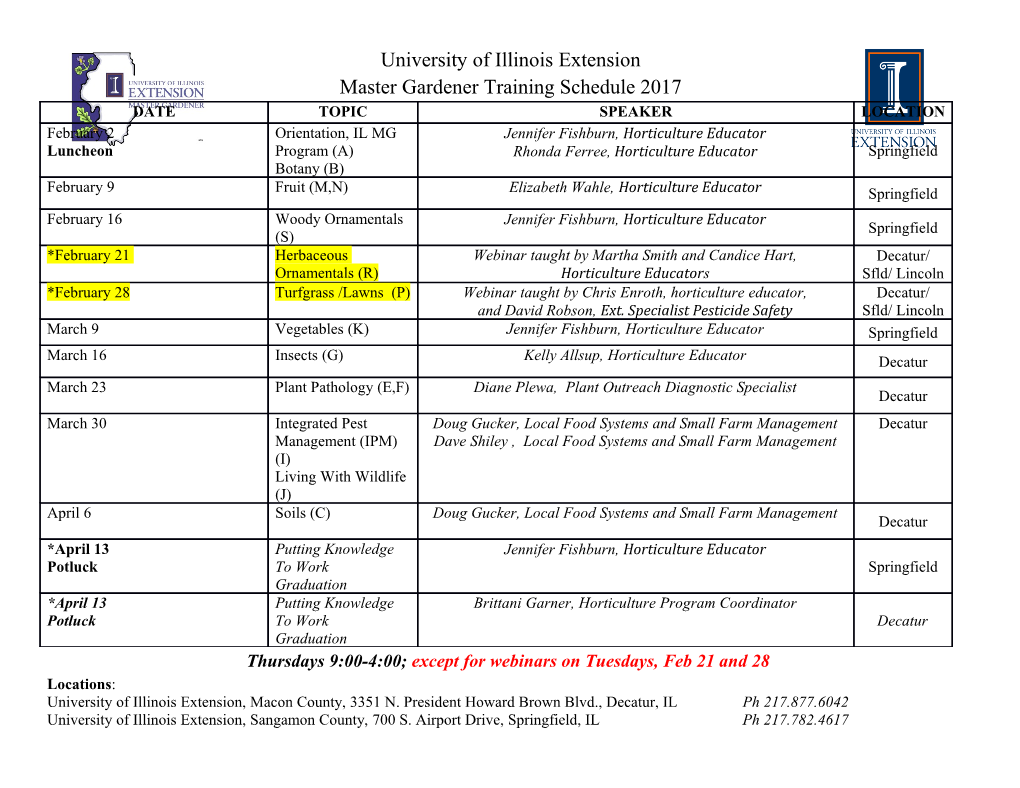
Index ˛, see significance level deep learning, 192 ˇ2, see kurtosis Asimov dataset, 231 , see unnormalized skewness asymmetric errors, 99, 110 1, see skewness combination of, 123 2, see excess asymptotic formulae for test statistics, ", see efficiency 231 . see Gaussian average value or signal average value strength continuous case, 27 , see correlation coefficient discrete case, 12 , see standard deviation or Gaussian standard in Bayesian inference, 69 deviation , see lifetime ˚, see Gaussian cumulative distribution back propagation, neural network, 191 2 background distribution, 32 dependence of Feldman–Cousins upper method, 114 limits, 218, 220 binned case, 119 determination from control regions, in multiple dimensions, 132 129 random variable, 32, 114, 120 fluctuation for significance level, 205 Baker–Cousins, 120 in convolution and unfolding, 160 Neyman’s, 119 modeling in extended likelihood, 107 Pearson’s, 119 modeling with Argus function, 43 , see sample space rejection in hypothesis test, 176 3 evidence, 207 treatment in iterative unfolding, 171 5 observation, 207 uncertainty in significance evaluation, 209 uncertainty in test statistic, 227, 236 Baker–Cousins 2, 120 activation function, 191 Bayes factor, 73 adaptive boosting, 198 Bayes’ theorem, 59 AI, artificial intelligence, 195 learning process, 67 alternative hypothesis, 175 Bayesian Anderson–Darling test, 184 inference, 68 Argus function, 43 probability, 59, 64 artificial intelligence, 195 visual derivation, 60 artificial neural network, 181, 190 unfolding, 166 © Springer International Publishing AG 2017 251 L. Lista, Statistical Methods for Data Analysis in Particle Physics, Lecture Notes in Physics 941, DOI 10.1007/978-3-319-62840-0 252 Index BDT, see boosted decision trees Clopper–Pearson binomial interval, 147 Bernoulli CLs method, 221 probability distribution, 17 CNN, see convolutional neural network random process, 16 coefficient of determination R2, 117 random variable, 17 combination Bertrand’s paradox, 7 of measurements, 129 best linear unbiased estimator, 133 principle, 136, 140 conservative correlation assumption, 137 conditional intrinsic information weight, 136 distribution, 53 iterative application, 139 probability, 9 marginal information weight, 136 confidence negative weights, 135, 137 interval, 100, 109, 143 relative importance, 136 level, 100 beta distribution, 83 conservative bias, 102 CLs method, 221, 223 in maximum likelihood estimators, 113 correlation assumption, BLUE method, 137 bifurcated Gaussian, 124 interval, 147 bimodal distribution, 14 limit, 217 bin migration, 158 consistency of an estimator, 102 binned Poissonian fit, 120 control binning, 118 region, 129 in convolution, 158 sample, 130 binomial convergence in probability, 22 coefficient, 18 ConvNet, see convolutional neural network interval, 147 convolution, 155 probability distribution, 18, 147 convolutional neural network, 194 Poissonian limit, 40 Fourier transform, 156 random process, 17 convolutional neural network, 193 random variable, 18 feature map, 194 BLUE, see best linear unbiased estimator local receptive fields, 194 boosted decision trees, 181 correlation coefficient, 14 adaptive boosting, 198 counting experiment, 