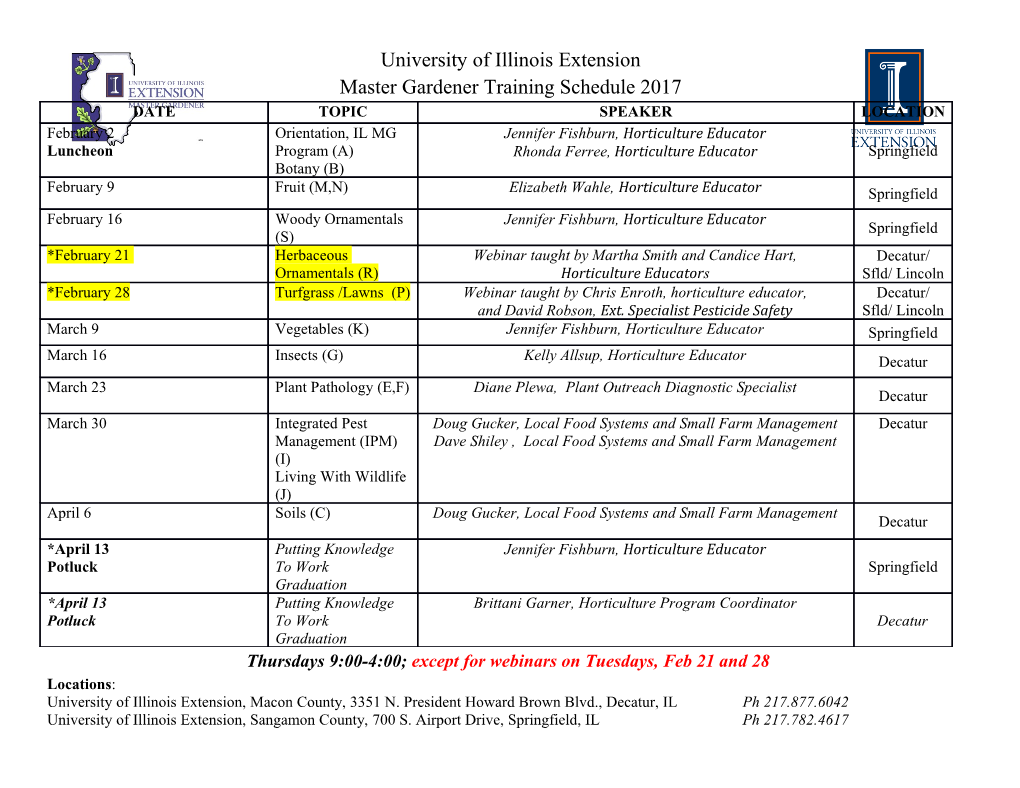
Automated Identification of Security Issues from Commit Messages and Bug Reports Yaqin Zhou Asankhaya Sharma SourceClear, Inc. SourceClear, Inc. [email protected] [email protected] ABSTRACT 1 INTRODUCTION The number of vulnerabilities in open source libraries is increasing To aid in the software development, companies typically adopt rapidly. However, the majority of them do not go through public issue-tracking and source control management systems, such as disclosure. These unidentied vulnerabilities put developers’ prod- GitHub, JIRA, or Bugzilla. In fact, as of April 2017, GitHub reports ucts at risk of being hacked since they are increasingly relying on having almost 20 million users and 57 million repositories. Accord- open source libraries to assemble and build software quickly. To ing to Atlassian, JIRA is used by over 75,000 companies. These nd unidentied vulnerabilities in open source libraries and se- tools are very popular for open source projects, and are essential cure modern software development, we describe an ecient au- to modern software development. Developers work on reported is- tomatic vulnerability identication system geared towards track- sues in these systems, then commit corresponding code changes ing large-scale projects in real time using natural language pro- to GitHub (or other source code hosting platforms, e.g. SVN or Bit- cessing and machine learning techniques. Built upon the latent in- Bucket). Bug xes and new features are frequently merged into a formation underlying commit messages and bug reports in open central repository, which is then automatically built, tested, and source projects using GitHub, JIRA, and Bugzilla, our K-fold stack- prepared for a release to production, as part of the DevOps prac- ing classier achieves promising results on vulnerability identica- tices of continuous integration (CI) and continuous delivery (CD). tion. Compared to the state of the art SVM-based classier in prior There is no doubt that the CI/CD pipeline helps improve de- work on vulnerability identication in commit messages, we im- veloper productivity, allowing them to address bugs more quickly. prove precision by 54.55% while maintaining the same recall rate. However, a signicant amount of security-related issues and vul- For bug reports, we achieve a much higher precision of 0.70 and nerabilities are patched silently, without public disclosure, due to recall rate of 0.71 compared to existing work. Moreover, observa- the focus on fast release cycles as well as the lack of manpower and tions from running the trained model at SourceClear in production expertise. According to statistics collected at SourceClear [2], 53% for over 3 months has shown 0.83 precision, 0.74 recall rate, and of vulnerabilities in open source libraries are not disclosed publicly detected 349 hidden vulnerabilities, proving the eectiveness and with CVEs. For Go and JavaScript language libraries, the percent- generality of the proposed approach. age is as high as 78% and 91% respectively. In today’s world of agile software development, developers are CCS CONCEPTS increasingly relying on and extending free open source libraries to get things done quickly. Many fail to even keep track of which open • Security and privacy → Software security engineering; source libraries they use, not to mention the hidden vulnerabilities that are silently patched in the libraries. So even if the vulnerabil- KEYWORDS ity is xed in source code as seen by the commit message or bug vulnerability identication, machine learning, bug report, commit report, there may be users of the library who are not made aware of the issue and continue using the old version. Unaware of these ACM Reference format: hidden vulnerabilities, they are putting their products at risk of be- Yaqin Zhou and Asankhaya Sharma. 2017. Automated Identication of Se- ing hacked. Thus, it is important to locate such silent xes as they curity Issues from Commit Messages and Bug Reports. In Proceedings of can be used to help developers decide about component updates. 2017 11th Joint Meeting of the European Software Engineering Conference Motivated to nd the unidentied vulnerabilities in open source and the ACM SIGSOFT Symposium on the Foundations of Software Engineer- libraries and secure modern software development, in this paper ing, Paderborn, Germany, September 4-8, 2017 (ESEC/FSE’17), 6 pages. we describe an automatic vulnerability identication system that https://doi.org/10.1145/3106237.3117771 tracks a large number (up to 10s of thousands) of open source li- braries in real time at low cost. Many techniques, such as static and dynamic analysis, are em- Permission to make digital or hard copies of all or part of this work for personal or ployed to ag potentially dangerous code as candidate vulnerabil- classroom use is granted without fee provided that copies are not made or distributed for prot or commercial advantage and that copies bear this notice and the full cita- ities, but they are not appropriate for tracking existing unknown tion on the rst page. Copyrights for components of this work owned by others than vulnerabilities on a large scale, at low cost (due to false positives). ACM must be honored. Abstracting with credit is permitted. To copy otherwise, or re- publish, to post on servers or to redistribute to lists, requires prior specic permission Firstly, these tools can only support one or two specic languages and/or a fee. Request permissions from [email protected]. and certain patterns of vulnerabilities well. For example, the static ESEC/FSE’17, September 4-8, 2017, Paderborn, Germany analysis tool FlawFinder [19] is limited to nding buer overow © 2017 Association for Computing Machinery. risks, race conditions, and potential shell meta-character usage in ACM ISBN 978-1-4503-5105-8/17/09...