses regarding specific possible program effects. Most commonly, inferences are made about whether a program has zero effect. It is difficult with this framework to test a hypothesis that, say, the program’s impact is larger than 10 (whether 10 percentage points, $10, or some other measure). Second, readers often view results through the lens of their own expectations. A program developer may interpret positive results that are not statistically significant as confir­ mation of the program’s effectiveness, while a skeptic might interpret with caution statistically significant impact estimates that do not follow theoretical expectations. An alternative paradigm that addresses both of these shortcomings is provided by Bayesian statistics. In interpreting new data from a social policy evaluation, a Bayesian analyst would formally incorporate
Details
-
File Typepdf
-
Upload Time-
-
Content LanguagesEnglish
-
Upload UserAnonymous/Not logged-in
-
File Pages58 Page
-
File Size-