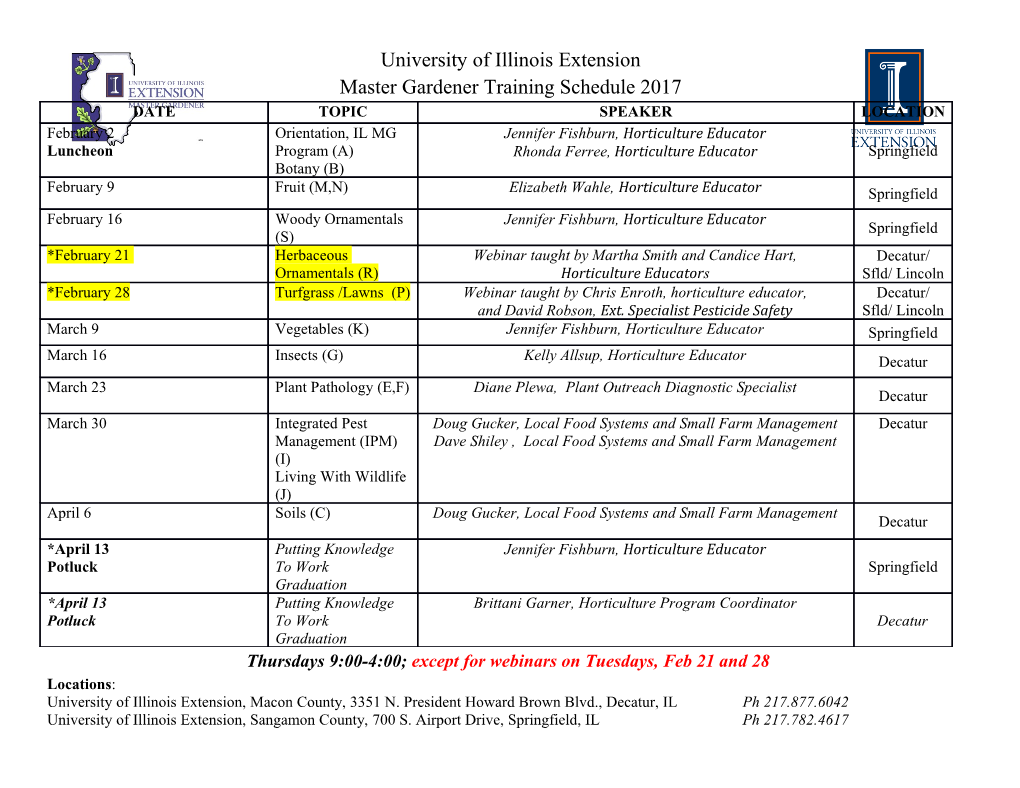
RELATIONS BETWEEN INFORMATION AND ESTIMATION: A UNIFIED VIEW A DISSERTATION SUBMITTED TO THE DEPARTMENT OF ELECTRICAL ENGINEERING AND THE COMMITTEE ON GRADUATE STUDIES OF STANFORD UNIVERSITY IN PARTIAL FULFILLMENT OF THE REQUIREMENTS FOR THE DEGREE OF DOCTOR OF PHILOSOPHY Kartik Venkat December 2015 © 2015 by Kartik Venkat. All Rights Reserved. Re-distributed by Stanford University under license with the author. This work is licensed under a Creative Commons Attribution- Noncommercial 3.0 United States License. http://creativecommons.org/licenses/by-nc/3.0/us/ This dissertation is online at: http://purl.stanford.edu/pv323kq3283 ii I certify that I have read this dissertation and that, in my opinion, it is fully adequate in scope and quality as a dissertation for the degree of Doctor of Philosophy. Tsachy Weissman, Primary Adviser I certify that I have read this dissertation and that, in my opinion, it is fully adequate in scope and quality as a dissertation for the degree of Doctor of Philosophy. Peter Glynn I certify that I have read this dissertation and that, in my opinion, it is fully adequate in scope and quality as a dissertation for the degree of Doctor of Philosophy. Andrea Montanari Approved for the Stanford University Committee on Graduate Studies. Patricia J. Gumport, Vice Provost for Graduate Education This signature page was generated electronically upon submission of this dissertation in electronic format. An original signed hard copy of the signature page is on file in University Archives. iii Abstract Measures of information, such as entropy, mutual information, and relative entropy play important roles in various domains: underlying algorithmic procedures in ma- chine learning, characterizing operational quantities in communication, storage and inference, and characterizing the likelihood of large deviations events in statistics and probability, among others. Such information measures also turn out to give a quantitative understanding of several estimation theoretic notions - such as the costs of causality and mismatch, and the benefit of lookahead - via relations between in- formation and estimation. This thesis presents a new framework for understanding the rich interconnections between information and estimation for a variety of widely applicable observation models. Many of these relations can be viewed as identities between expectations of ran- dom quantities. This dissertation presents these identities and their generalizations in new light. The first part of this thesis focusses on two canonical observation mod- els: The additive Gaussian channel and the Poisson channel. For both these models, we dispense with the expectations and explore the nature of the pointwise relations between the respective random quantities. Our results recover and generalize pre- viously known identities involving mutual information and minimum mean loss in estimation. Classical estimation and information theoretic quantities emerge with new and surprising roles. In the second part of the thesis, we introduce a natural family of L´evychannels where the distribution of the output conditioned on the input is infinitely divisible. This family includes, as special cases, both the Gaussian and Poisson channels. For L´evychannels, we establish new representations relating the mutual information between the channel input and output to an optimal expected iv estimation loss, thereby unifying and extending previously known results. Corollaries of our results include new formulae for entropy and relative entropy. The unified framework extends to continuous-time stochastic processes as well, where we intro- duce and study these connections for semi-martingale channels, which include the well-known white Gaussian channel and the multivariate point process channel. In the third, and final part of this dissertation, we revisit the white Gaussian channel and study the problem of estimation with finite lookahead. We look at the properties of finite lookahead minimum mean squared error and investigate connections between this quantity and mutual information. v To Mummy, Daddy, Ammai, Appa, and Kavya { for your eternal blessings and love Acknowledgments Over the last five years at Stanford, the one thing I cherish has been the unrestricted access to the wisdom, support, encouragement, and knowledge of my adviser, Tsachy Weissman. In Tsachy, I found a friend, a parent, and a guru who gave me so much more than whatever I hoped for. Tsachy has helped me identify important research questions, taught me how to approach them, has given me unconstrained freedom to explore new technical areas, honed my skills in disseminating research, and simply cared for everything that has been important to me, personally and professionally. I want to thank Tsachy Weissman, for everything. As an adviser