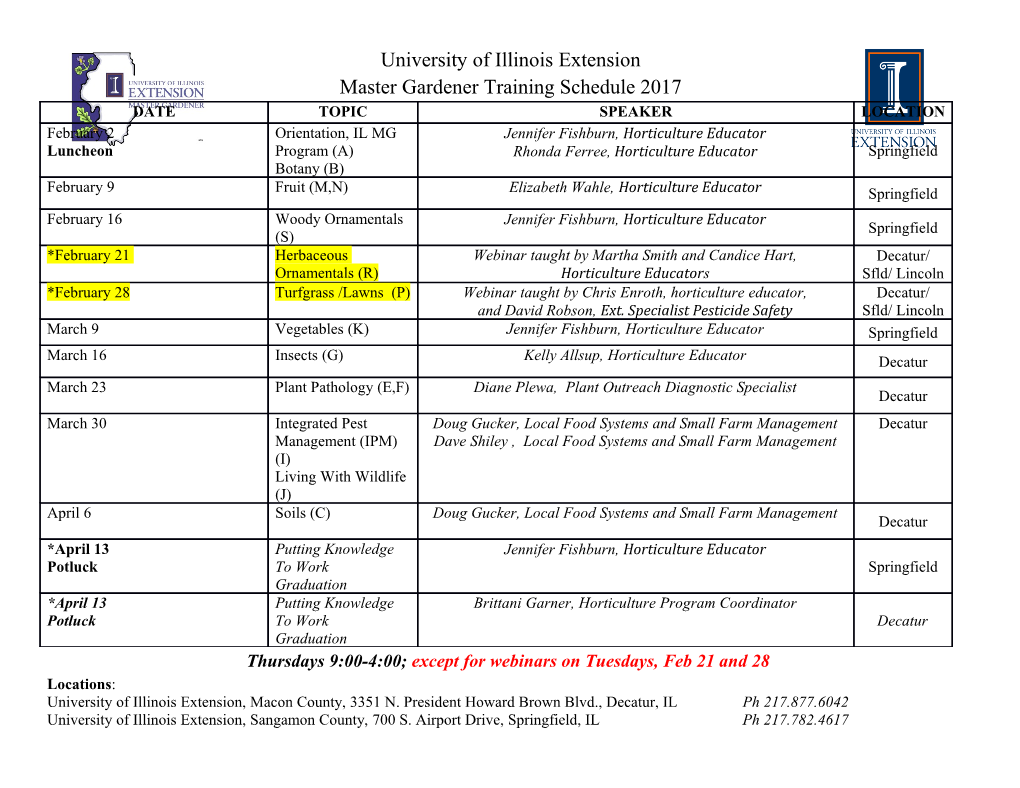
Available online at www.sciencedirect.com ScienceDirect Aquatic Procedia 4 ( 2015 ) 957 – 963 INTERNATIONAL CONFERENCE ON WATER RESOURCES, COASTAL AND OCEAN ENGINEERING (ICWRCOE 2015) Regression Analysis of Annual Maximum Daily Rainfall and Stream Flow for Flood Forecasting in Vellar River Basin P.Supriyaa*, M.Krishnavenia, M.Subbulakshmia a Centre for Water Resources, Anna University, Chennai-600025, India Abstract Flood is a natural demolishing phenomenon, forecast of which is of high importance, to reduce its impact. On the other hand, it also serves as a major source of water when conserved through proper means. For this purpose, estimation of flood is of at utmost importance. The present study employed regression analysis between weighted maximum rainfall and maximum stream flow for the flood events of respective catchments of Vellar river basin. Regression equation was framed between Annual Maximum Daily Rainfall, Stream Flow and area for each catchment. Based on the equation, flood prioritization rank was given to each of the catchment. Thereby, Lower Vellar is prioritized as first rank and Upper Vellar is prioritized as last rank respectively. This study reveals that the Lower Vellar is the most vulnerable catchment and which needs flood control measures. © 20152015 TheThe Authors. Authors. Published Published by by Elsevier Elsevier B.V. B.V. This is an open access article under the CC BY-NC-ND license Peer(http://creativecommons.org/licenses/by-nc-nd/4.0/-review under responsibility of organizing committee). of ICWRCOE 2015. Peer-review under responsibility of organizing committee of ICWRCOE 2015 Keywords: Flood Forecasting; Regression; Prioritization; Vulnerable * Corresponding author. E-mail address: [email protected] 2214-241X © 2015 The Authors. Published by Elsevier B.V. This is an open access article under the CC BY-NC-ND license (http://creativecommons.org/licenses/by-nc-nd/4.0/). Peer-review under responsibility of organizing committee of ICWRCOE 2015 doi: 10.1016/j.aqpro.2015.02.120 958 P. Supriya et al. / Aquatic Procedia 4 ( 2015 ) 957 – 963 1. Introduction The Vellar River basin was located between Ponnaiyar and Cauvery basins. The river originates in the Chitteri hills of the Eastern Ghats in the Salem district of Tamil Nadu. The river flows for a total length of 210 km in the Salem and Cuddalore districts and out falls in to the Bay of Bengal near Parangipettai. Every year, Vellar river basin is facing serious seasonal flood problems during the months of November and December. In 2009, heavy flood was occurred in both Vellar and Manimuktha river due to unforeseen precipitation and discharged 207000 Cusecs near the confluence point at Kudalayathur village. The flood water enters into the adjacent cultivable lands and causes serious threat to the livelihood of the local village people (Needhidasan. S. et al. 2013). Regression analysis performs an exploration of relationships between one dependent and one or more independent variables under consideration. Regression methods are widely used for many hydrological applications. Tangborn and Ramussen (1976) derived a regression equation based upon the relationship between winter precipitation and annual runoff. The regression coefficients were estimated with the help of mass balance equations. The accuracy of the model framed using the full record of precipitation and annual runoff by the standard error of estimate. MA Diaz-Granados and Rafael L. Bras (1982) investigated the computer based regression techniques such as ordinary least squares (OLS) and iterative Generalised Least Squares (GLS) to perform parameter estimation and residual analysis for seasonal stream flow. The residual statistics are calculated using regression equations. The results revealed that the iterative GLS performs better than the OLS in forecasting based upon the value of mean square of error. Huynh Ngoc Phien et al. (1990) compared five regression methods such as ordinary least squares, ridge, principle components, stepwise and least absolute value regression. The results revealed that the ordinary least squares, ridge, principle components regression methods are efficient in forecasting compared to the stepwise and least absolute value regression methods. Lynne Tolland (1998) discusses about the available empirical methods for the computation of peak flows in forest hydrology utilizing urban hydrology techniques. The rational methods and empirical methods are to be improvised to determine design floods for forests. Waltemeyer (2002) analyzed the magnitude and frequency of the 4-day annual low flow and regression equations for estimating the 4-day, 3-year low-flow frequency at ungauged sites on unregulated streams in New Mexico. Two regression equations were developed for estimating the 4-day, 3-year (4Q3) low-flow