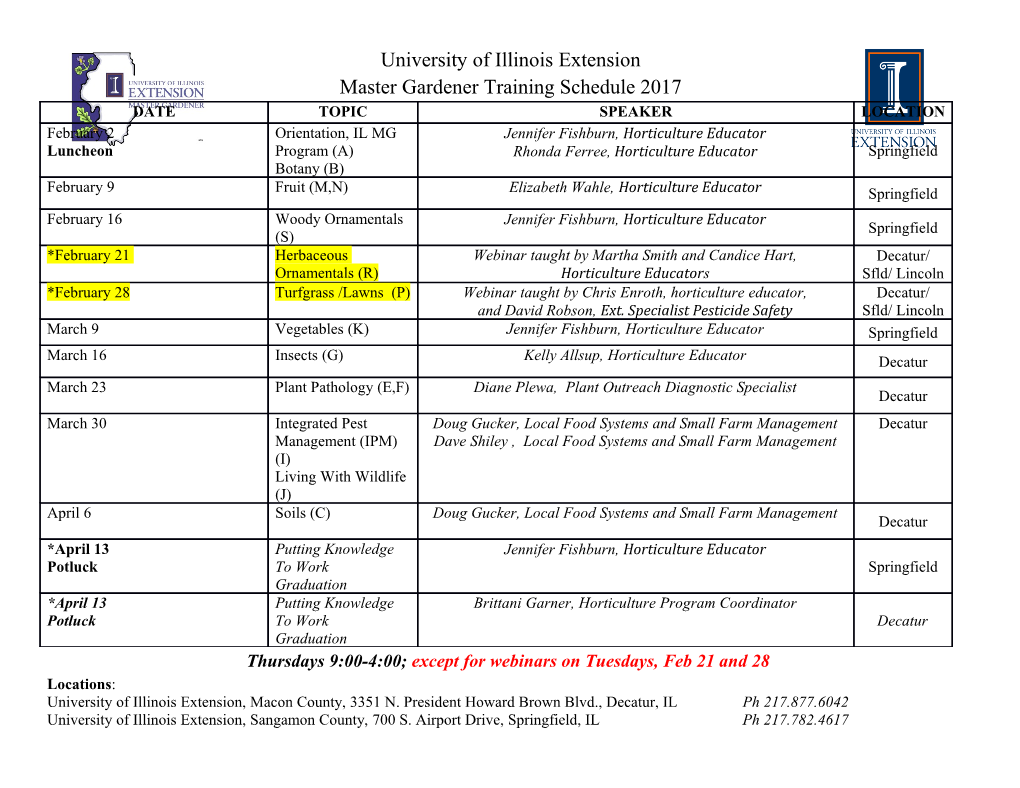
IMPROVED HARRIS’ ALGORITHM FOR CORNER AND EDGE DETEC- TIONS Soo-Chang Pei, Jian-Jiun Ding, Department of Electrical Engineering, National Taiwan University, Taipei, Taiwan, R.O.C Email address: [email protected], [email protected] ABSTRACT However, the algorithm has some problems, such as the A more accurate algorithm for corner and edge detections ability to distinguish the corner from the peak or the dip that is the improved form of the well-known Harris’ algo- and the robustness to noise should be improved. In [5], an rithm is introduced. First, instead of approximating |L[m+x, improved algorithm based on modifying the detection n+y]–L[m, n]|2 just in terms of x2, xy, and y2, we will ap- criterion was proposed. In this paper, we apply many new proximate |L[m+x, n+y]–L[m, n]|(L[m+x, n+y]–L[m, n]) by ideas listed in Section 2 to improve Harris’ algorithm for the linear combination of x2, xy, y2, x, y, and 1. With the corner detection. modifications, we can observe the sign of variation. It can avoid misjudging the pixel at a dot or on a ridge as a cor- 2. IDEAS FOR IMPROVING PERFORMANCE ner and is also helpful for increasing the robustness to (A) Instead of approximating |L[m+x, n+y]L[m, n]|2, we noise. Moreover, we also use orthogonal polynomial ex- use a quadratic polynomial to approximate L [m, n, x, y]: pansion and table looking up and define the cornity as the 1 L [m, n, x, y] “integration” of the quadratic function to further improve 1 the performance. From simulations, our algorithm can = L>@>@m x, n y L m, n (L>@>@m x, n y L m, n ) . (5) much reduce the probability of regarding a non-corner This modification is helpful for improving the robust- pixel as a corner. In addition, our algorithm is also effec- ness to noise. Suppose that the image is interfered by a tive for edge detection. noise K[m, n], i.e., H[m, n] = L[m, n] + K[m, n], and E[K[m, n]] = 0, E[K[m, n]K[m+x, n+y]] = Wx,,y, (6) Index terms: corner detection, edge detection, prob{K[m, n] = k} = prob{K[m, n] = k}, (7) quadratic polynomial, ridge detection, noise immunity then we can prove that 2 E{ H>@>@m x, n y H m, n } 1. INTRODUCTION 2 L>@>@m x, n y L m, n 2(W 0,0 W x, y ) , (8) Corner detection is important for feature extraction and pattern recognition. In [1], Harris and Stephens proposed a E{ H>@>@m x, n y