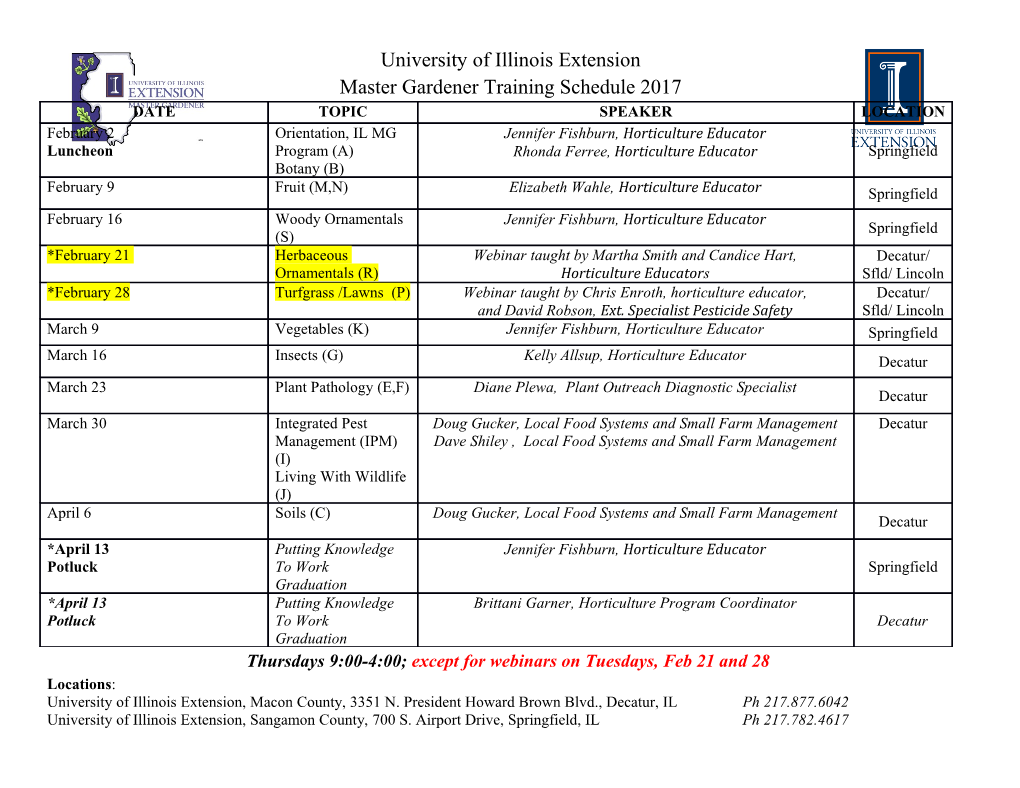
Algebraic Geometry and Model Selection American Institute of Mathematics 2011/Dec/12-16 I would like to thank Prof. Russell Steele, Prof. Bernd Sturmfels, and all participants. Thank you very much. Sumio Watanabe Tokyo Institute of Technology Contents 1. AIC, BIC, and DIC 2. Birational Invariants 3. Singular Fluctuation 4. Open Questions 1 AIC, BIC, and DIC 12/15/2011 Birational probability theory 3 Statistical Model and True Distribution N d x ∈ R , w ∈W(compact)⊂R (1) True dist. q(x) i.i.d. X1, X2, …, Xn (2) Statistical model p(x|w) (3) Prior dist. j(w) (Remark) E[ ] shows the expectation over X1,X2,…,Xn. Ex[ ] does that over X whose prob. dist. is q(x). 12/15/2011 Birational probability theory 4 Statistical Estimation Posterior Dist. n ( ) P p(Xi|w) j(w) dw i=1 E [ ] = w n P p(Xi|w) j(w) dw i=1 Predictive Dist. p*(x) = Ew[ p(x|w) ] (Remark) In Bayesian estimation, the true distribution q(x) is estimated by p*(x). 12/15/2011 Birational probability theory 5 Stochastic Complexity and Generalization Loss (1) Stochastic Complexity = - (Bayes Marginal) n F = - log P p(Xi|w) j(w) dw i=1 (2) Generalization Loss G = - Ex[ log p*(x) ] = S + KL( q(x) || p*(x) ) (Remark) S = -Ex[log q(X)] is the entropy of q(x). 6 12/15/2011 Birational probability theory BIC(Schwarz,1978) (1) BIC = - S log p(Xi|wMLE) + (d/2) log n If the posterior ~ normal distribution. F = BIC + Op(1). In general, yesterday, we learned RLCT In our workshop, Dr. Lin: Relation to asymptotic integral. F = - S log p(Xi|wMLE) + l log n Dr. Drton: How to use in statistics. – (m-1)loglog n + Op(1). Dr. Leykin: D-module and b-function. 12/15/2011 Birational probability theory 7 AIC(Akaike,1974) & DIC(Spiegelhalter,et.al. 2002) (2) AIC = - S log p(Xi|wMLE) + d (3) DIC = - S log Ew[p(Xi|w) ] + 2 S {-Ew[log p(Xi|w)]+log p(Xi| Ew[w] ) } If the posterior ~ the normal distribution. E[AIC]= n E[G]+o(1), E[DIC]= n E[G]+o(1). If otherwise, such relations do not hold. 12/15/2011 Birational probability theory 8 Estimation of G and F in regular cases AIC, DIC BIC Main Term Random Constant Estimated (Order 1) (log n) Consistency in model selection No Yes Unbiased Yes No Estimator of G 12/15/2011 Birational probability theory 9 2 Birational Statistics 12/15/2011 Birational probability theory 10 Birational Invariant If a value that is defined using resolution of singularities does not depend on the choice of resolution, then it is called a birational invariant. g1 U1 g2 U2 W g3 U3 12/15/2011 Birational probability theory 11 Nature in Statistics It is natural that statistical theory should be made to be invariant under birational transform. Fisher’s asymptotic theory does not satisfy such invariance. For example, it is not invariant under blow-up. Y = aX + b + Noise a = c =c’d’ b = cd=d’ Asymptotic normality of (a,b) holds, whereas that of (c,d) in projective space not . 