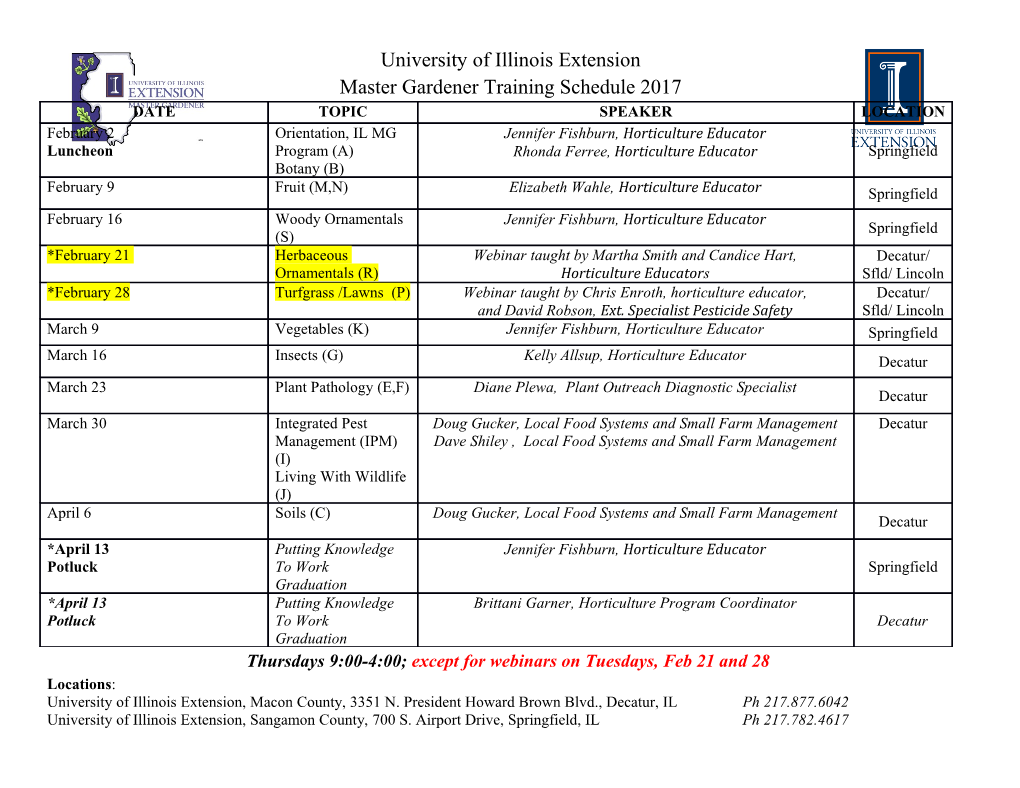
Missouri University of Science and Technology Scholars' Mine Electrical and Computer Engineering Faculty Research & Creative Works Electrical and Computer Engineering 01 Jun 2008 Differential Evolution Particle Swarm Optimization for Digital Filter Design Bipul Luitel Ganesh K. Venayagamoorthy Missouri University of Science and Technology Follow this and additional works at: https://scholarsmine.mst.edu/ele_comeng_facwork Part of the Electrical and Computer Engineering Commons Recommended Citation B. Luitel and G. K. Venayagamoorthy, "Differential Evolution Particle Swarm Optimization for Digital Filter Design," Proceedings of the IEEE Congress on Evolutionary Computation, 2008. CEC 2008. (IEEE World Congress on Computational Intelligence), Institute of Electrical and Electronics Engineers (IEEE), Jun 2008. The definitive version is available at https://doi.org/10.1109/CEC.2008.4631335 This Article - Conference proceedings is brought to you for free and open access by Scholars' Mine. It has been accepted for inclusion in Electrical and Computer Engineering Faculty Research & Creative Works by an authorized administrator of Scholars' Mine. This work is protected by U. S. Copyright Law. Unauthorized use including reproduction for redistribution requires the permission of the copyright holder. For more information, please contact [email protected]. Differential Evolution Particle Swarm Optimization for Digital Filter Design Bipul Luitel, 6WXGHQW0HPEHU ,((( and Ganesh K. Venayagamoorthy, 6HQLRU0HPEHU,((( Abstract— In this paper, swarm and evolutionary algorithms approximate the ideal filter. Since population based have been applied for the design of digital filters. Particle stochastic search methods have proven to be effective in swarm optimization (PSO) and differential evolution particle multidimensional nonlinear environment, all of the swarm optimization (DEPSO) have been used here for the constraints of filter design can be effectively taken care of design of linear phase finite impulse response (FIR) filters. Two by the use of these algorithms. different fitness functions have been studied and experimented, Previously, computational Intelligence based techniques each having its own significance. The first study considers a fitness function based on the passband and stopband ripple, such as neural networks, particle swarm optimization (PSO) while the second study considers a fitness function based on the and genetic algorithms (GA) have been implemented in the mean squared error between the actual and the ideal filter design of digital filters. One such approach using neural response. DEPSO seems to be promising tool for FIR filter networks has been described in [1]. Use of PSO and GA in design especially in a dynamic environment where filter the design of digital filters is described in [2]. Also, use of coefficients have to be adapted and fast convergence is of PSO in the design of frequency sampling finite impulse importance. response (FIR) filter has been described in [3]. Use of differential evolution in the design of digital filters has been I. INTRODUCTION implemented in Storn’s work [4], [5] and Karaboga’s work FILTER is a frequency selective circuit that allows a [6]. Design of infinite impulse response (IIR) filters using Acertain frequency to pass while attenuating the others. PSO is described in [7]. PSO with a preferential velocity Filters could be analog or digital. Analog filters use update mechanism has been explained and applied in the electronic components such as resistor, capacitor, transistor design of IIR filters in [8]. etc. to perform the filtering operations. These are mostly In this paper, swarm and evolutionary algorithms have used in communication for noise reduction, video/audio been applied for the design of digital filters. Particle swarm signal enhancement etc. In contrast, digital filters use digital processors which perform mathematical calculations on the optimization and differential evolution particle swarm sampled values of the signal in order to perform the filter optimization (DEPSO) have been used here for the design of operation. A computer or a dedicated digital signal linear phase FIR filters. processor may be used implementing digital filters. Traditionally, different techniques exist for the design of II. DIGITAL FILTER DESIGN digital filters. Of these, windowing method is the most Digital filters are classified as finite impulse response popular. In this method, ideal impulse response is multiplied (FIR) filter or infinite impulse response (IIR) filter with a window function. There are various kinds of window depending upon whether the response of the filter is functions (Butterworth, Chebyshev, Kaiser etc.), depending dependent on only the present input values or on the present on the requirements of