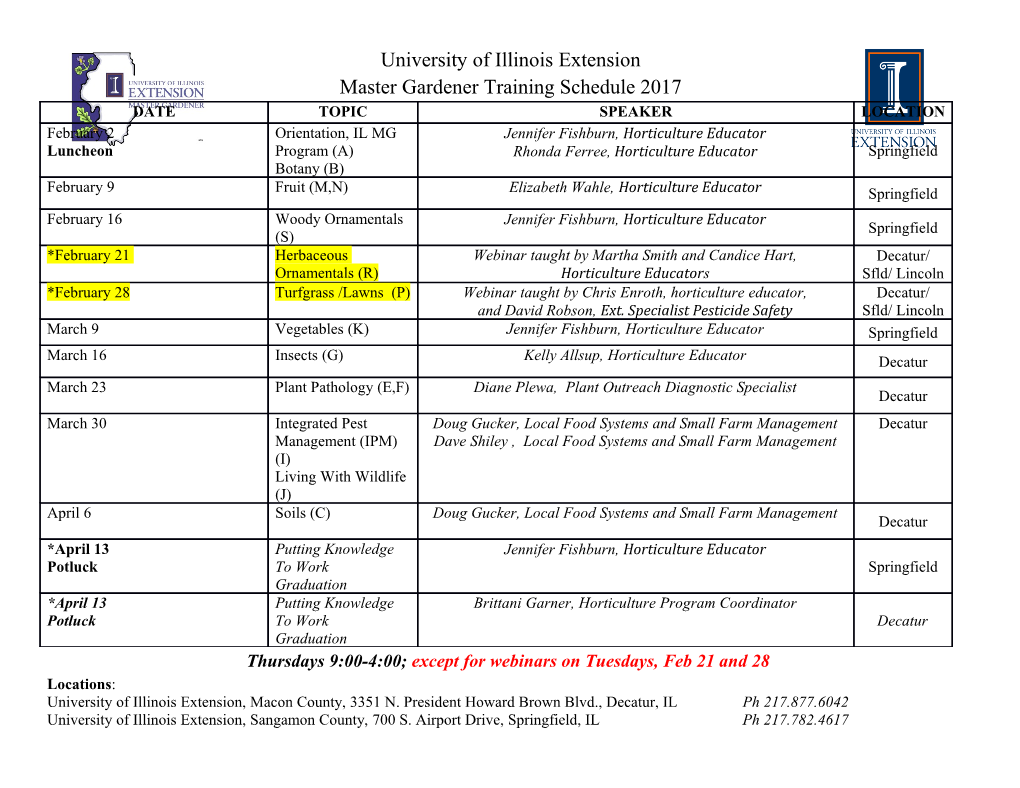
TD-GAMMON REVISITED: INTEGRATING INVALID ACTIONS AND DICE FACTOR IN CONTINUOUS ACTION AND OBSERVATION SPACE A THESIS SUBMITTED TO THE GRADUATE SCHOOL OF NATURAL AND APPLIED SCIENCES OF MIDDLE EAST TECHNICAL UNIVERSITY BY ENGIN˙ DENIZ˙ USTA IN PARTIAL FULFILLMENT OF THE REQUIREMENTS FOR THE DEGREE OF MASTER OF SCIENCE IN COMPUTER ENGINEERING SEPTEMBER 2018 Approval of the thesis: TD-GAMMON REVISITED: INTEGRATING INVALID ACTIONS AND DICE FACTOR IN CONTINUOUS ACTION AND OBSERVATION SPACE submitted by ENGIN˙ DENIZ˙ USTA in partial fulfillment of the requirements for the degree of Master of Science in Computer Engineering Department, Middle East Technical University by, Prof. Dr. Halil Kalıpçılar Dean, Graduate School of Natural and Applied Sciences Prof. Dr. Halit Oguztüzün˘ Head of Department, Computer Engineering Prof. Dr. Ferda Nur Alpaslan Supervisor, Computer Engineering, METU Examining Committee Members: Prof. Dr. Pınar Karagöz Computer Engineering, METU Prof. Dr. Ferda Nur Alpaslan Computer Engineering, METU Assist. Prof. Dr. Kasım Öztoprak Computer Engineering, Karatay University Date: I hereby declare that all information in this document has been obtained and presented in accordance with academic rules and ethical conduct. I also declare that, as required by these rules and conduct, I have fully cited and referenced all material and results that are not original to this work. Name, Last Name: Engin Deniz Usta Signature : iv ABSTRACT TD-GAMMON REVISITED: INTEGRATING INVALID ACTIONS AND DICE FACTOR IN CONTINUOUS ACTION AND OBSERVATION SPACE Usta, Engin Deniz M.S., Department of Computer Engineering Supervisor : Prof. Dr. Ferda Nur Alpaslan September 2018, 39 pages After TD-Gammon’s success in 1991, the interest in game-playing agents has risen significantly. With the developments in Deep Learning and emulations for older games have been created, human-level control for Atari games has been achieved and Deep Reinforcement Learning has proven itself to be a success. However, the ancestor of DRL, TD-Gammon, and its game Backgammon got out of sight, because of the fact that Backgammon’s actions are much more complex than other games (most of the Atari games has 2 or 4 different actions), the huge action space has much invalid actions, and there is a dice factor which involves stochasticity. Last but not least, the professional level in Backgammon has been achieved a long time ago. In this thesis, the latest methods in DRL will be tested against its ancestor game, Backgammon, while trying to teach how to select valid moves and considering the dice factor. Keywords: TDGammon, Deep Deterministic Policy Gradients, OpenAI-GYM, GYM- v Backgammon, Continuous Action Space vi ÖZ TD-GAMMON’A YENIDEN˙ BAKI¸S:TAVLA’DA SÜREKLI˙ AKSIYON˙ VE GÖZLEM ALANI IÇ˙ INE˙ GEÇERSIZ˙ HAMLELERI˙ VE ZAR FAKTÖRÜNÜ DAHIL˙ ETMEK Usta, Engin Deniz Yüksek Lisans, Bilgisayar Mühendisligi˘ Bölümü Tez Yöneticisi : Prof. Dr. Ferda Nur Alpaslan Eylül 2018 , 39 sayfa TD-Gammon’un 1991’deki ba¸sarısından sonra, oyun oynayabilen etmenlere olan ilgi bir hayli artmı¸sdurumda. Derin Ögrenme˘ ve eski oyunların emülatorlerindeki geli¸smelerden sonra, Atari oyunları için insan seviyesinde oynayabilen etmenler ortaya çıktı, ve Derin Takviyeli Ögrenme˘ kendi ba¸sarısınıkanıtladı. Ancak, Derin Takviyeli