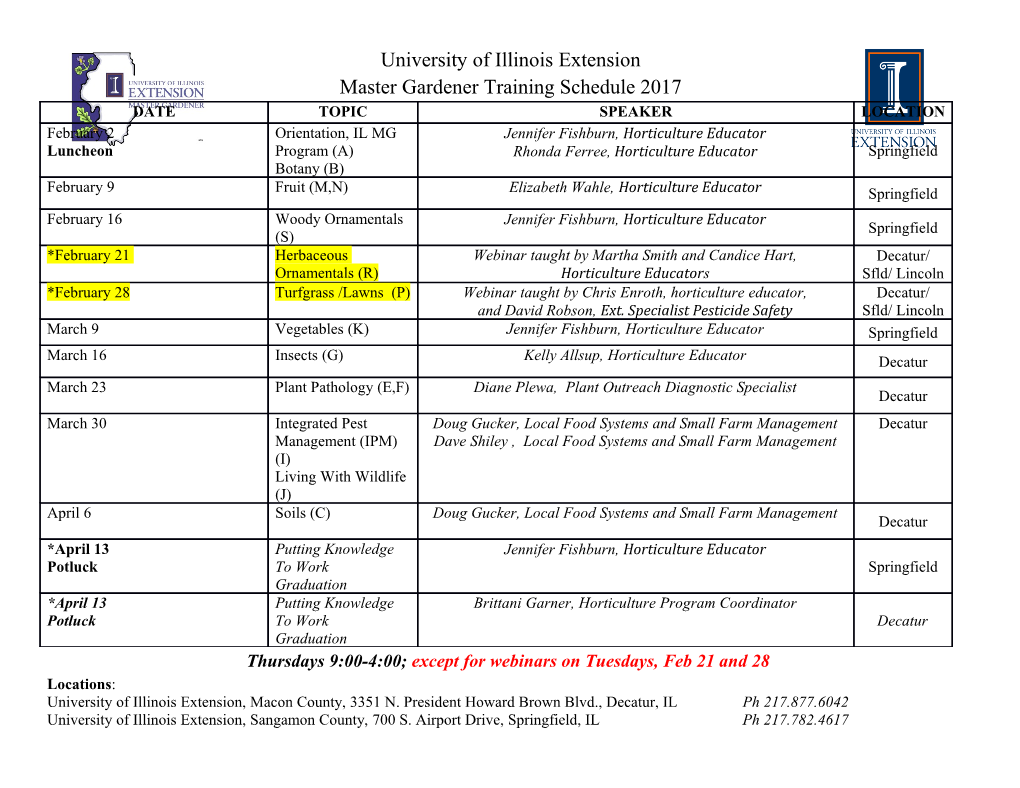
Algebraic Statistics Karl-Heinz Zimmermann Algebraic Statistics Hamburg University of Technology Prof. Dr. Karl-Heinz Zimmermann Hamburg University of Technology 21071 Hamburg Germany All rights reserved c 2009, 2015, 2016 Karl-Heinz Zimmermann, author urn:nbn:de:gbv:830-88213690 For my Teachers Thomas Beth† Adalbert Kerber Sun-Yuan Kung Horst M¨uller VI Preface Algebraic statistics brings together ideas from algebraic geometry, commutative algebra, and combina- torics to address problems in statistics and its applications. Computer algebra provides powerful tools for the study of algorithms and software. However, these tools are rarely prepared to address statistical challenges and therefore new algebraic results need often be developed. This way of interplay between algebra and statistics fertilizes both disciplines. Algebraic statistics is a relatively new branch of mathematics that developed and changed rapidly over the last ten years. The seminal work in this field was the paper of Diaconis and Sturmfels (1998) introducing the notion of Markov bases for toric statistical models and showing the connection to commutative algebra. Later on, the connection between algebra and statistics spread to a number of different areas including parametric inference, phylogenetic invariants, and algebraic tools for maximum likelihood estimation. These connection were highlighted in the celebrated book Algebraic Statistics for Computational Biology of Pachter and Sturmfels (2005) and subsequent publications. In this report, statistical models for discrete data are viewed as solutions of systems of polyno- mial equations. This allows to treat statistical models for sequence alignment, hidden Markov models, and phylogenetic tree models. These models are connected in the sense that if they are interpreted in the tropical algebra, the famous dynamic programming algorithms (Needleman-Wunsch, Viterbi, and Felsenstein) occur in a natural manner. More generally, if the models are interpreted in a higher di- mensional analogue of the tropical algebra, the polytope algebra, parametric versions of these dynamic programming algorithms can be established. Markov bases allow to sample data in a given fibre using Markov chain Monte Carlo algorithms. In this way, Markov bases provide a means to increase the sample size and make statistical tests in inferential statistics more reliable. We will calculate Markov bases using Groebner bases in commutative polynomial rings. The manuscript grew out of a lecture on algebraic statistics held for Master students of Computer Science at the Hamburg University of Technology. It appears that the first lecture held in the summer term 2008 was the first course of this kind in Germany. The current manuscript is the basis of a four-hour introductory course. The use of computer algebra systems is at the heart of the course. Maple is employed for symbolic computations, Singular for algebraic computations, and R for statistical computations. The monograph Statistical Computing with R from Maria L. Rizzo (2007) was an excellent source for implementing the R code in this book. The second and third editions are streamlined versions of the first one. Hamburg, Jan. 2016 Karl-Heinz Zimmermann Contents Part I Algebraic and Combinatorial Methods 1 Commutative Algebra ................................................... ........ 3 1.1 Polynomial Rings................................. ............................. 3 1.2 Ideals.......................................... .............................. 5 1.3 Monomial Orders.................................. ............................ 7 1.4 Division Algorithm ............................... ............................. 11 1.5 GroebnerBases ................................... ............................ 14 1.6 Computation of Groebner Bases...................... ........................... 16 1.7 Reduced Groebner Bases ............................ ........................... 19 1.8 ToricIdeals..................................... .............................. 21 2 Algebraic Geometry ................................................... .......... 25 2.1 AffineVarieties................................... ............................. 25 2.2 Ideal-Variety Correspondence ..................... .............................. 29 2.3 ZariskiTopology ................................. ............................. 34 2.4 Irreducible Affine Varieties........................ .............................. 34 2.5 Elimination Theory............................... ............................. 35 2.6 Geometry of Elimination ........................... ............................ 41 2.7 Implicit Representation .......................... .............................. 43 3 Combinatorial Geometry ................................................... ..... 