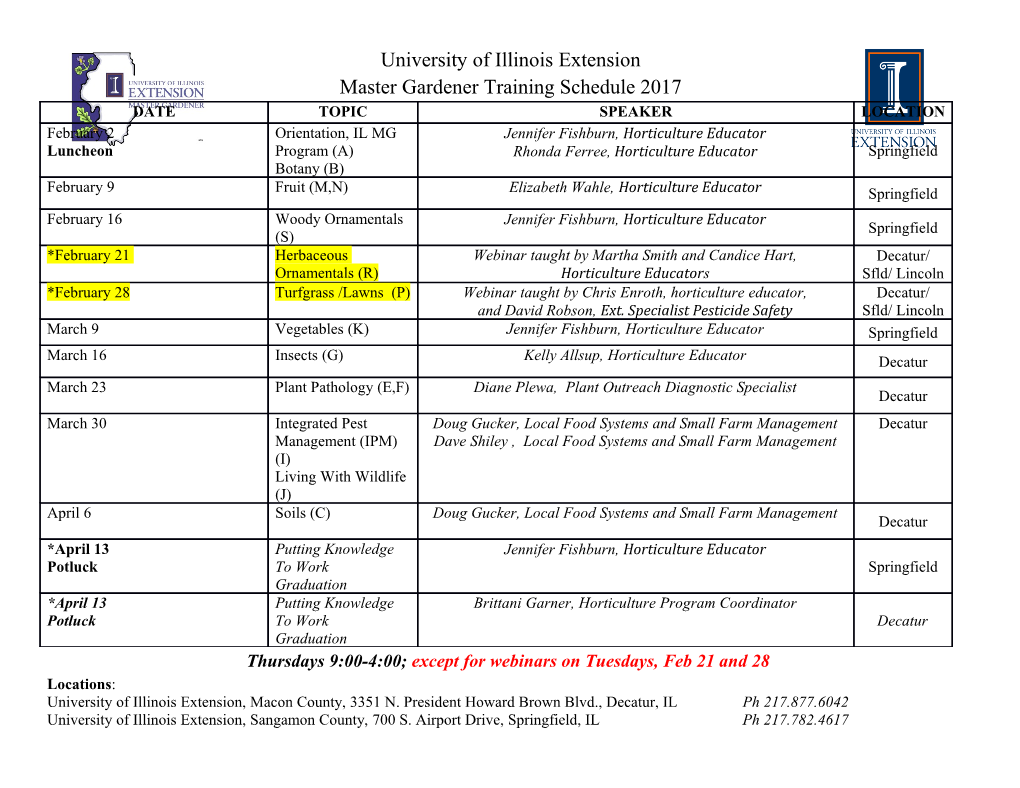
CHAPTER 3.5 The Biogeography of Microbial Communities and Ecosystem Processes: Implications for Soil and Ecosystem Models M ark A . B radford and N oah F ierer Although we might have “faith” that the true eco- 3.5.1 Predicting environmental system response lies somewhere within the predic- responses of soil processes tions from the models, predictions from We are entering a period of rapid and pronounced mathematical models that rely on empirical (or sta- environmental change. Understanding how this tistical) relationships may be much more similar. change will in! uence soil communities is essential Empirical models comprise the majority of ecosys- for accurate prediction of future climate, biogeo- tem models used to predict soil biogeochemical chemical cycles, and human well-being. Such relia- processes (see Box 3.5.1 ). Our con" dence in the pre- ble prediction is the fundamental test of scienti" c dictions by empirical models of future ecosystem understanding (i.e. can we use knowledge of under- response relies on the assumption that observa- lying mechanisms to predict accurately how phe- tions of regulatory variable-process rate relation- nomena change across space and time). Predicting ships hold across space and time ( Reynolds et al. how soils respond to environmental change will be 2001 ). So, we are faced with a dilemma. Do we central to adaptive management of ecosystems, develop and employ mechanistic models that may given our reliance on soils for sustained food pro- be more accurate but also more uncertain or do we duction, water puri" cation, carbon storage, and follow the more traditional approach, relying on nutrient retention. This ability to predict with cer- empirical models that may predict ecosystem proc- tainty how environmental change will in! uence esses with more certainty but less accuracy? There soil processes requires mechanistic understanding is likely no single right answer to this question of how soils work. Developing this mechanistic when developing models to predict how ecosys- understanding is perhaps the “Grand Challenge” tems will respond to environmental change. A fam- for soil ecologists if we are to advance our basic ily of models that covers this continuum of understanding of soils and apply this knowledge to approach—between empiricism and mechanism effective environmental management. –will permit us to determine where model predic- Mechanistic understanding improves our con " - tions agree. Where they disagree likely indicates dence in predictions of the future that extrapolate the greatest uncertainty in model predictions, and observed relationships between regulatory varia- it is in addressing these disagreements that future bles (e.g. temperature) and process rates (e.g. soil research might yield most insight. respiration) (Reynolds et al. 2001 ). Perhaps para- The soil ecology community predominately relies doxically, there may be much less agreement in on more empirical models and this reliance has predicted outcomes between ecosystem models repercussions beyond the discipline and can in! u- when more mechanistic understanding is included. ence global policy. For example, the soil submodels Soil Ecology and Ecosystem Services. First Edition. Edited by Diana H. Wall et al. © 2012 Oxford University Press. Published 2012 by Oxford University Press. 190 SOIL ECOLOGY AND ECOSYSTEM SERVICES Box 3.5.1 Comparison of mechanistic and empirical models Most commonly it is the perspective of an individual, not ecological and biogeochemical sciences—using regression model structure, which determines whether a model is relationships derived from data. When we extrapolate these classed as mechanistic or empirical. In reality, these two relationships we are assuming they are robust across space terms distinguish opposite ends of a continuum. Mechanistic and time, even if the physical, chemical, and biological models are designed to explain outcomes with formulations conditions under which the data were collected differ that represent actual physical, chemical, and biological markedly to those in the new location or time for which we processes, such as increased catalytic rates of respiratory make the prediction. With mechanistic models the argument enzymes as temperature increases. Empirical models are is that—if we understand and represent the underlying often referred to as “correlative or statistical models” and mechanisms accurately in the model formulations—then we are designed to predict—as opposed to explain—outcomes. can make robust predictions for other locations and times. To enable this prediction, formulations are used that best The challenges with mechanistic models are thus twofold: describe relationships between two measured variables, we have to both understand the mechanisms and then be such as temperature and