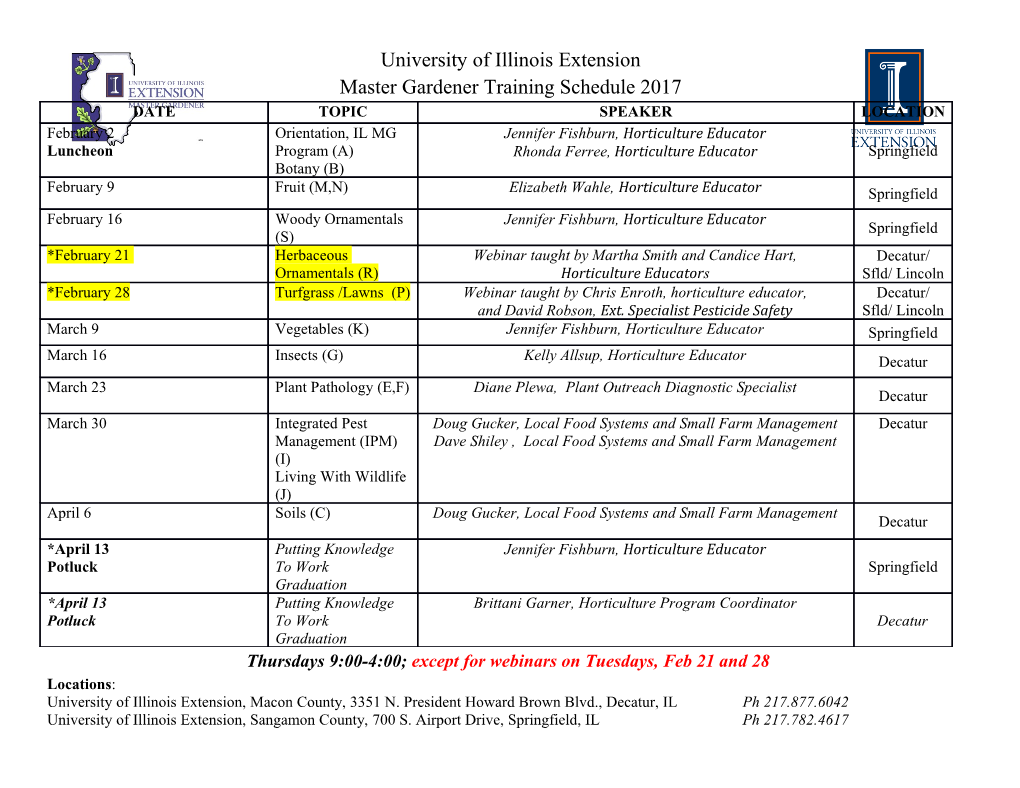
SSIIM - Seminários de Sistemas Inteligentes, Interacção e Mul8média, MIEIC Social Network Mining Eduarda Mendes Rodrigues Assistant Professor DEI-FEUP, Universidade do Porto hHp://www.fe.up.pt/~eduarda [email protected] @eduardamr Social Media Landscape Social Media Landscape • People – the individual is at the center of the social web • Social media networks – explicit and implicit social 8es – interac8on among millions of people • User-generated content – rich source of collecve knowledge – diffusion of informaon and opinions – drives social engagement Informaon Retrieval and Social Media • Proper8es of social media – Scale: millions of ac8ve users, millions of posts per day – Real-.me: breaking news, informaon novelty – Duplicates: informaon diffusion (re-tweets, cross-posts, etc.) – Content quality: spelling, grammar, punctuaon, emo8cons, etc. – Social fabric: informaon credibility, opinion leaders, topic experts • Some challenges: relevance and ranking – Social vs. non-social content – Novelty detec8on – Credibility of the informaon sources Informaon Credibility • Several newspapers picked up the fake photos • Wrongly indexed by search engines based on the news stories • Led to wider disseminaon Social Media Mining People interact through social media…" …and patterns are left behind!" Social Media Mining Can social network analysis enrich the content analysis? §! user ac8vity stas8cs §! §! interac8on paerns Social Network Content text features §! social network metrics §! topic analysis §! community detec8on Analysis! Analysis! §! clustering and classificaon §! visualizaon §! informaon extrac8on Can the content analysis help explain the social network structure and dynamics? Current Research •! Data mining and IR in social media –! social network mining –! text classificaon, opinion mining –! micro-blog search •! Network visualizaon –! layout and clustering algorithms –! design of interac8ve tools •! Data journalism –! informaon extrac8on from news –! real-8me social media analy8cs •! Social compu8ng applicaons Social Media Networks •! Explicit social es –! Friends on Facebook –! Followers on Twier –! Professional contacts on LinkedIn –! ... •! Implicit social .es –! Like, favorite, repin –! Reply, retweet, share –! Comment, review –! Tag, rate, vote –! ... Implicit Networks for Social Media Mining •! Discussion groups (usenet newsgroups) –! Can we idenfy posts with answers in Q&A groups? –! Can we predict agreement and disagreement in debate groups? •! Community Q&A –! What type of quesons are posted? –! Can we infer user intent when pos8ng a ques8on? Discussion Group Communies •! Discussion groups are extremely valuable sources of informaon •! Iden8fying the polarity of people’s opinions about certain topics is useful for business intelligence •! People seeking informaon through newsgroup search may want to be pointed at answers to their ques8ons Implicit Networks in Discussion Groups discussion thread" thread structure" social network graph" replies-to! w=2! Mining Paerns of Social Interac8on Author Networks Thread Networks • Reply-to Network: connects authors who reply to other authors • Common Authors Network: connects threads • Thread Participation Network: connects authors who co- that have common authors participate in threads • Text Similarity Network: connects threads of • Text Similarity Network: connects authors of similar content similar content Feature Sets Supervised Learning (Linear SVM) Message Categories § Agreement, Disagreement, Insult § Queson, Answer B. Fortuna, E. Mendes Rodrigues, N. Milic-Frayling. Improving the Classification of Newsgroup Messages through Social Network analysis. ACM 16th Intl. Conf. on Information and Knowledge Management, CIKM 2007 (PDF). Mining Paerns of Social Interac8on Topic Debaters Experts Reply-to network at distance 2 for the most prolific authors of talk.politics.guns (LEFT) and microsoft.public.internetexplorer.general (RIGHT) newsgroups. Analysis of CQA Communi8es Community Question-Answering (CQA)! question" 2010" 2006" 2006" answers" 2005" 2003" 2002" • CQA services aim build a large knowledge base of 2002" quesons and answers, on any topic, and make it available through search Challenge: content quality! User Intent & Ques8on Types Is the community sharing knowledge? Or socializing? Mendes Rodrigues, E., Milic-Frayling, N., Sharing Knowledge or socializing? Characterizing User Intent in Community Question Answering, Proceedings of the 2009 ACM International Conference on Information and Knowledge Management, CIKM ’09. Mining Ques8on Types •! Automac classificaon problem –! Social vs. Non-social quesons •! Feature sets –! Ques.on features Content (c.idf scores for single terms and n-grams), message length –! Thread features Responsiveness, user par8cipaon, presence of URLs in answers –! Tags and topic features Aggregate informaon about specificity of tag or topic –! Social network features for users involved in the thread Clustering coefficient, degree Social Network Structure •! Community ecosystem evolved in such a way that encouraged interac8ons of a social nature –! 