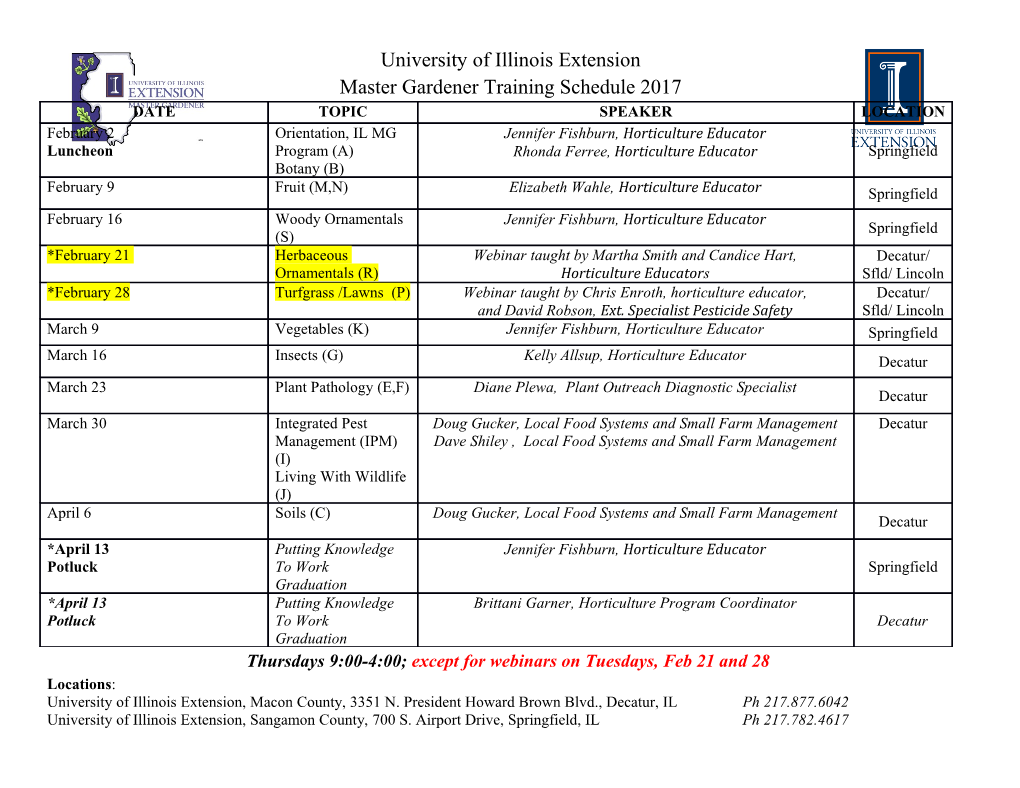
Uncertainty quantification in unfolding elementary particle spectra at the Large Hadron Collider THÈSE NO 7118 (2016) PRÉSENTÉE LE 22 JUILLET 2016 À LA FACULTÉ DES SCIENCES DE BASE CHAIRE DE STATISTIQUE MATHÉMATIQUE PROGRAMME DOCTORAL EN MATHÉMATIQUES ÉCOLE POLYTECHNIQUE FÉDÉRALE DE LAUSANNE POUR L'OBTENTION DU GRADE DE DOCTEUR ÈS SCIENCES PAR Mikael Johan KUUSELA acceptée sur proposition du jury: Prof. F. Nobile, président du jury Prof. V. Panaretos, directeur de thèse Prof. Ph. Stark, rapporteur Prof. S. Wood, rapporteur Prof. A. Davison, rapporteur Suisse 2016 “If everybody tells you it’s possible, then you are not dreaming big enough.” — Bertrand Piccard and André Borschberg, while crossing the Pacific Ocean on a solar-powered aircraft To my parents Acknowledgements I would first and foremost like to sincerely thank my advisor Victor Panaretos for the opportu- nity of carrying out this work as a member of the Chair of Mathematical Statistics at EPFL and for his tireless support and advice during these past four years. The opportunity of doing a PhD that combines statistical research with an intriguing applied problem from CERN was truly a dream come true for me and these years have indeed been very exciting and intellectually satisfying. I believe that during this work we have been able to make important contributions to both statistical methodology and to data analysis at the LHC and all this would not have been possible without Victor’s continuous support. Throughout this work, I have served as a Statistics Consultant on unfolding issues for the Statistics Committee of the CMS experiment at CERN. This interaction has significantly im- proved my understanding of the statistical issues that are pertinent to LHC data analysis and I hope that my contributions to the committee’s work have improved the way unfolding is carried out at the LHC. I would like to thank the Statistics Committee members, and especially Olaf Behnke, Bob Cousins, Tommaso Dorigo, Louis Lyons and Igor Volobouev, for stimulating discussions, which have often provided me with new insights and research directions. I am also grateful to Volker Blobel, Mikko Voutilainen and Günter Zech for very helpful discussions about unfolding. During autumn 2014, I was visiting Philip Stark at the Department of Statistics at the University of California, Berkeley. I am extremely grateful to Philip for agreeing to host me and to Victor for providing the opportunity for this visit. I would especially like to thank Philip for taking the time to discuss strict bounds confidence intervals with me. It was these discussions and the support of both Philip and Victor that eventually led to the work described in Chapter 7 of this thesis. During this work, I have had the great opportunity of discussing research questions with many outstanding statisticians. Besides Victor and Philip, I would especially like to thank Anthony Davison, Peter Green, George Michailidis and Simon Wood for discussions that were very helpful for this work. I would also like to thank Fabio Nobile, Victor Panaretos, Anthony Davison, Philip Stark and Simon Wood for agreeing to evaluate this thesis as members of my thesis committee and i Acknowledgements for providing insightful feedback on this work. I am also grateful to my colleagues Anirvan Chakraborty, Pavol Guriˇcan, Valentina Masarotto and Yoav Zemel for providing comments on selected parts of this thesis and to Marie-Hélène Descary for helping with the French translation of the abstract. I would like to gratefully acknowledge the Swiss National Science Foundation for partially funding this project. I am also grateful to EPFL for providing such a supportive, stimulating and international working environment and to CUSO for the organizing the fantastic statistics summer and winter schools up on the Swiss Alps. I would also like to thank all my friends in Lausanne, in Helsinki and in the Bay Area for all the memorable moments and fun experiences we have shared over the years. I am also grateful to the current and former SMAT, STAT and STAP group members for this nice time that we have spent together at EPFL. I would especially like to thank Yoav for being such a great officemate during these past 4.5 years and for helping me out with many mathematical questions. Finally, I would like to thank my parents for all their support during my studies which culmi- nate in this thesis. Lausanne, July 10, 2016 M. K. ii Abstract This thesis studies statistical inference in the high energy physics unfolding problem, which is an ill-posed inverse problem arising in data analysis at the Large Hadron Collider (LHC) at CERN. Any measurement made at the LHC is smeared by the finite resolution of the particle detectors and the goal in unfolding is to use these smeared measurements to make non- parametric inferences about