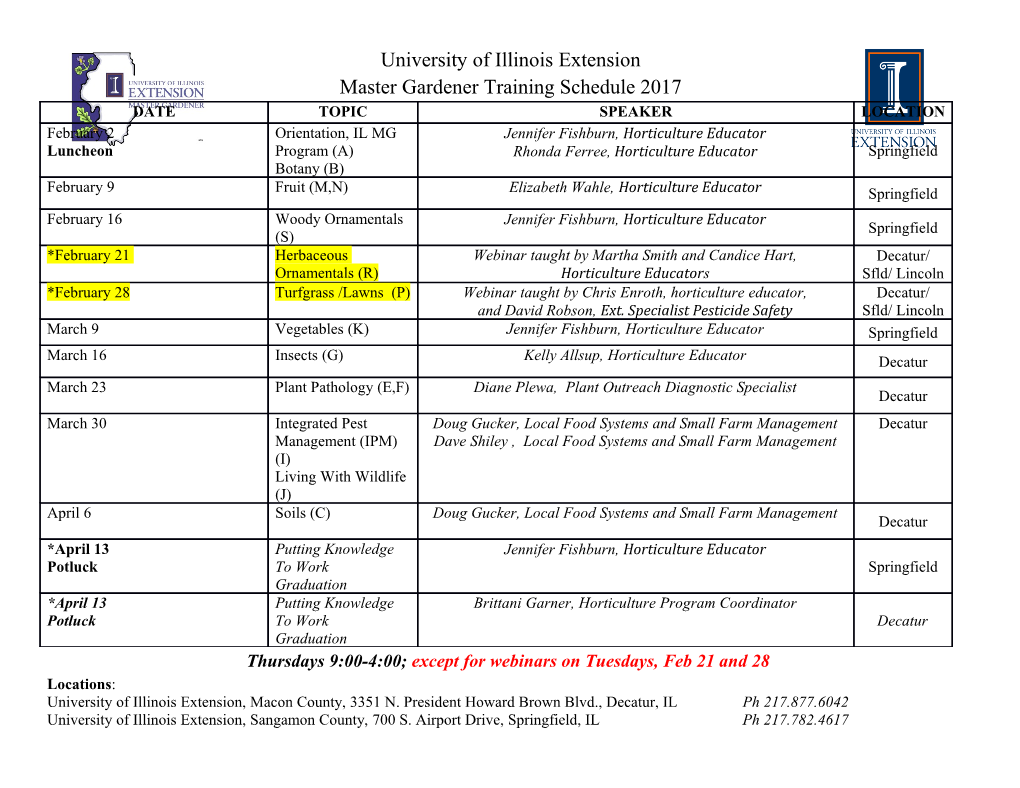
(PHUJLQJ5HVHDUFKLQWKH $QDO\VLVDQG0RGHOLQJ RI*HQH5HJXODWRU\ 1HWZRUNV ,YDQ9,YDQRY 7H[DV$ 08QLYHUVLW\86$ ;LDRQLQJ4LDQ 7H[DV$ 08QLYHUVLW\86$ 5DQDGLS3DO 7H[DV7HFK8QLYHUVLW\86$ $YROXPHLQWKH$GYDQFHVLQ 0HGLFDO7HFKQRORJLHVDQG &OLQLFDO3UDFWLFH $07&3 %RRN6HULHV Published in the United States of America by Medical Information Science Reference (an imprint of IGI Global) 701 E. Chocolate Avenue Hershey PA 17033 Tel: 717-533-8845 Fax: 717-533-8661 E-mail: [email protected] Web site: http://www.igi-global.com Copyright © 2016 by IGI Global. All rights reserved. No part of this publication may be reproduced, stored or distributed in any form or by any means, electronic or mechanical, including photocopying, without written permission from the publisher. Product or company names used in this set are for identification purposes only. Inclusion of the names of the products or companies does not indicate a claim of ownership by IGI Global of the trademark or registered trademark. Library of Congress Cataloging-in-Publication Data Names: Ivanov, Ivan V., 1962- | Qian, Xiaoning, 1975- | Pal, Ranadip, 1980- Title: Emerging research in the analysis and modeling of gene regulatory networks / Ivan V. Ivanov, Xiaoning Qian, and Ranadip Pal, editors. Description: Hershey, PA : Medical Information Science Reference, [2016] | Includes bibliographical references and index. Identifiers: LCCN 2016005966| ISBN 9781522503538 (hardcover) | ISBN 9781522503545 (ebook) Subjects: LCSH: Genetic regulation--Computer simulation. | Gene regulatory networks. Classification: LCC QH450 .E44 2016 | DDC 572.8/65--dc23 LC record available at https://lccn. loc.gov/2016005966 This book is published in the IGI Global book series Advances in Medical Technologies and Clinical Practice (AMTCP) (ISSN: 2327-9354; eISSN: 2327-9370) British Cataloguing in Publication Data A Cataloguing in Publication record for this book is available from the British Library. All work contributed to this book is new, previously-unpublished material. The views expressed in this book are those of the authors, but not necessarily of the publisher. &KDSWHU ,QIHUHQFHRI*HQH 5HJXODWRU\1HWZRUNV E\7RSRORJLFDO3ULRU ,QIRUPDWLRQDQG 'DWD,QWHJUDWLRQ David Correa Martins Jr. Federal University of ABC (UFABC), Brazil Fabricio Martins Lopes Federal University of Technology – Paraná (UTFPR), Brazil Shubhra Sankar Ray Indian Statistical Institute, India $%675$&7 The inference of Gene Regulatory Networks (GRNs) is a very challenging problem which has attracted increasing attention since the development of high-throughput sequencing and gene expression measurement technologies. Many models and algo- rithms have been developed to identify GRNs using mainly gene expression profile as data source. As the gene expression data usually has limited number of samples and inherent noise, the integration of gene expression with several other sources of information can be vital for accurately inferring GRNs. For instance, some prior information about the overall topological structure of the GRN can guide inference DOI: 10.4018/978-1-5225-0353-8.ch001 Copyright ©2016, IGI Global. Copying or distributing in print or electronic forms without written permission of IGI