49 3.1 Tropical Algebra ................................. ............................. 49 3.2 Shortest Paths Problem ............................ ............................ 50 3.3 GeometricZoo .................................... ............................ 51 3.4 Geometry of Polytopes............................. ............................ 57 3.5 Polytope Algebra................................. ............................. 64 3.6 Newton Polytopes ................................. ............................ 68 3.7 Parametric Shortest Path Problem................... ............................ 71 X Contents Part II Algebraic Statistics 4 Basic Algebraic Statistical Models ............................................... 75 4.1 Introductory Example............................. ............................. 75 4.2 General Algebraic Statistical Model................ .............................. 77 4.3 LinearModels.................................... ............................. 79 4.4 ToricModels..................................... ............................. 82 4.5 MarkovChainModel ................................ .......................... 87 4.6 Maximum Likelihood Estimation ..................... ........................... 90 4.7 Model Invariants ................................. ............................. 93 4.8 Statistical Inference............................ ................................ 96 5 Sequence Alignment ................................................... .......... 99 5.1 Sequence Alignment ............................... ............................ 99 5.2 Scoring Schemes.................................. .............................102 5.3 Pair Hidden Markov Model ........................... ..........................106 5.4 Sum-Product Decomposition........................ ............................108 5.5 Optimal Alignment ................................ ............................110 5.6 Needleman-Wunsch Algorithm ....................... ...........................111 5.7 Parametric Sequence Alignment ..................... ............................113 6 Hidden Markov Models ................................................... .......121 6.1 Fully Observed Markov Model ........................ ..........................121 6.2 Hidden Markov Model ............................... ..........................125 6.3 Sum-Product Decomposition........................ ............................127 6.4 Viterbi Algorithm ................................ .............................129 6.5 Expectation Maximization......................... .............................132 6.6 Finding CpG Islands............................... ............................135 7 Tree Markov Models................................................... ..........139 7.1 Data and General Models ............................ ..........................139 7.2 Fully Observed Tree Markov Model .................... ..........................143 7.3 Hidden Tree Markov Model........................... ..........................148 7.4 Sum-Product Decomposition........................ ............................150 7.5 Felsenstein Algorithm ............................ ..............................151 7.6 Evolutionary Models.............................. .............................153 7.7 Group-Based Evolutionary Models................... ............................160 8 Computational Statistics................................................... 175 8.1 MarkovBases..................................... ............................175 8.2 MarkovChains.................................... ............................178 8.3 Metropolis Algorithm ............................. .............................184 8.4 Contingency Tables............................... .............................189 8.5 Hardy-Weinberg Model............................. ............................198 Contents XI 8.6 Logistic Regression .............................. ..............................205 A Computational Statistics in R ................................................... 211 A.1 Descriptive Statistics........................... ................................211 A.2 Random Variables and Probability................... ............................217 A.3 Some Discrete Distributions ....................... .............................220 A.4 Some Continuous Distributions..................... .............................228 A.5 Statistics ...................................... ...............................238 A.6 MethodofMoments ................................. ..........................239 A.7 Maximum-Likelihood Estimation .................... ............................241
Details
-
File Typepdf
-
Upload Time-
-
Content LanguagesEnglish
-
Upload UserAnonymous/Not logged-in
-
File Pages296 Page
-
File Size-