the underlying particle spectrum. Mathematically the problem consists in inferring the intensity function of an indirectly observed Poisson point process. Rigorous uncertainty quantification of the unfolded spectrum is of central importance to particle physicists. The problem is typically solved by first forming a regularized point estima- tor in the unfolded space and then using the variability of this estimator to form frequentist confidence intervals. Such confidence intervals, however, underestimate the uncertainty, since they neglect the bias that is used to regularize the problem. We demonstrate that, as a result, conventional statistical techniques as well as the methods that are presently used at the LHC yield confidence intervals which may suffer from severe undercoverage in realistic unfolding scenarios. We propose two complementary ways of addressing this issue. The first approach applies to situations where the unfolded spectrum is expected to be a smooth function and consists in using an iterative bias-correction technique for debiasing the unfolded point estimator obtained using a roughness penalty. We demonstrate that basing the uncertainties on the variability of the bias-corrected point estimator provides significantly improved coverage with only a modest increase in the length of the confidence intervals, even when the amount of bias-correction is chosen in a data-driven way. We compare the iterative bias-correction to an alternative debiasing technique based on undersmoothing and find that, in several situations, bias-correction provides shorter confidence intervals than undersmoothing. The new methodology is applied to unfolding the Z boson invariant mass spectrum measured in the CMS experiment at the LHC. The second approach exploits the fact that a significant portion of LHC particle spectra are known to have a steeply falling shape. A physically justified way of regularizing such spectra is to impose shape constraints in the form of positivity, monotonicity and convexity. Moreover, when the shape constraints are applied to an unfolded confidence set, one can regularize the length of the confidence intervals without sacrificing coverage. More specifically, we form iii Abstract shape-constrained confidence intervals by considering all those spectra that satisfy the shape constraints and fit the smeared data within a given confidence level. This enables us to derive regularized unfolded uncertainties which have by construction guaranteed simultaneous finite-sample coverage, provided that the true spectrum satisfies the shape constraints. The uncertainties are conservative, but still usefully tight. The method is demonstrated using simulations designed to mimic unfolding the inclusive jet transverse momentum spectrum at the LHC. Keywords: bias-variance trade-off, deconvolution, empirical Bayes, finite-sample coverage, high energy physics, iterative bias-correction, Poisson inverse problem, shape-constrained inference, strict bounds confidence intervals, undersmoothing. iv Résumé Cette thèse traite de l’inférence statistique dans le cas du problème d’unfolding en physique des hautes énergies, qui est un problème inverse mal posé apparaissant lors de l’analyse des données au Grand collisionneur de hadrons (Large Hadron Collider (LHC) en anglais) au CERN. Toutes les mesures prises au LHC sont perturbées à cause de la résolution finie des détecteurs de particules et l’objectif du unfolding est d’utiliser ces mesures corrompues afin de faire de l’inférence non-paramétrique sur le spectre sous-jacent des particules. D’un point de vue mathématique, le problème consiste à inférer la fonction d’intensité d’un processus de Poisson ponctuel observé indirectement. La quantification rigoureuse de l’incertitude du spectre unfoldé est d’une importance ma- jeure pour les physiciens. Le problème est typiquement résolu en formant tout d’abord un estimateur ponctuel régularisé dans l’espace unfoldé et en utilisant par la suite la variabilité de cet estimateur afin de former des intervalles de confiance fréquentistes. Cependant, de tels intervalles sous-estiment l’incertitude puisqu’il néglige le biais introduit lors de la régulari- sation du problème. Nous démontrons qu’il en résulte que les techniques conventionnelles en statistique ainsi que les méthodes qui sont présentement utilisées au LHC produisent des intervalles de confiance qui peuvent présentés de sérieux problèmes de sous-couverture pour des scénarios réalistes d’unfolding. Nous proposons deux façons complémentaires afin d’aborder ce problème. La première approche s’applique aux situations pour lesquelles on s’attend à ce que le spectre soit une fonction lisse et elle utilise une technique itérative de correction du biais
Details
-
File Typepdf
-
Upload Time-
-
Content LanguagesEnglish
-
Upload UserAnonymous/Not logged-in
-
File Pages207 Page
-
File Size-