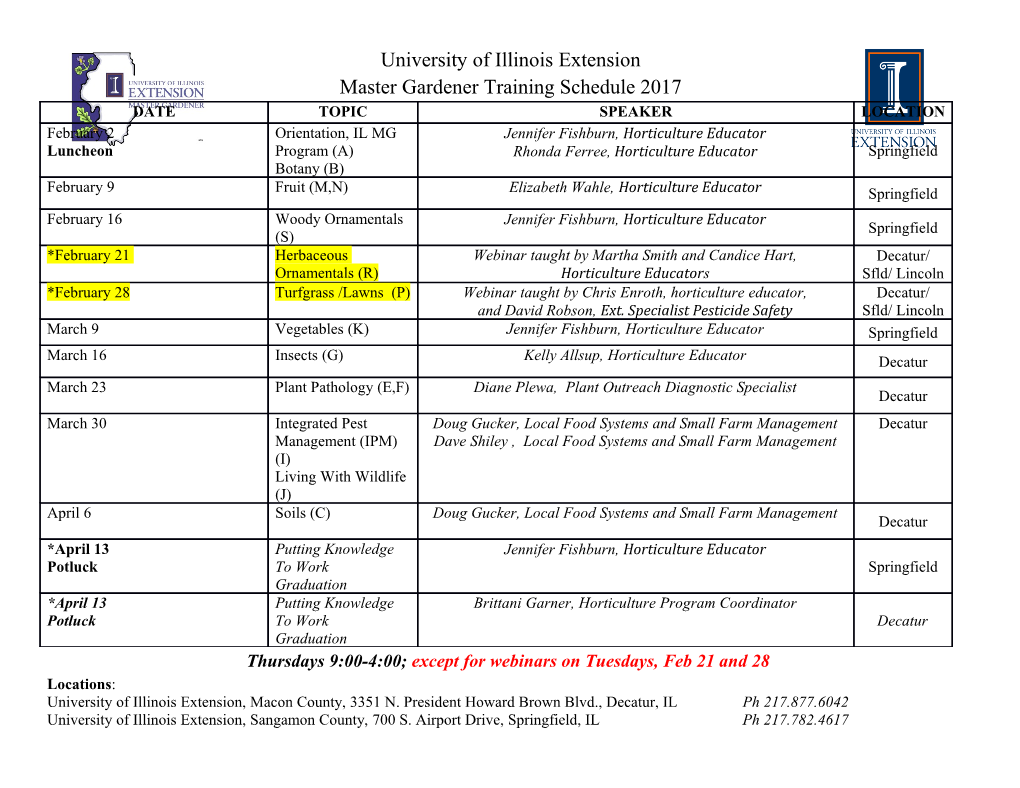
Improving Drug Discovery Efficiency via In Silico Calculation of Properties D. Ortwine 1 16th North American Regional ISSX meeting , 10/18/09 Outline • Background: Why Calculate Properties? • Calculable properties • Modeling Methods and Molecule Descriptors • Reporting Results From Calculations • Available Commercial Software • Strategies for Implementation • A Real Project Example • The Future • Conclusions • References 2 16th North American Regional ISSX meeting , 10/18/09 Lead Optimization in Drug Discovery The Needle in the Haystack 3 16th North American Regional ISSX meeting , 10/18/09 Why Calculate Properties? They can be related to the developability of drugs! Most marketed oral drugs have defined “property profiles” Paul D. Leeson and Brian Springthorpe, “The Influence of Drug-Like Concepts on Decision-Making in Medicinal Chemistry”, Nature Reviews Drug Discovery, vol. 6, pp. 881-890, 2007. Mark C. Wenlock, et.al, “A Comparison of Physiochemical Property Profiles of Development and Marketed Oral Drugs”, J. Med. Chem, 2003, 46, 1250-1256. 4 16th North American Regional ISSX meeting , 10/18/09 Why Calculate Properties? They can also be related to the ADMET Profile M. Paul Gleeson. Generation of a Set of Simple, Interpretable ADMET Rules of Thumb. J. Med. Chem. (2008), 51(4), 817-834. 5 16th North American Regional ISSX meeting , 10/18/09 Why Calculate Properties? • Prioritize synthesis -> Generate virtual individual molecules or combinatorial libraries, calculate properties, map back to R groups • Build an understanding of SAR • Combine with docking scores in a multiparameter optimization paradigm • Assist HTS triage • Replace measurements • Guide the growth of the compound collection • Guide the subsetting of the compound collection 6 16th North American Regional ISSX meeting , 10/18/09 7 16th North American Regional ISSX meeting , 10/18/09 Calculable Properties Descriptive Binding, 3D Shape N+O, Donors, Rings, Docking scores, TPSA, Size, Similarity, Fit to a pharmacophore, Connectivity Shape overlap Physiochemical MW, Log P, Log D, pKa, Composite Solubility, Polarizability, Ligand Efficiency, Critical packing (crystallinity) Ligand Lipophilic Efficiency, Cellular Efficiency DMPK LM, Hep Stability, Accuracy Calculation Difficulty PPB, Permeability, High Easy Vdiss, Cyp inhibition, Moderate Medium Metabolic ‘hotspots’ Low Hard 8 16th North American Regional ISSX meeting , 10/18/09 Property Definitions TPSA Topological polar surface area <120 desirable; <80 for CNS drugs LE Ligand Efficiency = -1.4 logKi / # of heavy atoms 1 0.3 is a good hit; 0.35-0.5 is a good clinical candidate LLE Ligand Lipophilic Efficiency = -log(Ki) – logD 2 7-9 is a good clinical candidate CellE Cellular Efficiency = -log( in vitro Ki) – log(cellular EC50) 0 is goal, <1.5 is acceptable 1Andrew Hopkins, et.al., Drug Discovery Today, 2004, 9, 430-431. 2Paul Leeson and Brian Springthorpe, Nature Reviews Drug Discovery, 2007, 6, 881-890. 9 16th North American Regional ISSX meeting , 10/18/09 Calculated vs Measured pKa R2 = 0.82 n = 133 s = 1.5 (75 compounds) (intercept not signif.) Calculated (MoKa(*) v1.0.3) v1.0.3) (MoKa(*) (MoKa(*) Calculated Calculated (*) http://www.moldiscovery.com Measured 10 16th North American Regional ISSX meeting , 10/18/09 Calcd. (Volsurf+) vs Expt’l. Thermodynamic Sol’y. (970 compounds) s = 0.8 log units G. Cruciani, P. Crivori, P.