12/15/2011 Birational probability theory 12 Differential and Birational Algebraic geometry studies mathematical properties those are invariant under the birational transform. diffeo birational To construct birational statistics might be one of the purposes of algebraic statistics. 12/15/2011 Birational probability theory 13 Singular Fluctuation 3 and model selection 12/15/2011 Birational probability theory 14 Loss for estimation Predictive Dist. p*(x) = Ew[ p(x|w) ] Generalization Loss Gn = - Ex[ log Ew[p(X|w)] ] Training Loss n Tn = - (1/n) S log Ew[p(Xi|w)] i=1 12/15/2011 Birational probability theory 15 Functional Cumulant Def. Two Cumulant Generating Functions a g(a)= - Ex[ log Ew[p(X|w) ] ] n a t(a) = - (1/n) S log Ew[p(Xi|w) ] i=1 Then g(0)=t(0)=0, and Gn=g(1), Tn= t(1). 12/15/2011 Birational probability theory 16 Invariance Two functions g(a) and t(a) are invariant under w = g(u) p(x|w) = p(x|g(u)) j(w) dw = j(g(u)) |g’(u)| du Cumulant generating function = Birational invariant generating function Example. l = lim n { E[g(1)]-S } n o o Birational probability theory Notation Def. Log density ratio function f(x,w)=log( q(x)/p(x|w) ). Then log p(x|w)= log q(x) + f(x,w). Birational probability theory Expansion of g(a) g(a) is rewritten as g(a) = aS - Ex[ log Ew[exp(-af(X,w))] ] Therefore g’(0) = S + Ex[ Ew[f(x,w)] ] 2 2 g’’(0) = - Ex[ Ew[f(x,w) ] - Ew[f(x,w)] ] 12/15/2011 19 Birational probability theory Expansion of t(a) By the same way, n t(a) = aSn - (1/n) S log Ew[exp(-af(Xi|w))] i=1 Therefore n t’(0) = Sn + (1/n) S Ew[f(Xi,w)] i=1 n 2 2 t’’(0) = - (1/n) S { Ew[f(Xi,w) ] - Ew[f(Xi,w)] } i=1 12/15/2011 20 Birational probability theory Functional Variance Def. Two random variables V1 = n Ex[ Ew[f(x,w)] ] – l n 2 2 V2 = S { Ew[ (log p(Xi|w) ) ] - Ew[ log p(Xi|w) ] } i=1 Remark. V2 can be calculated by samples and a model without any information about true dist.. In order to calculate V1, we need the information of the true distribution. 12/15/2011 Birational probability theory 21 Singular Fluctuation Theorem. Convergences hold, V1 V1* E[V1] E[V1*] V2 V2* E[V2 ] E[V2*] Theorem and Def. Singular Fluctuation E[V1*] = E[V2*] = 2n Remark. In order to prove the above theorems, we need resolution theorem and empirical process theory. In regular cases, l=n=d/2. 