$15.00 https://doi.org/10.1145/3106237.3117771 C/C++. Moreover, most of these approaches operate on an entire 914 ESEC/FSE’17, September 4-8, 2017, Paderborn, Germany Yaqin Zhou and Asankhaya Sharma software project and deliver long lists of potentially unsafe code the underlying issues due to imprecision of analysis. For example, with extremely high false positive rates (i.e., 99% shown in [14]). RATS does not nd Cross-Site Scripting (XSS) or SQL Injection vul- They require a considerable amount of manual review and thus nerabilities. Moreover, when applied to real world projects, these are not suitable to track projects at large scale. tools raise massive false positives that are hard to reduce. For most software systems, bugs are tracked via issue trackers Dynamic analysis analyzes the source code by executing it on and code changes are merged in the form of commits to source real inputs. Basic dynamic analysis (or testing) tools search for vul- control repositories. Therefore, it is convenient to check these ba- nerabilities by trying a wide range of possible inputs. There are sic artifacts (a new bug report or commit) of software development also dynamic taint analysis tools that do taint tracking at runtime to detect vulnerabilities in real time. Besides, source code changes, [1, 3, 13]. For example, PHP Aspis does dynamic taint analysis to bug reports and commits messages contain rich contextual infor- identify XSS and SQL vulnerabilities [13]. ZAP [1], a popular test- mation expressed in natural language that is often enough for a ing tool in industry, nds certain types of vulnerabilities in web security researcher to determine if the underlying artifact relates applications. It requires users to dene scan policies before scan- to a vulnerability or not. ning and perform manual penetration testing after scanning. Building on these insights, we automate the above identica- Symbolic execution [8] is a technique that exercises various code tion process via natural language processing and machine learning paths through a target system. Instead of running the target system techniques. From GitHub, JIRA, and Bugzilla, we collected a wide with concrete input values like dynamic analysis, a symbolic execu- range of security related commits and bug reports for more than tion engine replaces the inputs with symbolic variables which ini- 5000 projects, created between Jan. 2012 and Feb. 2017, to build tially could be anything, and then runs the target system. Cadar et commits and bug reports datasets with ground truth. The collected al. [5] present KLEE, an open-source symbolic execution tool to an- data, however, demonstrates a highly imbalanced nature, where alyze programs and automatically generate system input sets that vulnerability-related commits and bug reports are less than 10%. achieve high levels of code coverage. However, it requires manual Hence, it is challenging to train classiers to identify vulnerabili- annotation and modication of the source code. Also, like most ties with good performance. To address it, we design a probability- symbolic execution tools, runtime grows exponentially with the based K-fold stacking algorithm that ensembles multiple individ- number of paths in the program which leads to path explosion. ual classiers and exibly balances between precision and recall. Machine learning. Besides the above techniques that focus exclu- We summarize our major contributions below. sively on the raw source code, machine-learning techniques pro- • We present an automatic vulnerability identication sys- vide an alternative to assist vulnerabilities detection by mining con- tem that ags vulnerability-related commits and bug re- text and semantic information beyond source code. Among these ports using machine learning techniques. Our approach works, some [11, 16, 17, 20, 20] focus on detecting vulnerabilities can identify various vulnerabilities regardless of program- combined with program analysis. For instance, Shar et al. [17] fo- ming languages at low cost and large scale. Our proposed cus on SQL injection and cross-site scripting while Sahoo et al. [16] K-fold stacking model for commits outperforms the state investigate malicious URL detection. of the art SVM model by 54.55% in precision.
Details
-
File Typepdf
-
Upload Time-
-
Content LanguagesEnglish
-
Upload UserAnonymous/Not logged-in
-
File Pages6 Page
-
File Size-