-A. Carrupt, B. Testa. Journal of Molecular Structure: THEOCHEM 503, 17-30, 2000. Volsurf+ manual (http://www.moldiscovery.com). 11 16th North American Regional ISSX meeting , 10/18/09 It’s Not Just About Lipophilicity Calculated Solubility vs. cLogD7.4 cLogS, µµµM (Stardrop (*) software) (*) http://www.optibrium.com/ cLogD7.4 12 16th North American Regional ISSX meeting , 10/18/09 Methods for Deriving DMPK Models • Regression • Partial Least Squares • Neural Networks • Discriminant Analysis (ADAPT, SIMCA, Support Vector Machines) • Decision Trees (Random Forest) • Baysean Methods (probabilistic approaches) • Use of 3D Structure of Target (CYPs, Transporters, Efflux Pumps,...) Models are derived using a subset of compounds, then the property is predicted for the held-out compounds (prediction or validation set) 13 16th North American Regional ISSX meeting , 10/18/09 Molecule Descriptors Used to Derive DMPK Models • Molecular fingerprints (Pipeline Pilot, MOE, Unity) • General molecular descriptors (MDL keys, OEchem) • Calculated properties (AlogP, ClogP, TPSA, ...) • Connectivity descriptors (e-state keys from Molconn-Z) • Geometrically derived (pharmacophores, from 3D structure of target correlograms in MetaSite) 14 16th North American Regional ISSX meeting , 10/18/09 Reporting Results • Predicted value • Confidence in the prediction (std. error, probability, quality of prediction) • The nearest neighbor in the training set (Tc* is typically used) • The number of near neighbors in the training set (above a Tc threshhold) • Geometric fit score (docking, pharmacophore overlap, shape similarity) • Details (model version, date) *Tc = Tanimoto coefficient = difference in binary fingerprints between two compounds = 15 16th North American Regional ISSX meeting , 10/18/09 Reporting Results: Example (*) cLM_HRM = Calculated liver microsomal stability in Human, Rat, and Mouse Result, probability, and error are reported (*) Output from a property calculator used at Genentech 16 16th North American Regional ISSX meeting , 10/18/09 Combining 2D and 3D Worlds 17 16th North American Regional ISSX meeting , 10/18/0I. Aliagas9 Comparing Multiple Ligands 18 16th North American Regional ISSX meeting , 10/18/09 Commercial Software • Pipeline Pilot v7.5 Accelrys • Stardrop Optibrium • MoKa, MetaSite, Volsurf+ Molecular Discovery • ACDlabs Molecular Discovery Ltd. • ADME Boxes PharmaAlgorithms • ADMET predictor Simulations Plus (Now merged with Molecular Discovery Ltd.) • SARchitect Strand Life Sciences • Metabolizer ChemAxon • Spotfire (visualization) Tibco • Vortex (visualization) Dotmatics 19 16th North American Regional ISSX meeting , 10/18/09 From Molecular Discovery Ltd. 20 16th North American Regional ISSX meeting , 10/18/09 Strategies For Implementation Obtain commercial software or develop your own -> A full-featured ‘chemically aware’ graphing package is a must Try generating global models on DMPK endpoints first ->If this fails, try project- or chemotype- specific models Report probabilities and errors along with the calculated