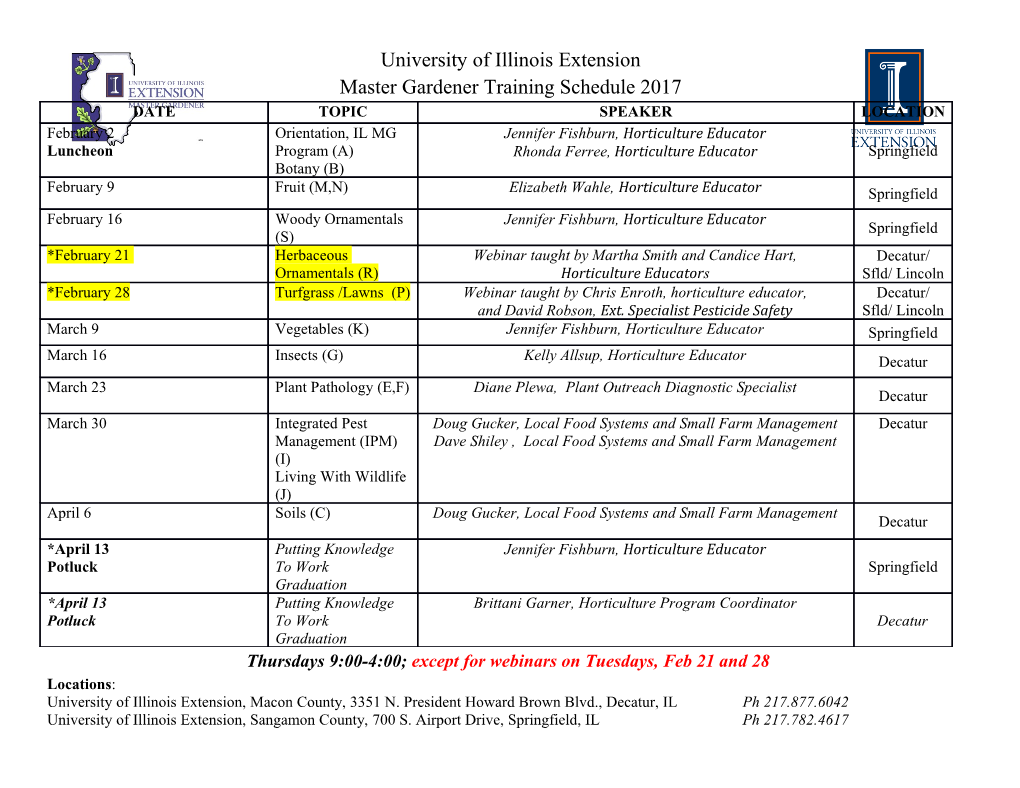
1 Deep Learning for Road Traffic Forecasting: Does it Make a Difference? Eric L. Manibardo, Ibai Lana,˜ and Javier Del Ser, Senior Member, IEEE Abstract—Deep Learning methods have been proven to be average speed, occupancy, travel time, etc.), can be used to flexible to model complex phenomena. This has also been the enhance traffic management and implement operational mea- case of Intelligent Transportation Systems (ITS), in which several sures to relieve or prevent traffic congestion and its consequent areas such as vehicular perception and traffic analysis have widely embraced Deep Learning as a core modeling technology. implications [6], [7]. Motivated by this problem, a plethora of Particularly in short-term traffic forecasting, the capability of short-term traffic forecasting works is published every year, as Deep Learning to deliver good results has generated a prevalent can be seen in recent surveys on this topic [8], [9], [10]. inertia towards using Deep Learning models, without examining Although there are plenty of data-driven methods that can in depth their benefits and downsides. This paper focuses on deliver a short-term traffic prediction model, Deep Learning critically analyzing the state of the art in what refers to the use of Deep Learning for this particular ITS research area. To methods have monopolized the majority of publications of this end, we elaborate on the findings distilled from a review of this type in recent years, becoming the reference for the publications from recent years, based on two taxonomic criteria. community when facing new forecasting problems [11], [12]. A posterior critical analysis is held to formulate questions and This apogee of the application of Deep Learning methods trigger a necessary debate about the issues of Deep Learning for to ITS problems is commonly justified by its theoretical traffic forecasting. The study is completed with a benchmark of diverse short-term traffic forecasting methods over traffic capability to approximate any non-linear function [13], which datasets of different nature, aimed to cover a wide spectrum is often the case of patterns underneath traffic time series [14]. of possible scenarios. Our experimentation reveals that Deep In general, short-term prediction models estimate future time Learning could not be the best modeling technique for every case, series values based on recent measurements, whereas long- which unveils some caveats unconsidered to date that should be term traffic forecasting solutions rather focuses on finding addressed by the community in prospective studies. These insights reveal new challenges and research opportunities in road traffic typical traffic profiles. However, Deep Learning models have forecasting, which are enumerated and discussed thoroughly, with their own drawbacks in the form of an inability to understand the intention of inspiring and guiding future research efforts in their behavior [15], [16], and the need for large quantities of this field. data and specialized hardware resources. Index Terms—Machine Learning, Deep Learning, short-term Under this premise, this work elaborates on Deep Learning traffic forecasting, data-driven traffic modeling, spatio-temporal for short-term traffic forecasting in order to ascertain the areas data mining. in which its implementation brings the best outcomes, as well as other scenarios where less computational expensive data- I. INTRODUCTION driven methods provide similar or superior performance. To shed light on this matter, we first analyze thoroughly recent It is undeniable that the boom of the Big Data era has literature on traffic forecasting, specifically those works that revolutionized most research fields [1]. The reason for this propose Deep Learning based solutions. Parting from this prior advent is that much more data are collected from a variety of analysis of the state of the art, we enumerate and discuss a sources, which must be processed and converted into various series of insights, good and poor practices followed by the forms of knowledge for different stakeholders. Intelligent arXiv:2012.02260v1 [cs.LG] 2 Dec 2020 community to date. Our critical analysis is supported by the Transportation Systems (ITS), which aim to improve efficiency results yielded by an experimental study comprising several and security of transportation networks, embody one of the shallow and Deep Learning models and traffic forecasting domains that has largely taken advantage of the availability of datasets. Finally, we outline several research niches, open chal- data generated by different processes and agents that interact lenges, and valuable research directions for the community, in with transportation. Some examples of ITS applications and close connection to the overall conclusions drawn from our use cases that benefit from data availability