ripples on the passband and inputs as well as previous outputs, respectively. stopband, stopband attenuation and the transition width. Any time varying signal & [ W sampled at a sampling These various windows limit the infinite length impulse interval of K has input signals [ [ [ [«««« [Q in response of ideal filter into a finite window to design an intervals K K K ««« QK. These inputs have actual response. But windowing methods do not allow corresponding outputs \\\\««««\Q depending sufficient control of the frequency response in the various upon the kind of operation performed. Thus, the order of the frequency bands and other filter parameters such as filter is determined by the number of the previous input transition width. Designer always has to compromise on one terms used to calculate the current output. The D D D or the other of the design specifications. So, evolutionary terms appearing in the following equations are called the methods have been implemented in the design of digital filter coefficients and determine the operation of the filter. filters to design with better parameter control and to better =HUR2UGHU : \Q D0 [Q (1) The funding provided by the National Science Foundation, USA under the CAREER grant ECCS #0348221 and ECCS #0625737 is gratefully acknowledged )LUVW2UGHU : \Q D0 [Q D1 [Q1 (2) Bipul Luitel and Ganesh K. Venayagamoorthy are with the Real-Time Power and Intelligent Systems Laboratory, Department of Electrical and Computer Engineering, Missouri University of Science and Technology, 6HFRQG2UGHU : \Q D0 [Q D1 [Q1 D2 [Q2 (3) Rolla, MO 65401 USA (e-mail: bl7f3@ mst.edu and [email protected]). 3954 978-1-4244-1823-7/08/$25.00c 2008 IEEE Authorized licensed use limited to: IEEE Xplore. Downloaded on December 9, 2008 at 12:45 from IEEE Xplore. Restrictions apply. III. DEPSO ALGORITHM Now FIR filter or also called the non-recursive filter can be represented by the following equation: $ 3DUWLFOH6ZDUP2SWLPL]DWLRQ Particle swarm optimization is an evolutionary algorithm developed by Eberhart and Kennedy in 1995 [9]. It is a \Q D0 [Q D1 [Q1 D2 [Q2 (4) population based search algorithm and is inspired by the Similarly, IIR filter or also called the recursive filter is observation of natural habits of bird flocking and fish schooling. In PSO, a swarm of particles moves through a ' represented as: dimensional search space. The particles in the search process are the potential solutions, which move around the defined \Q [Q \Q1 (5) search space with some velocity until the error is minimized or the solution is reached, as decided by the fitness function. By introducing a unit delay element ] , such that] [Q [ Q The particles reach to the desired solution by updating their and ] \Q \ Q , the transfer function of FIR filter can be position and velocity according to the PSO equations. In represented as: PSO model, each individual is treated as a volume-less particle in the D-dimensional space, with the position and velocity of LWK particle represented as: \Q 1 2 D0 D1] D2 ] (6) [Q ; L ([L1, [L2 ,........, [L' ) (8) and that of IIR filter as: 9L (YL1,YL2 ,........,YL' ) (9) 1 2 \Q D0 D1] D2 ] (7) 1 2 9LG Z*9LG F1 *UDQG1 ()*(3LG ; LG ) [Q E0 E1] E2 ] (10) F2 *UDQG 2 ()*(3J ; LG ) Various filter parameters which come into picture are the stopband and passband normalized frequencies (ȦVȦS), the ; LG ; LG 9LG (11) passband and stopband ripple (įS) and (įV), the stopband attenuation and the transition width. These parameters are mainly decided by the filter coefficients as is evident from These particles are randomly distributed over the search transfer functions in (6) and (7). Significance of these space with initial position and velocity. They change their parameters in actual filters with respect to ideal filter is positions and velocity according to (10) and (11) where F illustrated in Fig. 1. In any filter design problem, some of and F are cognitive and social acceleration constants, these parameters are fixed while others are determined. In UDQG and UDQG are two random functions uniformly this paper, swarm and evolutionary optimization algorithms distributed in the range of [0,1] andZ is the inertia weight are applied in order to obtain the actual filter response as introduced to accelerate the convergence speed of PSO [9]. close as possible to the ideal response. Vector 3L 3L 3L3L' is the best previous position (the position giving the best fitness value) of particle L called the pbest, and vector 3J 3J 3J 3J' is the position of the best particle among all the particles in the population and is called the th gbest. ;LG9LG3LG are the G dimension of vector of ;L9L3L. The basic pseudocode for PSO can be written as: )RUHDFKSDUWLFOH ,QLWLDOL]H3DUWLFOH
Details
-
File Typepdf
-
Upload Time-
-
Content LanguagesEnglish
-
Upload UserAnonymous/Not logged-in
-
File Pages9 Page
-
File Size-