Ögrenme’nin˘ atası olan TD-Gammon, ve oyunu Tavla, arka planda kaldı. Bunun sebepleri ise, Tavla’nın aksiyonlarının diger˘ Atari oyunlarına gore çok daha kompleks olması (genelde çogu˘ Atari oyununda 2 veya 4 farklı aksiyon alınabilir), aksiyon alanında çok fazla geçersiz aksiyon olması, ve zar faktörünün getirdigi˘ rastgelelik olarak gorülüyor. Son sebep olarak ise, Tavla’da uzun sure once profesyonel seviyede oynayabilen etmenlerin varlıgı˘ oldugunu˘ söyleyebiliriz. Bu tezde, son çıkan Derin Takviyeli Ögrenme˘ yöntemleri onların atası olan oyuna, Tavla’ya kar¸sı test edilecektir. Bu sırada ek olarak, etmenlerimiz zar faktörünü de hesaba katarak geçerli hamleleri bulmaya çalı¸sacaktır. vii Anahtar Kelimeler: TD-Tavla, Derin Deterministik Poliçe Egimi,˘ OpenAI-GYM, GYM- Tavla, Sürekli Aksiyon Alanı viii To my dearest family and my lovely Müge... ix ACKNOWLEDGMENTS First of all, I would like to thank my supervisor, Prof. Dr. Ferda Nur Alpaslan who always supported and kept my motivation high. Her extensive knowledge and intuitions greatly helped me through hardest times. It has been a pleasure being guided by her, and I hope I will have a chance to work with her again. I would also like to thank Özgür Alan for his extensive data and motivation support. Last but not least, I would like to thank Ömer Baykal for providing his knowledge about his prior experiences on NeuroGammon. This work is supported and funded by the Scientific and Technological Research Council of Turkey (TUBITAK) under Grant No. 2228-A, 1059B281500849. x TABLE OF CONTENTS .ABSTRACT . .v .ÖZ......................................... vii .ACKNOWLEDGMENTS . .x .TABLE OF CONTENTS . xi .LISTOF TABLES . xiv .LISTOF FIGURES . xv .LISTOF ABBREVIATIONS . xvii . CHAPTERS 1. INTRODUCTION . .1 1.1. Reinforcement Learning in Continuous State and Action Spaces1 1.2. Revisiting TD-Gammon: Factoring Invalid Actions and Dice2 1.3. Contributions . .2 1.4. Outline . .3 2. BACKGROUND AND RELATED WORK . .5 2.1. Reinforcement Learning . .5 2.1.1. Temporal Difference . .5 2.1.2. Q-Learning . .6 2.1.3. Deep Q Network (DQN) . .6 xi 2.1.4. Deep Deterministic Policy Gradients (DDPG) . .6 2.1.5. Continuous Control with Deep Reinforcement Learning7 2.1.5.1. Policy-Gradient Method . .7 2.1.5.2. Actor-Critic Concept . .7 2.1.5.3. Batch Normalization . .8 2.1.5.4. Algorithm . .9 2.2. TD-Gammon: The Ancestor of Deep Reinforcement Learning 10 2.2.1. Information and Background Info . 10 2.2.2. Continuous Action and Observation Space In Backgammon 10 2.2.2.1. Mapping Discrete Action Space to Continuous Action Space . 11 2.2.2.2. Continous Observation Space . 12 2.2.3. Diversity of Actions and Dice Factor . 13 2.3. OpenAI-GYM . 15 2.4. Related Work . 16 2.4.1. Deep Reinforcement Learning Compared with Q- Table Learning Applied to Backgammon . 16 3. DDPG-GAMMON . 19 3.1. Preparing Backgammon For Networks . 19 3.2. GYM-Backgammon . 20 3.2.1. GYM-Backgammon-Discretized . 20 3.2.1.1. Test Of Concept . 21 3.2.2. GYM-Backgammon-Continuous . 22 3.2.2.1. Test Of Concept . 23 xii 3.3. Experiments . 24 3.3.1. Network Layouts . 24 3.3.2. Setups . 25 3.3.2.1. Random Seed . 25 3.3.2.2. Custom Reward and Action System (CRAS) . 26 3.3.2.3. Configurations . 27 3.4. Results . 27 3.4.1. Setup 1 . 27 3.4.2. Setup 2 . 28 3.4.3. Setup 3 . 28 3.4.4. Setup 4 . 29 3.4.5. Setup 5 . 