Global is prohibited. ,QIHUHQFHRI*HQH5HJXODWRU\1HWZRUNVE\7RSRORJLFDO3ULRU,QIRUPDWLRQ techniques toward better results. In addition to gene expression data, recently bio- logical information from heterogeneous data sources have been integrated by GRN inference methods as well. The objective of this chapter is to present an overview of GRN inference models and techniques with focus on incorporation of prior in- formation such as, global and local topological features and integration of several heterogeneous data sources. ,1752'8&7,21 Systems Biology is an interdisciplinary research field that aims at the study of complex interactions occurring in living organisms (Snoep & Westerhoff, 2005). Research in this field focuses on the study of biological processes such as cell cycles and the conditions for the origin of certain diseases. The ultimate goal of these stud- ies is to help the development of new treatments and drugs against diseases, biofuel production techniques, among many other applications. The genome of an organism has a central role in the control of cell processes such as cell response to environmental stimuli, cell differentiation in its respective functional groups, DNA replication for cell division, and many others. An organism can be seen as a network of molecules connected by biochemical reactions (Voet, Voet & Pratt, 2005). Proteins synthesized from genes may work as transcription fac- tors which bind to regulatory sites of other genes, such as enzymes which catalyze metabolic reactions or components of signal transduction pathways. Such regulatory mechanism forms a complex system of sending and receiving signals (RNAs) which can be investigated to identify the control mechanisms of the cell and the relationships among various biological entities like genes, RNAs and proteins. However, there is still much to be discovered about the functional relationships of control mechanisms, e.g., transcription levels and proteins, in the regulatory system (Barabasi,2002; Fall, Marland, Wagner & Tyson, 2002; Shmulevich & Dougherty, 2007). With few exceptions, all cells of an organism contain the same genetic mate- rial, although cells of different tissues are functionally different. The cell function is partially determined and controlled by gene expression profiles. With the aim of understanding how genes are involved in control of intra and inter cell processes, the scope of the molecular biology studies needs to be enlarged to include not only the discovery of nucleotide sequences that codes for proteins, but also the unravel- ing of the regulatory systems which determine what genes are expressed, when, where, and to how much extent (Snoep & Westerhoff, 2005). The explanation of these regulatory networks functioning, by means of sending and receiving signals, is currently one of the main objectives of the systems biology studies. ,QIHUHQFHRI*HQH5HJXODWRU\1HWZRUNVE\7RSRORJLFDO3ULRU,QIRUPDWLRQ One of the most challenging research problems of Systems Biology is the infer- ence (or reverse-engineering) of gene regulatory networks (GRNs) from expression profiles (Werhli, Grzegorczyk & Husmeier, 2006; Marbach et al, 2012). This research issue became important after the development of high-throughput