frequency at ungaged sites on unregulated streams in New Mexico. The first, a statewide equation for estimating the 4Q3 low- flow frequency from drainage area and average basin mean winter precipitation, was developed from the data for 50 stream flow-gaging stations that had non-zero 4Q3 low-flow frequency. The 4Q3 low-flow frequency for the 50 gaging stations ranged from 0.08 to 18.7 cubic feet per second. For this statewide equation, the average standard error of estimate was 126 percent and the coefficient of determination was 0.48. The second, an equation for estimating the 4Q3 low-flow frequency in mountainous regions from drainage area, average basin mean winter precipitation, and average basin slope, was developed from the data for 40 gaging stations located above 7,500 feet in elevation. For this regression equation, the average standard error of estimate was 94 percent and the coefficient of determination was 0.66. Bhatt. V. K (2008) developed regression equation of mean annual peak discharge for 5 & 10 return periods with channel cross-section area as independent variable provides a greater correlation coefficient and adequate flood estimates. Roland and Stuckey (2008) developed regression equations for estimating flood flows at selected recurrence intervals for ungaged streams in Pennsylvania. These equations were developed utilizing peak-flow data from 322 stream flow-gaging stations within Pennsylvania and surrounding states. All stations used in the development of the equations had 10 or more years of record and included active and discontinued continuous-record as well as crest- stage partial-record stations. The state was divided into four regions, and regional regression equations were developed to estimate the 2-, 5-, 10-, 50-, 100-, and 500-year recurrence-interval flood flows. The equations were developed by means of a regression analysis that utilized basin characteristics and flow data associated with the stations. Significant explanatory variables at the 95-percent confidence level for one or more regression equations included the following basin characteristics: drainage area; mean basin elevation; and the percentages of carbonate bedrock, urban area, and storage within a basin. The regression equations can be used to predict the magnitude of flood flows for specified recurrence intervals for most streams in the state; however, they are not valid for streams with drainage areas generally greater than 2000 square miles or with substantial regulation, diversion, or mining activity within the basin. P. Supriya et al. / Aquatic Procedia 4 ( 2015 ) 957 – 963 959 K. Engeland (2009) estimated low flow index in ungauged catchments using regional regression models and (HBV) regional hydrological model. The regression models provided the better estimates of lowest discharge values compared to HBV method. Saeid Eslamian (2010) attempted to estimate the low flow index using Principle Component Regression (PCR) based upon physiographic and hydrologic variables. The yearly minimum discharge data of respective gauging stations are ranked assessed upon its fitness distribution. M. Taylor (2011) made a comparison between Quantile Regression Technique (QRT) and Parameter Regression Technique (PRT) of regression based Regional Flood Frequency Analysis techniques. In QRT, the individual flood quantiles are regressed against catchment characteristics. In PRT, the parameters of a probability distribution are regressed against catchment characteristics. An ordinary least squares regression method is used to develop prediction equations. The reasonable predictions are achieved using the equations produced by both the methods are easily applied between catchment area and rainfall intensity. Jianzhu Li et al. (2013) performed multi linear regression analysis with flood volume and flood peak as dependent variables and changes in rainfall depth, intensity, land use area, watershed area as independent variables. The quantified effect on flood peak and flood volume was accomplished using four regression equations. Nomenclature AMDR Annual Maximum Daily Rainfall AMDSF Annual Maximum Daily Stream Flow ANFIS Adaptive Network-Based Fuzzy Inference System ANN Artificial Neural Network GLS Generalised Least Squares HBV Regional Hydrological Model MLR Multiple Regression Model MNLR Multiple Non-Linear Regression Model OLS Ordinary Least Squares PCR Principle Component Regression PRT Parameter Regression Technique PWD Public Works Department QRT Quantile Regression Technique WRD Water Resources Department X1 Annual Maximum Daily Rainfall in mm 2 X2 Catchment Area in km Y Annual Maximum Daily Stream flow in Cusecs 2. Purpose and Scope This paper presents regression equations
Details
-
File Typepdf
-
Upload Time-
-
Content LanguagesEnglish
-
Upload UserAnonymous/Not logged-in
-
File Pages7 Page
-
File Size-