and a teacher, he has gone beyond the call of duty on all fronts. Tsachy is my role model in life, and I feel privileged to have this bond. I have been fortunate to have wonderful mentors who have helped me develop as a researcher over these years, by taking a deep and personal interest in me, and giving me the gift of knowledge and encouragement for which I am indebted to them. With all my heart, I would like to thank Thomas Cover, my co-adviser, who demonstrated the importance of being • guided by beauty in research, and made me fall in love with information theory. Shlomo Shamai, for helping me identify important research problems (some that • helped shape this thesis), for collaborating closely even though we are continents apart, and for being a reservoir of knowledge and encouragement throughout. Andrea Goldsmith, for her boundless energy and enthusiasm for research, for • her enjoyable course on wireless communications, and for her constant guidance and support. vii Thomas Courtade, for the many stimulating white-board discussions, and for • being a wonderful collaborator and friend. Abbas El Gamal, for his course on network information theory, for offering • me great advice whenever I needed it, and for helping me understand what constitutes good research. I would also like to thank Haim Permuter for our interactions over these years during his regular visits to Stanford, and Ayfer Ozg¨urfor¨ the remarkable course on wireless information theory as well as the valuable one-one discussions. I am particularly grateful to Andrea Montanari and Peter Glynn, who graciously agreed to be part of my reading committee. I would also especially like to thank my lab-mate Jiantao Jiao for our wonderful partnership in research which led to many interesting research problems and intellectual discussions, and for being a constant source of technical inspiration during my PhD. The Information Systems Lab at Stanford is a place where I have forged friendships that will last a lifetime. I would like to thank various members of the ISL community whom I have been lucky to interact with, both technically and personally: Albert, Bobbie, Jiantao, Yash, Vinith, Idoia, Himanshu, Ritesh, Mikel, Alexandros, Dinesh, Gowtham, Lei, Yeow-Khiang, Chinmoy, Aditya, Yanjun, Dmitri, and Manu. There are several friends I have been fortunate to have, who haven't featured here but have shared in my journey closely, from near and afar. I thank you all. Finally, I would like to return to where it all began. I am eternally grateful and indebted to my family. My mom especially, has been a pillar of strength and support for me. After the death of my father and grandfather, it was not an easy decision to move so far away from home for the pursuit of knowledge. But my mother, grandmother, and sister have always been cheering for me in the background. Without their encouragement and support, none of this could be possible. I dedicate this thesis to my family. viii Contents Abstract iv Acknowledgments vii 1 Introduction1 2 Pointwise Gaussian 15 2.1 Main Results............................... 16 2.1.1 Scalar Estimation......................... 16 2.1.2 Continuous Time......................... 21 2.2 Scalar Setting: Examples, alternative Couplings, further Observations, and Identities............................... 34 2.2.1 The Original Coupling...................... 34 2.2.2 Additive Standard Gaussian................... 37 2.2.3 Independent Standard Gaussian's................ 38 2.2.4 Comparison of Variances..................... 41 2.2.5 An identity............................ 43 2.3 Proofs................................... 44 2.4 Chapter Summary............................ 64 3 Pointwise Poisson 66 3.1 Preliminaries and Notation........................ 69 3.1.1 The Poisson Channel....................... 69 3.1.2 Minimum Mean Loss Estimation in the Poisson Channel... 71 ix 3.2 Main Results............................... 72 3.2.1 Continuous-time Causal Estimation in the absence of Feedback 72 3.2.2 Continuous-time Causal Estimation where Feedback may be Present.............................. 73 3.2.3 Continuous Time Causal Mismatched Estimation....... 74 3.3 Non-Causal Estimation.......................... 76 3.4 The Scalar Poisson Channel....................... 77 3.5 Chapter summary............................. 81 4 L´evyChannels 82 4.1 Scalar L´evy Channels........................... 86 4.1.1 A short introduction to L´evyProcesses............. 86 4.1.2 The Scalar L´evyChannel..................... 88 4.2 Main Results............................... 91 4.3 Recovering Gaussian and Poisson results................ 95 4.4 Entropy and Relative Entropy: New Formulae............. 97 4.5 Two New Examples: The gamma and negative
Details
-
File Typepdf
-
Upload Time-
-
Content LanguagesEnglish
-
Upload UserAnonymous/Not logged-in
-
File Pages227 Page
-
File Size-