84.5% of ques8on are non-social and 6.5% are social –! Over 8me, the percentage of social ques8ons and respec8ve answers and comments increased significantly •! How social are individual users? •! Social score: –! S(u) = |social| / |non-social| –! S(u) > 1 ⇒ most contribu8ons are with a social intent Social Network Structure •! Users with high degree post a large percentage of social quesons •! Users who answer and comment on social threads have dense in- neighborhoods Social Network Analysis •! Mapping and measurement of relaonships and flows between en88es that include people •! Views social relaonships in terms of network theory consis8ng of nodes and links –!node: “actor” on which relaonships act –!link: relaonship connec8ng nodes Social network graph Social Network Analysis Social network graphs can be analysed using a number of metrics including: •! cohesion of the network or sub-network measures the ease with which connecons can be made •! density of the network or sub-network measures the robustness of the connecons •! centrality of the nodes gives a rough indica/on of the social power of a node in the network -! degree -! betweenness -! closenness Social network graph Degree Centrality Count of the number of links to other nodes in the network Higher degree of a node might indicate that the node is a hub in the network Most connected does not mean most powerful! © David Ramos / GeHy Images Betweeness Centrality Number of shortest paths between each node pair that a node is on Boundary spanners that bridge between groups have high betweeness High betweenness generally indicates a powerful posi8on in the network! © John Lund Closeness Centrality Mean shortest path between a node and all other nodes in the network reachable from it Reflects the ability of a node in accessing informaon through the network Low closeness generally indicates high visibility of what’s going on in the network! © Will Ockenden Centrality Mesures and Node Roles •! Peripheral – below average centrality (C) •! Central connector – above average centrality (D) •! Broker – above average betweenness (E) Social network graph Visual Signatures of Social Roles Answerer Connector Originator •! Outward links to local •! Links from local isolates •! Links from local isolates isolates oren inward only oren inward only •! Relave absence of •! Dense, many triangles •! Sparse, few triangles triangles •! Numerous intense links •! Few intense links •! Few intense links Welser, H., Smith, M., Gleave, E. and Fisher, D. Visualizing the Signatures of Social Roles in Online Discussion Groups. Journal of Social Structure, vol. 8, 2007. Network Visualizaon Visualizaon should support knowledge discovery and communicaon How good is a network visualizaon? Ideally… •! Every node is visible •! The degree of every node can be counted •! It is possible to follow every link from source to des8naon •! Clusters and outliers are iden8fiable NetViz Nirvana!!! C. Dunne and B. Shneiderman, “Improving graph drawing readability by incorporang readability metrics: A sorware tool for network analysts,” University of Maryland, HCIL Tech Report HCIL-2009-13, May 2009. How good is a network visualizaon? Challenge: real networks are oren very complex structures. Standard layout algorithms don’t help much when the size of the network is above a few hundred nodes and the network is relavely dense in the number of links. Edges crossings and node occlusions! Interpretaon of the network structure oren requires visualizing addi8onal informaon about the nodes and links. Some Visualizaon Approaches •! Overview of the network •! Zoom and details on demand •! Dynamically filter nodes and links •! Integrate metrics and visualizaon •! Layout through seman8c substrates Network Analysis and Visualizaon Process Model Define Adjust visual Collect Choose network layout Interpret Analysis properesInterpret Network Data Goals DataData Apply data filters D. L. Hansen, D. Rotman, E. M. Bonsignore, N. Milic-Frayling, E. Mendes Rodrigues, M. Smith, and B. Shneiderman, “Do you know the way to SNA?: A process model for analyzing and visualizing social media data.” in University of Maryland Tech Report: HCIL-2009-17. Network Analysis and Visualizaon Process Model Define Collect Adjust visual Choose Interpret network layout Analysis Network properes Data Goals Data Apply data filters D. L. Hansen, D. Rotman, E. M. Bonsignore, N. Milic-Frayling, E. Mendes Rodrigues, M. Smith, and B. Shneiderman, “Do
Details
-
File Typepdf
-
Upload Time-
-
Content LanguagesEnglish
-
Upload UserAnonymous/Not logged-in
-
File Pages52 Page
-
File Size-