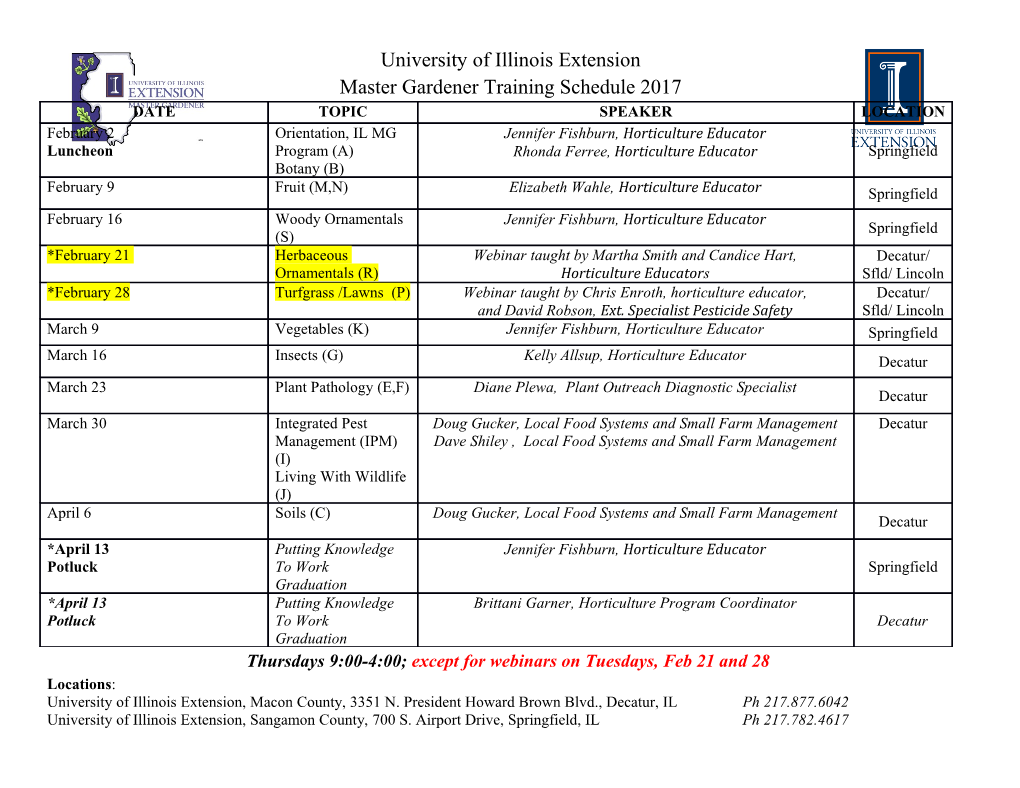
Finding Eigenvalues: Arnoldi Iteration and the QR Algorithm Will Wheeler Algorithms Interest Group Jul 13, 2016 Outline Finding the largest eigenvalue I Largest eigenvalue power method I So much work for one eigenvector. What about others? I More eigenvectors orthogonalize Krylov matrix I build orthogonal list Arnoldi iteration Solving the eigenvalue problem I Eigenvalues diagonal of triangular matrix I Triangular matrix QR algorithm I QR algorithm QR decomposition I Better QR decomposition Hessenberg matrix I Hessenberg matrix Arnoldi algorithm Power Method How do we find the largest eigenvalue of an m × m matrix A? I Start with a vector b and make a power sequence: b; Ab; A2b;::: I Higher eigenvalues dominate: n n n n A (v1 + v2) = λ1v1 + λ2v2 ≈ λ1v1 Vector sequence (normalized) converged? I Yes: Eigenvector I No: Iterate some more Krylov Matrix Power method throws away information along the way. I Put the sequence into a matrix K = [b; Ab; A2b;:::; An−1b] (1) = [xn; xn−1; xn−2;:::; x1] (2) I Gram-Schmidt approximates first n eigenvectors v1 = x1 (3) x2 · x1 v2 = x2 − x1 (4) x1 · x1 x3 · x1 x3 · x2 v3 = x3 − x1 − x2 (5) x1 · x1 x2 · x2 ::: (6) I Why not orthogonalize as we build the list? Arnoldi Iteration I Start with a normalized vector q1 xk = Aqk−1 (next power) (7) k−1 X yk = xk − (qj · xk ) qj (Gram-Schmidt) (8) j=1 qk = yk = jyk j (normalize) (9) I Orthonormal vectors fq1;:::; qng span Krylov subspace n−1 (Kn = spanfq1; Aq1;:::; A q1g) I These are not the eigenvectors of A, but make a similarity y (unitary) transformation Hn = QnAQn Arnoldi Iteration I Start with a normalized vector q1 xk = Aqk−1 (next power) (10) k−1 X yk = xk − (qj · xk ) qj (Gram-Schmidt) (11) j=1 qk = yk =jyk j (normalize) (12) I Orthonormal vectors fq1;:::; qng span Krylov subspace n−1 (Kn = spanfq1; Aq1;:::; A q1g) I These are not the eigenvectors of A, but make a similarity y (unitary) transformation Hn = QnAQn Arnoldi Iteration - Construct Hn We can construct the matrix Hn along the way. y I For k ≥ j: hj;k−1 = qj · xk = qj Aqk−1 I For k = j: hj;k−1 = jyk j (equivalent) I