30 3.4.6. Setup 6 . 30 3.4.7. Setup 7 . 31 3.4.8. Setup 8 . 31 3.4.9. Summary . 33 4. CONCLUSION AND FUTURE WORK . 35 4.1. Conclusion . 35 4.2. Future Work . 36 .REFERENCES . 37 xiii LIST OF TABLES . TABLES xiv LIST OF FIGURES . FIGURES Figure 2.1. The summary of Actor-Critic Methodology. [7] . .8 Figure 2.2. An example GUI from Backgammon [5] . 12 Figure 2.3. Initial observation space from Backgammon. 13 Figure 2.4. New observation space after one turn. 14 Figure 2.5. Example OpenAI-GYM Environment Visuals . 16 Figure 3.1. Backgammon Board Notation [10] . 19 Figure 3.2. Test Of Discretized Backgammon . 21 Figure 3.3. Test of Continuous Backgammon . 23 Figure 3.4. Network Layout [16] . 25 Figure 3.5. Networks without CRAS . 26 Figure 3.6. Results of Setup 1 . 27 Figure 3.7. Results of Setup 2 . 28 Figure 3.8. Results of Setup 3 . 29 Figure 3.9. Results of Setup 4 . 29 Figure 3.10.Results of Setup 5 . 30 Figure 3.11.Results of Setup 6 . 31 xv Figure 3.12.Results of Setup 7 . 32 Figure 3.13.Results of Setup 8 . 32 xvi LIST OF ABBREVIATIONS RL Reinforcement Learning TD Temporal Difference TDGammon Temporal Difference Backgammon DRL Deep Reinforcement Learning DQN Deep Q-Network DDPG Deep Deterministic Policy Gradients DDPG-Gammon Deep Deterministic Policy Gradients - Backgammon Agent RNG Random Number Generator CRAS Custom Reward and Action System xvii xviii CHAPTER 1 INTRODUCTION 1.1 Reinforcement Learning in Continuous State and Action Spaces Previous reinforcement-learning algorithms are created for small discrete action and observation spaces. On the other hand, most of the real-world problems have continuous action and/or observation spaces, which requires to find good decision policies within more complex situations [28]. Although Q-Learning is proven to be able to integrate with continuous action/observation spaces, Actor-Critic learning has an edge over Continuous-Q-Learning [4], because of its ability to respond fast-changing observations with fast-changing actions. In order to tackle with continuous action/observation spaces, there are many algorithms proposed, such as Continuous Actor-Critic Learning Automaton [29], Gradient Temporal Difference Networks [18], Trust Region Policy Optimization [17], Deep Deterministic Policy Gradients [8]. Among all these methods, this thesis will focus on Deep Deterministic Policy Gradients (DDPG) [8], which is constructed upon Policy-Gradient and Actor-Critic algorithms. 1 1.2 Revisiting TD-Gammon: Factoring Invalid Actions and Dice Although TD-Gammon’s success is proven in 1992 World Cup Backgammon tournament [24], there are still room for improvement in TD-Gammon. Since TD-Gammon does not require any information regarding valid/invalid actions [25], we may ask the question: "Can an agent learn which action is valid/invalid in Backgammon?". In order to be able to integrate every move in Backgammon, the dice factor must be integrated into the game knowledge, too. Without any filtering of information, the proposed agent, DDPG-Gammon, will be trained to separate the valid moves from invalid moves. 1.3 Contributions This thesis proposes and focuses on a major novelty: GYM-Backgammon, suited from its ancestor, TD-Gammon.
Details
-
File Typepdf
-
Upload Time-
-
Content LanguagesEnglish
-
Upload UserAnonymous/Not logged-in
-
File Pages57 Page
-
File Size-