soil respiration rates, with no able to represent them accurately when formulating models. necessary regard for the underlying mechanism. Empirical These challenges are not trivial, and may delimit the very models are typically parameterized—at least in the edges of our understanding of soils. embedded within the coupled atmosphere– actually at odds with the fact the models are usu- biosphere carbon cycle models used by the Inter- ally also implicitly rooted in fundamental biologi- governmental Panel on Climate Change (IPCC) to cal understanding. But what it does mean is that predict future climate are largely empirical. The we assume we can omit biology (and within “biol- IPCC has high uncertainty in some of the assump- ogy” we include ecology) from the models as a tions inherent to the embedded soil models, and force that could shape responses of soil processes this leads to uncertainty in climate prediction. For to perturbation. That is, we assume physicochemi- example, there is marked uncertainty as to whether cal controls on soil processes are unaffected by warmer temperatures will lead to the net loss of soil phenotypic or genotypic changes in biota, and carbon that then generates a positive feedback to biotic interactions, across time and space. Yet at global warming ( Denman et al . 2007 ). Given the vast the same time we’re aware that soil organisms—at published literature on this topic (e.g. Davidson & least in part—mediate many soil processes (e.g. Janssens 2006 ; Bradford et al . 2008 ; Allison et al . respiration and nitri" cation). The omission of biol- 2010 ), it is unsettling that we—the soil ecology com- ogy from soil models is perhaps best illustrated munity—cannot reach agreement on the question through example. Take the approach of using a of how soil carbon stocks will respond to tempera- single, " rst-order decay equation to estimate how ture change, especially given the societal need for the rate of soil carbon decomposition varies with resolution. One plausible reason for this disagree- temperature (e.g. Kirschbaum 2004 ; Knorr et al . ment is that biological and ecological mechanisms 2005 ). The equations are typically parameterized are typically represented implicitly—not by data collected across a large range in one or explicitly—in soil models ( Bahn et al . 2010 ). more controlling factors, in this case temperature. Variation in temperature is generated by use of 3.5.2 Misplaced physics envy broad spatial gradients (e.g. elevation, latitude) or in soil models short temporal changes (e.g. incubators, daily vari- ation). Using these statistical relationships to Soils are not merely physical and chemical entities, project how soil carbon decomposition responds yet we generally model soil processes by assuming to environmental change assumes that the biology they can be represented simply as a product of is invariant across space and time. In other physics and chemistry. This assumption is not words, understanding of biological mechanism is THE BIOGEOGRAPHY OF MICROBIAL COMMUNITIES AND ECOSYSTEM PROCESSES 191 unnecessary for accurately predicting how soil exhibit identical life-history strategies (e.g. Clark processes will respond to environmental change. 2009 ; Rosindell et al . 2010 ). Yet recent work high- Social scientists are well aware of the shortcom- lights that those patterns attributed to this assump- ings of using principles from classical physics to tion of identical strategies can equally well be predict response of human (and hence biological) explained by statistical artefact introduced when systems to perturbation (for an excellent commen- dealing with large data sets, and that the same data tary see Bernstein et al . 2000 ). Application of princi- sets used to make this “probability argument” can ples such as 1) invariance, 2) probability, and actually only have arisen through complex (i.e. eco- 3) simplicity “promise” a level of certainty in pre- logical niche) processes ( Warren et al . 2011 ). In other diction that will not be realized where biological words, the assumption of probability might accu- processes in! uence outcomes. With invariance (1) rately predict patterns (or process rates) that we we assume that history does not matter, with observe, but for the wrong mechanistic reasons. responses a " xed function of controlling variables. Finally, with simplicity (3) the assumption is that In human systems, we can understand that “experi- only a few variables in! uence outcomes and ence” in! uences decision-making, so outcomes of that these variables can be measured with accuracy. perturbations differ if a person is naïve or familiar Yet even if simplicity holds then phenomena that with the event. Soil organisms may
Details
-
File Typepdf
-
Upload Time-
-
Content LanguagesEnglish
-
Upload UserAnonymous/Not logged-in
-
File Pages12 Page
-
File Size-