technologies for extraction of gene expressions such as DNA microarrays (Schena, Shalon, Davis & Brown, 1995) or SAGE (Velculescu, Zhang, Vogelstein, & Kinzler, 1995), and more recently RNA-Seq (Wang, Gerstein, & Snyder, 2009). The importance of GRN reconstruction can be seen through initiatives taken for this purpose such as DREAM (Dialogue for Reverse Engineering Assessments and Methods) (Marbach et al, 2012). The inference problem involves discovery of complex regulatory relationships among biological molecules which can describe not only diverse biological functions, but also the dynamics of molecular activities. Once the network is recovered, intervention studies can be conducted to control the dynamics of the biological systems aiming to prevent or treat diseases (Shmulevich & Dougherty, 2007). In general, it is not possible to recover GRNs very accurately based only on gene expression profiles for several reasons, including the presence of significant noise in the data, limited number of samples and large dimensionality. Also, GRN infer- ence is considered an ill-posed problem, meaning that many networks may be able to explain the data in hand. Besides, the lack of information about the biological organism and the high complexity of the networks are additional challenges involved in GRN inference. From the computational point of view, this problem is NP-hard, requiring the development of approximation algorithms and high performance computing (including parallelization) techniques. To infer, analyze and compare the interrelationship between genes with adequate precision is an open research problem. In this regard, the integration of mathemati- cal models with several types of molecular information can be crucial for GRN inference and discovery of biological knowledge as well as their characterization (synthesis) (Ray, Bandyopadhyay, & Pal, 2009; Hecker, Lambeck, Toepfer, van Someren, & Guthke, 2009; Ristevski, 2013; Lopes, Ray, Hashimoto, & Cesar Jr., 2014b). Some widely studied available sources of biological data are Gene Ontol- ogy (GO) (Ashburner et al, 2000), GenBank (Benson, Karsch Mizrachi, Lipman, Ostell, & Wheeler, 2008), KEGG (Kyoto Encyclopedia of Genes and Genomes) (Kanehisa, Goto, Furumichi, Tanabe, & Hirakawa, 2010), The Arabidopsis Informa- tion Resource (TAIR) (D’Angelo, Kilian, Kudla, Batistic, & Weinl, 2009), Munich Information Center for Protein Sequences (MIPS) (Mewes, et al, 2011), Protein- Protein Interaction databases (Prasad et al, 2009; Licata et al, 2012; Orchard et al, 2014), to cite but a few. Another important trend is the inclusion of global and local topological information to improve GRN inference methods (Hecker et al, 2009; Lopes, Martins Jr, Barrera, Cesar Jr, 2014a). ,QIHUHQFHRI*HQH5HJXODWRU\1HWZRUNVE\7RSRORJLFDO3ULRU,QIRUPDWLRQ The objective of this chapter is to present an overview of GRN inference tech- niques with focus on incorporation of prior information such as global and local topological features and integration of several heterogeneous data sources. The chapter will cover modeling, inference, validation and computational
Details
-
File Typepdf
-
Upload Time-
-
Content LanguagesEnglish
-
Upload UserAnonymous/Not logged-in
-
File Pages53 Page
-
File Size-