22 12/15/2011 Birational probability theory Outline of Proofs Posterior distribution measure Kn(w)=(1/n)S f(Xi,w) exp(-nKn(w)) j(w) dw 2k 1/2 k = exp( - nu + n u ξn(u) ) j(g(u))|g’(u)| du o o 2k h 1/2 = dt d(t-nu ) |u | b(u) exp( - t + t ξn(u) ) du 0 m-1 (log n) l-1 1/2 = l dt t exp( - t + t ξn(u) ) D(u)du n 12/15/2011 Birational probability theory 23 Outline of Proofs 2 Def. Expectation over the limit posterior distribution < > dt duD(u)( ) tl-1exp( -t+t1/2x(u)) = dt duD(u) tl-1exp( -t+t1/2x(u)) Lemma. For s ≧0, s/2 s s/2 s n Ew [ f(x,w) ] < t a(x,u) > 12/15/2011 Birational probability theory 24 Cumulants --- Random Variables g’(0) = S + (l+V1/2)/n + op(1/n) g’’(0)= - V2/n + op(1/n) t’(0) = Sn + (l-V1/2)/n + op(1/n) t’’(0) = - V2 /n + op(1/n) 12/15/2011 25 Birational probability theory Cumulants --- Birational invariants. E[ g’(0) ] = S + (l+n)/n + op(1/n) E[ g’’(0) ] = - 2n/n + op(1/n) E[ t’(0) ] = S + (l-n)/n + op(1/n) E[ t’’(0) ]= - 2n /n + op(1/n) 12/15/2011 26 Birational probability theory Theorem Generalization Loss Gn = S + [ l + V1/2 – V2/2 ] / n +op(1/n) Training Loss Tn = Sn + [ l - V1/2 - V2/2 ] / n +op(1/n) 12/15/2011 Birational probability theory 27 Theorem When n tends to infinity, E[ Gn ] = S + l / n + o(1/n), E[ Tn ] = S + ( l-2n ) / n + o(1/n), E[ V2 ] = 2n + o(1). 12/15/2011 28 Birational probability theory WAIC Def. WAIC is defined by Wn = Tn + V2/n. Theorem For arbitrary set (q(x),p(x|w),j(w)), 2 E[ Gn ] = E[ Wn ] + o(1/n ). (Gn-S) +( Wn–Sn) = 2l/n + op(1/n). Remark. WAIC is asymptotically equivalent to Bayes cross validation (Watanabe,JMLR,Vol.11,3571-3594,2010) 12/15/2011 29W Birational probability theory 0.15 Wn-Sn + G-S 0.1 Red =Wn-Sn 0.05 Theory=l/n Blue =Gn- S 0 1 8 15 22 29 36 43 50 57 64 71 78 85 92 99 100 Indep. Reduced Rank -0.05 Trials Regression 5-5-5 TrueThank 5-3- you5 -0.1 By Theory, l=12 n=200 Tn-Sn Metropolis -0.15 100000-200000 12/15/2011 Birational probability theory 30 0.14 (Gn-S)+(Wn-Sn) = 2l/n 0.12 Gn-S 0.1 0.08 0.06 0.04 0.02 Wn - Sn 0 -0.02 0 0.02 0.04 0.06 0.08 0.1 0.12 WAIC 2 (1) E[ Wn ]= E[ Gn ]+o(1/n ) holds even if q(x) is not realizable by p(x|w). (2) The essential main term is fluctuated. Inconsistency in model selection. (3) If the posterior can be approximated by a normal distribution, WAIC is equivalent to AIC and DIC as a random variable. (4) If otherwise, WAIC is unbiased estimator of generalization error, whereas either AIC or DIC not. 12/15/2011 32 Birational probability theory 4 Open Problems 12/15/2011 Birational probability theory 33 Two Biratinal Invariants If the regularity condition is satisfied, l = n = d/2. In general, they are different. l = Dimension that shows how fast the posterior shrinks. n = Dimension that shows how strong the posterior fluctuates. Q. Mathematically, what is n ? 12/15/2011 Birational probability theory 34 Generalized RLCT a g(a)= - Ex[ log Ew[p(x|w) ] ] n a t(a) = - (1/n) S log Ew[p(Xi|w) ] i=1 Q.
Details
-
File Typepdf
-
Upload Time-
-
Content LanguagesEnglish
-
Upload UserAnonymous/Not logged-in
-
File Pages37 Page
-
File Size-