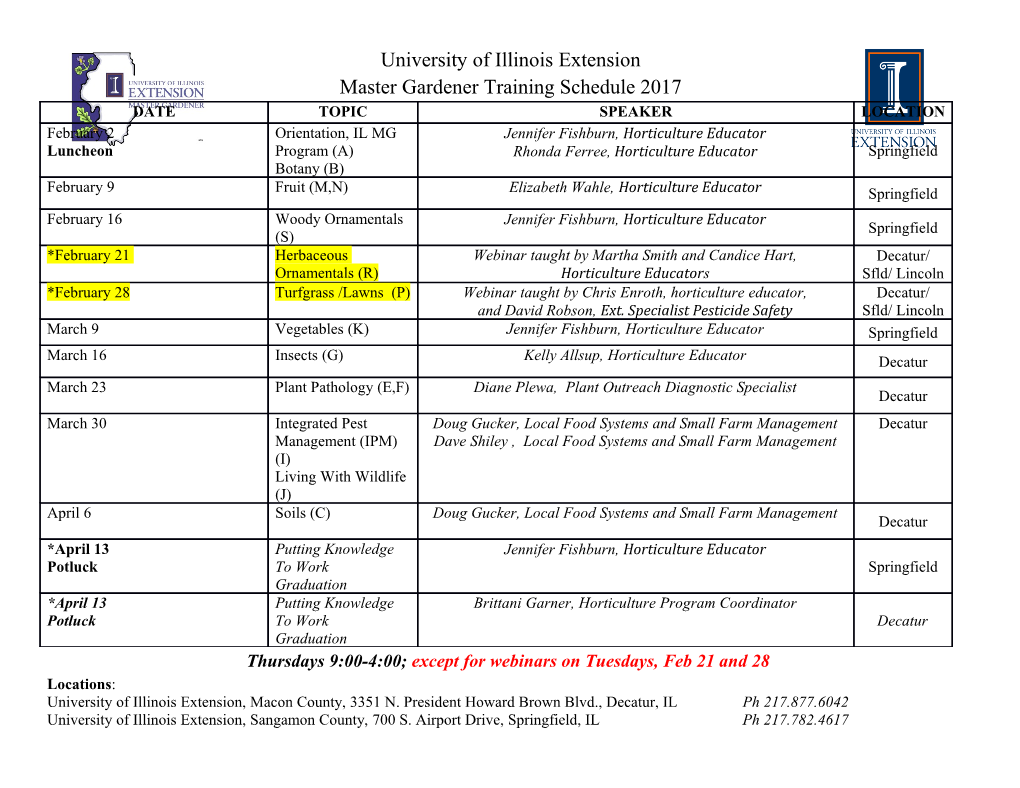
Environmental Earth Sciences (2021) 80:75 https://doi.org/10.1007/s12665-020-09345-0 ORIGINAL ARTICLE Developing geographic weighted regression (GWR) technique for monitoring soil salinity using sentinel‑2 multispectral imagery Mohammad Mahdi Taghadosi1 · Mahdi Hasanlou1 Received: 6 June 2020 / Accepted: 16 December 2020 © The Author(s), under exclusive licence to Springer-Verlag GmbH, DE part of Springer Nature 2021 Abstract Soil salinity is a widespread natural hazard that negatively infuences soil fertility and crop productivity. Using the potential of earth observation data and remote sensing technologies provides an opportunity to address this environmental issue and makes it possible to identify salt-afected regions accurately. While most of the utilized methods and model development techniques for monitoring soil salinity to date have been globally considered and tried to detect salinity and create predic- tive maps with a single regression algorithm, fewer studies have investigated the potential of local models and weighted regression techniques for estimating soil salinity. Accordingly, this research deals with monitoring surface soil salinity by the potential of Sentinel-2 multispectral imagery using the geographic weighted regression (GWR) technique. The feld study was conducted in an area that has sufered from salinization, and the salinity of several soil samples was measured to be used as a source of ground truth data. The most efcient satellite features, which accurately predict surface soil salinity by its higher spectral refectance, were derived from the Sentinel-2 data to be used as explanatory variables in the analysis. The GWR algorithm was then implemented with a fxed Gaussian kernel, and the optimized bandwidth was calculated in a calibration process using the cross-validation score (CV score). The results of the analysis proved that the GWR method has a great capability to predict soil salinity with an accuracy of two decimal places. The visual interpretation of the local estimates of coefcients and local t-values for each predictor variable has also been provided, which highlights the local variations in the study site. Finally, the achieved results were compared with the outcomes obtained from implementing two global regression techniques, Support Vector Machines (SVM), and Multiple Linear Regression (MLR), which confrmed the higher performance of the GWR algorithm. Keywords Soil salinity · Sentinel-2 multispectral imagery · Geographic weighted regression · Local variation Introduction regions (Elhag 2016; Cuevas et al. 2019). Accordingly, in the last few years, the potential of modern technologies and Soil salinity is a natural environmental hazard that has a advanced methods like remote sensing (RS) has been widely destructive infuence on soil quality and crop productiv- investigated for monitoring soil salinity (Taghadosi et al. ity in agricultural lands (Allbed and Kumar 2013; Gorji 2018). Remotely sensed data makes it possible to monitor et al. 2017). Regarding the importance of conservation of soil salinity rapidly and cost-efectively and can characterize natural resources and to increase the sustainability of crop salt-afected regions at larger scales due to its wide spatial production, a reliable method should be sought for soil coverage (Brunner et al. 2007; Allbed and Kumar 2013). reclamation and preventing further salinization of afected Over the past few years, optical remotely sensed data have been widely used for salinity monitoring through high spec- tral refectance of salt-afected regions in the visible and * Mahdi Hasanlou [email protected] near-infrared range of the electromagnetic spectrum. The main strategy is to establish a relationship between satellite Mohammad Mahdi Taghadosi [email protected] features as predictor variables and measured soil salinity values as responses to build a satellite-based model for salin- 1 School of Surveying and Geospatial Engineering, College ity estimation in study sites. A variety of satellite features, of Engineering, University of Tehran, P. O. Box 11155-4563, known as salinity indices (SI), which are mostly developed 1439957131 Tehran, Iran Vol.:(0123456789)1 3 75 Page 2 of 14 Environmental Earth Sciences (2021) 80:75 based on the ratio between the visible and NIR spectral analysis showed an accuracy of no more than R2 = 0.52 and bands, have been investigated for this task to construct a R2 = 0.61 for HH and VV polarization, respectively. high precision mathematical model for soil salinity estima- One of the main obstacles in providing accurate results tion. However, the obtained accuracies in previous research for monitoring and mapping soil salinity by the potential of have been varied and largely depend on various factors such RS is that the data analysis procedure and