values Track calculated vs. measured values on a regular basis -> Continually check the models’ performance (predicted vs. measured) -> Update the model regularly with new data -> Regularly discuss results with chemists Add calculated properties to your compound database -> Facilitates searching, subsetting, and rank ordering of compounds 21 16th North American Regional ISSX meeting , 10/18/09 “Real Project” Example 22 16th North American Regional ISSX meeting , 10/18/09 Solubility: Calculated vs Measured (Kinetic) Rough correlation is evident: Used as a guideline/filter on proposed compounds Average cLogS, µµµM (from Stardrop software) # of cpds Measured LogS (kinetic) 23 16th North American Regional ISSX meeting , 10/18/09 Performance of Human Liver Microsome (HLM) Stability Model Strong correlation is evident: Used as a guideline/filter on proposed compounds cHLM CL_Hep Probability # of cpds Measured HLM CL_Hep (mL/min/kg) 24 16th North American Regional ISSX meeting , 10/18/09 Predictivity of HLM Stability Model >14.5 6.2-14.5 <6.2 ( mL/min/kg) Calculated HLM Probability 25 16th North American Regional ISSX meeting , 10/18/09 PPB Model Validation: Human, Rat, Mouse (Training set, 750 compounds; Test set, ~250 compounds) Human Rat Mouse Predicted PPB >95% Predicted PPB <95% 26 16th North American Regional ISSX meeting , 10/18/09 Calculated Solubility vs PK Rat F% Average cLogS, µµµM (from Stardrop software) # of cpds PK Rat F% 27 16th North American Regional ISSX meeting , 10/18/09 In Vitro vs. Cellular Potency Disconnects In Vitro IC50 vs Cellular EC50 R2 = 0.46 -log( in vitro IC50) What causes the shift? -log(cellular EC50) 28 16th North American Regional ISSX meeting , 10/18/09 In Vitro vs. Cellular Potency Disconnects Looking for Relationships With Calculated Properties # of cpds CellE In Vitro vs Cellular Potency 29 16th North American Regional ISSX meeting , 10/18/09 Calcd. Solubility vs. Cellular Potency Average cLogS, µµµM (from Stardrop software) # of cpds -log(Cellular EC50) 30 16th North American Regional ISSX meeting , 10/18/09 Increased Sol’y. Reduces In Vitro/Cellular Pcy. Disconnect Average cLogS, µµµM (from Stardrop software) # of cpds CellE (in vitro/cellular potency) 31 16th North American Regional ISSX meeting , 10/18/09 CellE In Vitro vs Cellular potency by R Group Average CellE 1000x 100x 10x 1x # of cpds 32 16th North American Regional ISSX meeting , 10/18/09 cLogD: Progress Over Time cLogD7.4 Compound Registration Date 33 16th North American Regional ISSX meeting , 10/18/09 Ligand Lipophilic Efficiency(*): Progress Over Time LLE Compound Registration Date (*) LLE = pIC50 – cLogD7.4 [Values of 7-9 Desirable] 34 16th North American Regional ISSX meeting , 10/18/09 Whole Blood Potency: Progress Over Time -log(EC50) 100nM 1 µM 10 µM 100 µM Compound Registration Date 35 16th North American Regional ISSX meeting , 10/18/09 Stability in Hepatocytes: Progress Over Time CLhep, mL/min/kg Compound Registration Date 36 16th North American Regional ISSX meeting , 10/18/09 Med Chem Prioritizes Based on Filters 7041 compound targets - 87% cHLMprob >0.6 884 67%
Details
-
File Typepdf
-
Upload Time-
-
Content LanguagesEnglish
-
Upload UserAnonymous/Not logged-in
-
File Pages42 Page
-
File Size-