are railway passen- study on the current status of the field. In summary, the main ger train delay prediction [2], airport gate assignment problem contributions of this paper can be described as follows: [3], adaptive control of traffic signaling in urban areas [4] and improvements of autonomous driving [5], to mention a few. • We categorize recent Deep Learning based short-term traffic Within the diversity of ITS sub-domains, this work is forecasting publications under a taxonomy that considers focused on traffic state forecasting. An accurate traffic state two criteria: 1) the specific forecasting problem; and 2) prediction, based on measurements of different nature (e.g. the selected techniques and methods to model the actual phenomena. Eric L. Manibardo, Ibai Lana˜ and Javier Del Ser are with TECNALIA, • We critically examine the state of the art by following the Basque Research and Technology Alliance (BRTA), 48160 Derio, Bizkaia, above criteria, which allow us to detect research trends and Spain. Javier Del Ser is also with the University of the Basque Country (UPV/EHU) 48013 Bilbao, Bizkaia, Spain to extract insights about overlooked issues and pitfalls. e-mail: [eric.lopez, ibai.lana, javier.delser]@tecnalia.com • We design an extensive experimentation comprising traffic 2 data of different characteristics (e.g. highways and urban Deep Learning models, like others belonging to different arterials) captured from several locations, covering the most subsets of Machine Learning, can perform many tasks such as common scopes of traffic forecasting, intending to show unsupervised learning, classification, or regression. But what good practices when evaluating the performance of novel makes them particularly relevant is their unique capabilities traffic forecasting techniques. to automatically learn hierarchical features from data that are • We enumerate a series of learned lessons drawn from the useful for the task under consideration. Classical Machine experimental setup, underscoring poor research habits that Learning methods are also called flat or shallow learning should be avoided for the sake of valuable advances in the methods because they cannot learn data representations di- field. rectly from unprocessed data. Feature extraction needs to • Finally, we discuss challenges and research opportunities be applied beforehand, often assisted by expert knowledge of the field, all directed towards achieving actionable and about the domain of the problem. Deep Learning methods, trustworthy short-term traffic forecasting models. however, can learn an implicit representation of raw data for The rest of the paper is organized as follows: Section a better understanding of the process to be modeled. This II provides an introduction to the evolution of short-term capability has been proven to go beyond human reasoning traffic forecasting in recent years, Deep Learning concepts, and limits. As a result, for many fields dealing with complex, how this technology has become the spearhead of the traffic highly dimensional data, features discovered by Deep Learning prediction field. Section III defines the proposed taxonomic methods lead to unprecedented performance with respect to the criteria, classifies and reviews the recent state of the art. state of the art. A discussion on the findings and conclusions drawn by the The other main capability of Deep Learning methods stems previous review is held in Section IV. Next, a case study is from their architectural flexibility: data fusion. Deep Learning conducted in Section V, and lessons learned therefrom are flexible architectures allow for the different format data types covered in Section VI. Challenges and research opportunities to be merged, combining the information of multiple sources related to short-term traffic forecasting are discussed in Section and extracting more knowledge about the process to model. VII. Finally, Section VIII ends this survey with a summary of Therefore, Deep Learning allows researchers to resolve com- final thoughts and an outlook. plex learning problems, specially when dealing with highly- dimensional data. II. CONCEPTS AND PRELIMINARIES Short-term traffic forecasting has been one of the cor- B. Short-term traffic forecasting nerstones for traffic management, as it is a reliable tool to The development of the short-term traffic forecasting field manage and maintain traffic networks. In turn, Deep Learning began when researchers started to apply time series forecasting comprehends a mixture of data-driven models which excellent methods to characterize traffic congestion measurements [20]. results in many applications have stimulated their widespread Back then, one popular approach relied on the assumption adoption for short-term
Details
-
File Typepdf
-
Upload Time-
-
Content LanguagesEnglish
-
Upload UserAnonymous/Not logged-in
-
File Pages25 Page
-
File Size-