208, 212, 216, 227 boosting, 198 Cousins–Highlands method, 227 cross entropy, 196 covariance, 14 decision forest, 197 matrix, 14 Gini index, 196 coverage, 100 leaf, 196 Cramér–Rao bound, 102 node, 196 Cramér–von Mises test, 184 boosting, boosted decision trees, 198 credible interval, 70 Box–Muller transformation, 89 cross entropy, decision tree, 196 Brazil plot, 225 Crystal Ball function, 44 breakdown point, robust estimator, 103 cumulative distribution, 28 Breit–Wigner cut, 176 non-relativistic distribution, 41 relativistic distribution, 42 data sample, 99 decision Cauchy distribution, 41 forest, 197 central tree, 196 interval, 70 deep learning, artificial neural network, 192 limit theorem, 46 degree of belief, 65 value, 99 dices, 4–6, 16, 21 chaotic regime, 82 differential probability, 26 classical probability, 4 discovery, 205, 207, 208 Index 253 distribution, see probability distribution flat (uniform) distribution, 6, 30 dogma, extreme Bayesian prior, 66 flip-flopping, 150 drand48 function from C standard library, 84 forest, boosted decision trees, 197 Fourier transform of PDF convolution, 156 efficiency frequentist hit-or-miss Monte Carlo, 90 inference, 100 of a detector, 10, 158 probability, 3, 22 estimate, 104 full width at half maximum, 31, 41 of an estimator, 102 fully asymmetric interval, 70 elementary event, 4, 6, 9 FWHM, full width at half maximum, equiprobability, 4, 6, 25 31 ergodicity, 94 error of a measurement gamma function, 33, 71 Bayesian approach, 70 Gaussian frequentist approach, 99 average value, ,31 of the first kind, 177 bifurcated, 124 of the second kind, 177 contours in two dimensions, 55 propagation cumulative distribution, 31 Bayesian case, 79 distribution, 31 frequentist case, 121 in more dimensions, 54 simple cases, 121 intervals, 32, 58 estimate, 68, 97, 99, 100 likelihood function, 108 estimator, 100 random number generator, 89 efficiency, 102 central limit theorem, 88 maximum likelihood, 105 standard deviation, ,31 properties, 101 generator, see pseudorandom number generator robust, 103 Gini index, decision tree, 196 Euler characteristic, 246 global significance level, 242 event, 2 goodness of fit, 33, 118, 120 counting experiment, 187, 206 gsl_rng_rand function from GSL library, elementary, 4, 6, 9 84 in physics, 105 in statistics, 2 independent, 10 Hastings ratio, 93 evidence histogram, 119 3 significance level, 207 convolution, 158 Bayes factor, 73 in Asimov dataset, 231 excess, 15 PDF approximation, 182 exclusion, 211 hit-or-miss Monte Carlo, 90 expected value. see average value homogeneous Markov chain, 93 exponential distribution, 34 Hui’s triangle, 18, 23 random number generator, 87 hybrid frequentist approach, 227 extended likelihood function, 106, 186 hypothesis test, 175 fast Fourier transform, 156 IID, independent identically distributed feature map, convolutional neural network, 194 random variables, 82 feedforward multilayer perceptron, 190 IIW, intrinsic information weight, BLUE Feldman–Cousins unified intervals, 152 method, 136 FFT. see fast Fourier transform importance sampling, 91 Fisher information, 75, 102, 136 improper prior distribution, 76 Fisher’s linear discriminant, 178 incomplete Gamma function, 41 254 Index independent linear regression, 115 and