algorithm devel- as the spatial/spectral resolutions of sensors, the used meth- opment techniques are usually globally considered. This may odology, study sites and other related issues. Such variations weaken the strength and efciency of predictive models in in model performance to predict target variables through estimating dependent variables on a large scale because the remote sensing and machine learning approaches are not model tries to estimate coefcients and predict all dependent limited to soil salinity studies, but expand to other related variables at the same time and with only one global equa- felds, as reported in Veronesi and Schillaci (2019). They tion (Mennis 2006; Matthews and Yang 2012). So that the compared popular regression algorithms for soil organic car- accuracy cannot go beyond a certain limit. In other words, it bon (SOC) mapping in semi-arid Mediterranean region of would be difcult to consider an inclusive model to predict Sicily, and pointed out it is unclear from the literature which salinity at a regional scale for soils of diferent character- technique performs well for SOC mapping. They mentioned istics (e.g., soil texture, constituents, moisture level, etc.). that uncertainty is a challenging issue in predicting response The situation exacerbates when soils with diferent land variables by machine learning strategies. cover types are considered (Taghadosi and Hasanlou 2017). There have been similar fndings in the literature that For instance, in green areas, spectral refectance of vegeta- imply the accuracy of a satellite-based model depends on tion covers or plants decreases due to salinity occurrence, varying factors, and developing regression algorithms to pre- whereas in bare soil land salt-afected regions can be easily dict target variables may be a challenging issue. Zhang et al. detected through high spectral refectance of salt deposits, (2015) evaluated the potential of MODIS time series vegeta- which are apparent in the site. In such conditions, the spatial tion indices data for monitoring soil salinity in the Yellow locations of salinity occurrence in the scene and the salinity River Delta, China. The results showed that the correla- ranges, play a signifcant role in properly estimating soil tion of the satellite-derived feature and soil salinity highly salinity, which suggests using local models and weighted depends on land cover heterogeneity. The best ft model regression techniques for modeling and mapping salinity reached no more than R2 = 0.70 using a binomial regres- rather than a global model for the entire scene. Using Geo- sion model. Some authors also investigated the potential of graphic Weighted Regression (GWR) method enables us to various satellite sensors and their derived features to predict signifcantly improve the models’ accuracy and guarantee salinization. Ivushkin et al. (2019) used three diferent UAV the strength and reliability of the predictive maps (Wang sensors to measure the salinity stress of quinoa plants in the et al. 2005; Kala et al. 2017; Guo et al. 2017) because it Netherlands. The multiple linear regression model was used builds a unique model for each regression point according to relate remotely sensed data and ground truth data meas- to the spatial location of observations (Murayama 2012). urements. However, the achieved results reached no more Despite the great potential of the GWR method in mod- than R2 = 0.46 when the acquired data from all three sensors eling scale-dependent and nonstationary relationships were combined. In another study, Allbed et al. (2014) used between predictor and response variables, limited studies the high spatial resolution IKONOS satellite data with pixel have focused on addressing an environmental issue by the size 1 m to assess soil salinity in the area that was dominated potential of remotely sensed data and the GWR technique. In by date palm. Various salinity and vegetation indices were a case study in China, Li et al. (2010) used the GWR method investigated to predict soil salinity in the site, and the best to investigate the nonstationary spatial relationships between results were achieved with R2 = 78% using SAVI and SI-T Land Surface Temperature (LST) of an urban area and envi- indices. In a case study in India, George and Kumar (2015) ronmental factors. The results of the analysis indicated that investigated the potential of Hyperion hyperspectral satel- the GWR method has a higher performance than the tradi- lite data to map various soil salinity levels. The Support tional Ordinary Least Square (OLS) model and also provides Vector Machine technique was used as a classifer, and the detailed information about the local variation of LST in the map
Details
-
File Typepdf
-
Upload Time-
-
Content LanguagesEnglish
-
Upload UserAnonymous/Not logged-in
-
File Pages14 Page
-
File Size-