For k < j: hj;k−1 = 0 I ) Hn is upper Hessenberg 0 1 h1;1 h1;2 h1;3 h1;4 h1;5 h1;6 Bh2;1 h2;2 h2;3 h2;4 h2;5 h2;6C B C B 0 h3;2 h3;3 h3;4 h3;5 h3;6C Hn = B C (13) B 0 0 h4;3 h4;4 h4;5 h4;6C B C @ 0 0 0 h5;4 h5;5 h5;6A 0 0 0 0 h6;5 h6;6 y I Why is this useful? Hn = QnAQn ! same eigenvalues as A Finding Eigenvalues How do we find eigenvalues of a matrix? I Start with a triangular matrix A I The diagonal elements are the eigenvalues 0 1 a11 a12 a13 a14 a15 a16 B 0 a22 a23 a24 a25 a26C B C B 0 0 a33 a34 a35 a36C A = B C (14) B 0 0 0 a44 a45 a46C B C @ 0 0 0 0 a55 a56A 0 0 0 0 0 a66 What if A isn't triangular? QR algorithm Factorize A = QR I Q is orthonormal (sorry this is a different Q) I R is upper triangular (aka right triangular) Algorithm: 1. Start A1 = A 2. Factorize Ak = Qk Rk 3. Construct Ak+1 = Rk Qk −1 −1 = Qk Qk Rk Qk = Qk Ak Qk similarity transform 4. Ak converges to upper triangular QR Algorithm Example (numpy.linalg.qr) 00:313 0:106 0:8991 A1 = @0:381 0:979 0:375A 0:399 0:488 0:876 0 1:649 0:05 −0:2561 A2 = @ 0:035 0:502 0:534 A −0:014 0:004 0:017 01:653e + 00 2:290e − 02 2:305e − 01 1 A3 = @5:966e − 03 5:063e − 01 −5:342e − 01A 8:325e − 05 −9:429e − 05 9:666e − 03 0 1:653e + 00 1:872e − 02 −2:285e − 011 A4 = @ 1:800e − 03 5:063e − 01 5:349e − 01 A −4:812e − 07 1:803e − 06 9:554e − 03 QR Decomposition - Gram-Schmidt How do we get the matrices Q and R? http://www.seas.ucla.edu/ vandenbe/103/lectures/qr.pdf Recursively solve for the first column of Q and the first row of R: I A = QR r11 R12 I a1 A2 = q1 Q2 0 R22 I a1 A2 = r11q1 q1R12 + Q2R22 I r11 = jja1jj, q1 = a1=r11 | | I Note q1q1 = 1 and q1Q2 = 0 | I R12 = q1A2 I Solve A2 − q1R12 = Q2R22 QR Decomposition of Hessenberg Matrix What if we have a matrix in upper Hessenberg form? 0 1 h1;1 h1;2 h1;3 h1;4 h1;5 h1;6 Bh2;1 h2;2 h2;3 h2;4 h2;5 h2;6C B C B 0 h3;2 h3;3 h3;4 h3;5 h3;6C Hn = B C (15) B 0 0 h4;3 h4;4 h4;5 h4;6C B C @ 0 0 0 h5;4 h5;5 h5;6A 0 0 0 0 h6;5 h6;6 We just need to remove the numbers below the diagonal by combining rows ! Givens rotation. Givens Rotation A Givens rotation acts only on two rows and leaves the others unchanged. 