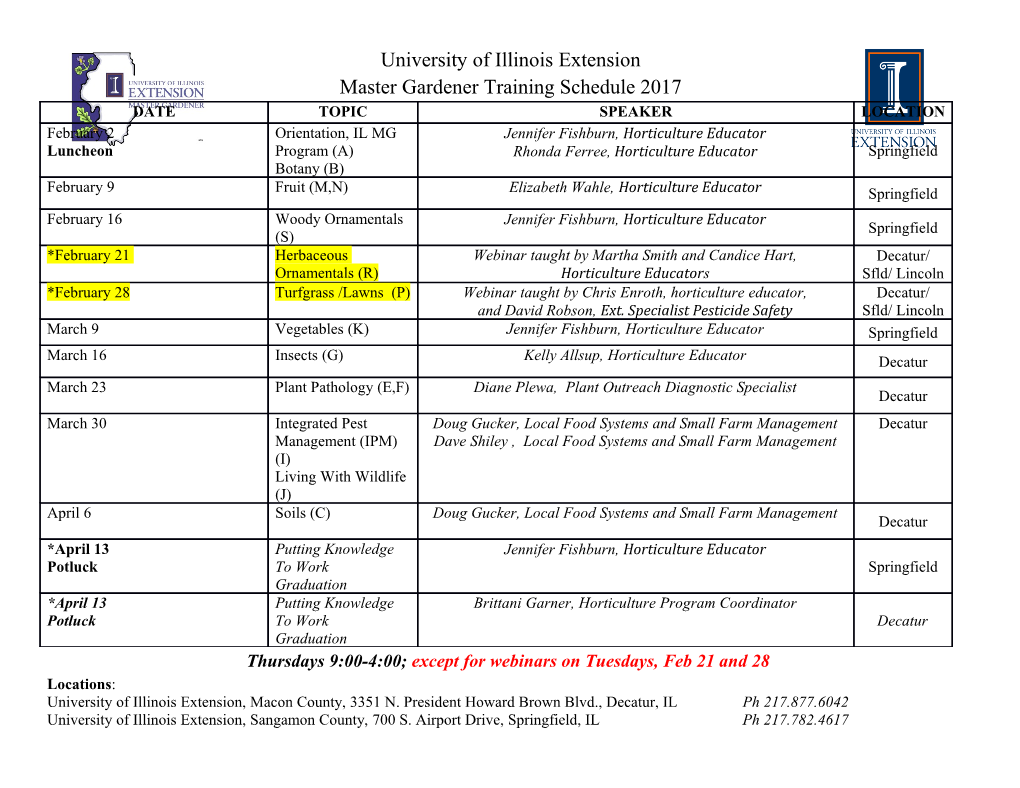
The Moran Process as a Markov Chain on Leaf-labeled Trees David J. Aldous∗ University of California Department of Statistics 367 Evans Hall # 3860 Berkeley CA 94720-3860 [email protected] http://www.stat.berkeley.edu/users/aldous March 29, 1999 Abstract The Moran process in population genetics may be reinterpreted as a Markov chain on a set of trees with leaves labeled by [n]. It provides an interesting worked example in the theory of mixing times and coupling from the past for Markov chains. Mixing times are shown to be of order n2, as anticipated by the theory surrounding Kingman’s coalescent. Incomplete draft -- do not circulate AMS 1991 subject classification: 05C05,60C05,60J10 Key words and phrases. Coupling from the past, Markov chain, mixing time, phylogenetic tree. ∗Research supported by N.S.F. Grant DMS96-22859 1 1 Introduction The study of mixing times for Markov chains on combinatorial sets has attracted considerable interest over the last ten years [3, 5, 7, 14, 16, 18]. This paper provides another worked example. We must at the outset admit that the mathematics is fairly straightforward, but we do find the example instructive. Its analysis provides a simple but not quite obvious illustration of coupling from the past, reminiscent of the elementary analysis ([1] section 4) of riffle shuffle, and of the analysis of move-to-front list algorithms [12] and move-to-root algorithms for maintaining search trees [9]. The main result, Proposition 2, implies that while most combinatorial chains exhibit the cut-off phenomenon [8], this particular example has the opposite diffusive behavior. Our precise motivation for studying this model was as a simpler version of certain Markov chains on phylogenetic trees: see section 3(b) for further discussion. The model also fits a general framework studied in [4]: see section 3(a). 1.1 The Moran chain The Moran model ([11] section 3.3) in population genetics models a popu- lation of constant size n. At each step, one randomly-chosen individual is killed and another randomly-chosen individual gives birth to a child. The feature of interest is the genealogy of the individuals alive at a given time, that is how they are related to each other by descent. In population genetics these “individuals” are in fact genes and there is also mutation and selection structure, but our interest goes in a different direction. There is some flexibility in how much information we choose to record in the genealogy of the current population, and we will make the choice that seems simplest from the combinatorial viewpoint. Label the individuals as [n] := {1, 2, . , n}, and declare that if individual k is killed then the new child is given label k. The left diagram in figure 1 shows a possible genealogy, in which we keep track of the order of the times at which all the splits in all the lines of descent occured, but not the absolute times of splits. 2 level 0 t t = f7(t, 4, 7) 7 6 ¨H ¨H ¨¨ HH ¨¨ HH 5 ¨ H ¨ H @ QQ A 4 @ Q A ¡A ¡@ @ 3 ¡ A ¡ @ @ ¡@ ¡@ ¡A 2 ¡ @ ¡ @ ¡ A ¡A ¡A ¡@ 1 ¡ A ¡ A @ ¡A ¡A ¡A 0 ¡ A ¡ A ¡ A 6 4 3 7 1 4 2 6 4 3 1 4 2 6 4 7 3 1 4 2 Figure 1. A transition t → t0 in the Moran chain. Precisely, the left diagram shows a tree t with leaf-set [n] and height n, where at each level one downward edge splits into two downward edges, and where we distinguish between left and right branches. Such a tree has n(n + 1)/2 edges of unit length. Write Tn for the set of such trees. The cardinality of this set is #Tn = n!(n − 1)! (1) We leave to the reader the (very easy) task of giving a bijective proof of (1); an inductive proof will fall out of the proof of Lemma 1 below. Interpreting the Moran model as a Tn-valued process gives a Markov chain on Tn which we call the Moran chain. Here is a careful definition. Take a tree t ∈ Tn and distinct ordered (j, k) from [n]. Delete leaf k from t, then insert leaf k into the edge incident at leaf j, placing it to the right of leaf j. This gives a new tree 0 t = fn(t, j, k). (2) Such a transition is illustrated in figure 1. Starting from the tree t in the left diagram, leaf 7 is deleted and the levels adjusted where necessary, to give the center diagram; then leaf 7 is inserted to the right of leaf 4, and levels adjusted, to give the tree t0 in the right diagram. The Moran chain is now defined to be the chain with transition probabilities 0 0 p(t, t ) = P (fn(t, J, K) = t ) where (J, K) is a uniform random distinct ordered pair from [n]. It is easy to check this defines an aperodic irreducible chain, which there- fore has some limiting stationary distribution. Our choice of Tn as the precise state-space was motivated by 3 Lemma 1 The stationary distribution of the Moran chain is the uniform distribution on Tn. Proof. From a tree t there are n × (n − 1) equally likely choices of (k, j) which define the possible transitions t → t0. To prove the lemma we need to show the chain is doubly stochastic, i.e. that for each tree t0 there are n(n − 1) such choices which get to t0 from some starting trees t. For a tree t0 (illustrated by the right diagram in figure 1) there is only one leaf k (leaf 7, in figure 1) which might have been inserted last, into a diagram like the tree t00 in the center diagram. The trees t such that deleting leaf k from t gives t00 are exactly the trees obtainable by attaching leaf k to any of the (n−1)n/2 edges of t00 and to the right or the left of the existing edge, giving a total of (n − 1)n/2 × 2 choices, as required. Remark. The final part of the argument says that the general element t ∈ Tn may be constructed from a tree in Tn−1 by attaching leaf n to one of the (n − 1)n/2 edges, to the right or the left of the existing edge. So #Tn = n(n − 1) #Tn−1, establishing (1) by induction. 1.2 Mixing time for the Moran chain Write (Xn(m), m = 0, 1, 2,...) for the Moran chain on Tn. One of the standard ways of studying mixing is via the maximal variation distance 1 X 0 0 dn(m) := max P (Xn(m) = t |Xn(0) = t) − πn(t ) (3) t∈T 2 n t0 0 where πn(t ) = 1/#Tn is the stationary probability. Our result is most easily expressed in terms of the following random variables. Let (ξi, 2 ≤ i < ∞) be 1 independent, and let ξi have the exponential distribution with mean i(i−1) ; then let ∞ X L = ξi. (4) i=2 2 Proposition 2 (a) lim supn dn(zn ) ≤ P (L > z) < 1 for each 0 < z < ∞ 2 (b) lim infn dn(zn ) ≥ φ(z), for some φ(z) ↑ 1 as z ↓ 0. Thus mixing time (as measured by variation distance) for the Moran chain is order n2 but variation distance does not exhibit the cut-off phenomenon of [8] which usually occurs with Markov chains on combinatorial sets. As briefly sketched in the next section, what’s really going on is that 2 dn(zn ) → d∞(z) 4 where d∞(·) is the maximal variation distance associated with a certain limit continuous-time continuous-space Markov process. We might call this dif- fusive behavior, from the case of simple random walks on the k-dimensional integers modulo n, whose n → ∞ limit behavior is of this form with the limit process being Brownian motion on [0, 1]k. 1.3 Remarks on a limit process We now outline why Proposition 2 is not unexpected. In the original Moran model for a population, one can look back from the present to determine the number of steps Ln back until the last common ancestor of the present pop- −2 ulation. It is standard (see section 2.3) that n Ln converges in distribution to L. Loosely speaking, this implies that the genealogy after n2L steps can- not depend on the initial genealogy, and Proposition 2(a) is a formalization of that idea. A more elaborate picture is given by the theory surrounding Kingman’s coalescent [13, 17], which portrays the rescaled n → ∞ limit of the genealogy of the current size-n population as a genealogy C of an infinite population. Informally, what’s really going on is that 2 dn(zn ) → d∞(z), 0 < z < ∞ where d∞(·) is the maximal variation distance associated with a certain continuous-time Markov process (Ct, 0 ≤ t < ∞) whose state space is a set of possible genealogies for an infinite population. However, defining the process (Ct) precisely and proving mixing time bounds via this weak convergence methodology is technically hard. It turns out to be fairly simple to give an analysis of Proposition 2 directly in the discrete setting, by combining the standard analysis of Ln with a coupling construction, so that is what we shall do. 2 Proof of Proposition 2 2.1 Coupling from the past The proof is based on a standard elementary idea. Suppose a Markov chain (X(s)) can be represented in the form X(s + 1) = f(X(s),Us+1) for some function f, where the (Us) are independent with common distribu- tion µ.
Details
-
File Typepdf
-
Upload Time-
-
Content LanguagesEnglish
-
Upload UserAnonymous/Not logged-in
-
File Pages10 Page
-
File Size-