identically distributed random local variables, 82, 106 receptive fields, 194 events, 10 significance level, 210, 242 random variables, 50 log normal distribution, 33 inference, 97 logistic map, 82 Bayesian, 68 look elsewhere effect, 210, 242 intersubjective probability, 75 in more dimensions, 246 intrinsic information weight, BLUE method, Lorentz distribution, 41 136 loss function, 191 invariant prior, see Jeffreys’ prior lower limit, 70 iterative unfolding, 166 lrand48 function from C standard library, 84 Jeffreys’ prior, 75 machine learning, 188 joint probability distribution, 49 observation, 189 supervised, 188 kernel function, see response function unsupervised, 188 Kolmogorov distribution, 183 marginal Kolmogorov–Smirnov test, 182 distribution, 49 kurtosis, 15 information weight, BLUE method, 136 coefficient, 15 Markov chain, 93 homogeneous, 93 Monte Carlo, 69, 93 L’Ecuyer pseudorandom number generator, 84 maximum likelihood L-curve, 165 estimator, 105 Lüscher pseudorandom number generator, 84 bias, 113 Landau distribution, 46 properties, 112 large numbers, law of, 21 method, 69, 105 law uncertainty, 109 of large numbers, 21 MC, see Monte Carlo of total probability, 11 MCMC, Markov chain Monte Carlo, 93 leaf, decision tree, 196 median, 14, 28, 103 learning Mersenne-Twistor pseudorandom number process in Bayesian probability, 67 generator, 84 rate parameter, artificial neural network, Metropolis–Hastings 191 algorithm, 93 least squares method, 114 proposal distribution, 93 lifetime, 35, 39 ratio, 95 Bayesian inference, 76 minimum Jeffreys prior, 77 2 method, see 2 method maximum likelihood estimate, 112 variance bound, 102 measurement combination, 140 MINUIT, 106, 110 likelihood misidentification probability, 176 function, 67, 105 MIW, marginal information weight, BLUE extended, 106, 186 method, 136 Gaussian, 108 mode, 14, 28 in Bayesian probability, 67 modified frequentist approach, 221 ratio Monte Carlo method, 6, 46, 69, 81 discriminant, 181 hit-or-miss, 90 in search for new signals, 185, 209 numerical integration, 92 projective discriminant, 182 sampling, 89 test statistic in Neyman–Pearson multilayer perceptron, 190 lemma, 181 multimodal distribution, 14, 28 Index 255 multinomial distribution, 20 pooling, convolutional neural network, 194 multivariate analysis, 178 posterior MVA, multivariate analysis, 178 odds, 64, 73 probability, 60, 65, 67 prior negative weights, 135, 137 odds, 73 nested hypotheses, see Wilks’ theorem probability, 60, 65 neural network, see artificial neural network distribution, 67 Neyman distribution, improper, 76 confidence belt distribution, uniform, 71, 74 binomial case, 147 subjective choice, 74 construction, 144 uninformative, 69, 75 Feldman–Cousins, 152, 218 probability, 2 Gaussian case, 146 axiomatic definition, 8 inversion, 146 Bayesian, 3, 4 confidence intervals, 215 classical, 4 Neyman’s 2, 119 density, 25 Neyman–Pearson lemma, 181 dice rolls, 5 node, decision tree, 196 distribution, 9, 25 normal 2,32 distribution, see Gaussian distribution Bernoulli, 17 random variable, 31 beta, 83 normalization condition, 9, 26 bimodal, 14 nuisance parameter,
Details
-
File Typepdf
-
Upload Time-
-
Content LanguagesEnglish
-
Upload UserAnonymous/Not logged-in
-
File Pages7 Page
-
File Size-