0γ −σ 0 0 01 Bσ γ 0 0 0C B C G1 = B0 0 1 0 0C (16) B C @0 0 0 1 0A 0 0 0 0 1 a r p Want G = ; r = a2 + b2 b 0 p 2 2 I γ = a= a + b p 2 2 I σ = −b= a + b QR Decomposition of Hessenberg Matrix 0 1 he11 he12 he13 he14 he15 B C B 0 he22 he23 he24 he25C B C G2G1Hn = B 00 he33 he34 he35C (17) B C @ 0 0 h43 h44 h45A 0 0 0 h54 h55 | | | So Hn = G1G2 ::: Gn−1R = QR QR Decomposition of Hessenberg Matrix QR algorithm is iterative; is RQ also upper Hessenberg? Yes: Acting on the right, the Givens rotations mix two columns instead of rows, but change the same zeros. 0 1 re11 re12 re13 r14 r15 Br21 r22 r23 r24 r25C Be e e C G2G1Hn = B 0 r32 r33 r34 r35C (18) B e e C @ 0 00 r44 r45A 0 0 0 0 r55 Householder Reflections I Arnoldi finds a few eigenvalues of a large matrix I In practice, QR uses Householder reflections I www.math.usm.edu/lambers/mat610/sum10/lecture9.pdf Reflection across the plane perpendicular to a unit vector v: P = I − 2vv| (19) Px = x − 2vv|x (20) Px − x = −v(2v|x) (21) Want to arrange first column into zeros: find v so that Px = αe1. Householder Reflections Want to arrange first column into zeros: find v so that Px = αe1. jjxjj = α (22) | x − 2vv x = αe1 (23) 1 (x − αe ) = v(v|x) (24) 2 1 So we know I v / x − αe1 I v is a unit vector x − αe v = 1 (25) jjx − αe1jj Lanczos What if we apply the Arnoldi algorithm to a Hermitian matrix? I Start with a normalized vector q1 xk = Aqk−1 (next power) (26) k−1 X yk = xk − (qj · xk ) qj (Gram-Schmidt) (27) j=k−2 qk = yk = jyk j (normalize) (28) y Since Hn = QnAQn, I Hn is also Hermitian I Entries of Hn above the first diagonal are 0 (tridiagonal) I We don't need to calculate them! Lanczos What if we apply the Arnoldi algorithm to a Hermitian matrix? y Since Hn = QnAQn, I Hn is also Hermitian I Entries of Hn above the first diagonal are 0 (tridiagonal) I We don't need to calculate them! 0 ∗ 1 h11 h21 0 0 0 ∗ Bh21 h22 h32 0 0 C B ∗ C Hn = B 0 h32 h33 h43 0 C (29) B ∗ C @ 0 0 h43 h44 h54 A 0 0 0 h54 h55.
Details
-
File Typepdf
-
Upload Time-
-
Content LanguagesEnglish
-
Upload UserAnonymous/Not logged-in
-
File Pages19 Page
-
File Size-