H m, n (H>@>@m x, n y H m, n )} corner detection algorithm. First, they used a quadratic L>@>@m x, n y L m, n (L>@>@m x, n y L m, n ) . (9) polynomial to approximate the variation around [m, n]: Thus, the mean of (1) is affected by noise, but from (9) 2 L>@>@m x, n y L m, n the mean of L1[m, n, x, y] will be not affected by noise. The sign of variation is also important to distinguish A x 2 2C x y B y 2 + remained terms, (1) m,n m,n m,n a corner from a peak. For both a corner pixel and a peak, where Am,n, Bm,n, and Cm,n were calculated from the corre- the variations along all the directions are large, as in Fig. lations between the variations and a window function: 1. If we observe only the amplitude of variations, as Har- 2 2 Am,n X w , Bm,n Y w , Cm,n XY w , (2) ris’ algorithm, they are hard to distinguish. In contrast, if T the sign of variations can be observed, we can distin- X L>@>m, n 1, 0,1 @, Y L>@>m, n 1, 0,1 @, guish them. For a peak, the signs of variations along all 2 2 2 wx,y = exp[(x +y )/2V ]. (3) the directions are negative. For a corner, sometimes Then the variations along the principal axes can be calcu- variation is positive and sometimes it is negative. lated from the eigenvalues of the following 2u2 matrix: (B) We will approximate the variation by the linear com- bination of x2, xy, y2, x, y, and 1, see (16). For Harris’ al- ªAm,n Cm,n º H . (4) gorithm in (1), only the terms of x2, xy, and y2 are used. m,n «C B » ¬ m,n m,n ¼ Note that x2 and y2 are even-even bases (i.e., even re- If both the two eigenvalues of Hm,n are large, then we rec- spect to both x and y) and xy is an odd-odd basis. Using ognize the pixel [m, n] as a corner. Harris and Stephens them is hard to observe even-odd and odd-even varia- proposed a systematic and effective way to detect corners. tions. Thus, to observe all types of variations, we should 1-4244-1437-7/07/$20.00 ©2007 IEEE III - 57 ICIP 2007 use the terms of x (an odd-even basis) and y (an even- odd basis). With these terms, we can observe more Corner Edge + Xm,n(n) +Xm,n(n) styles of variations and classify the pixel into more types. Ym,n(p) higher gray higher gray (C) Instead of (3), we use “orthogonal polynomial expan- Ym,n(~) level re- level re- sion” to find the coefficients. It can assure that the mean i i +Ym,n(~) square error of approximation to be minimal [2]. [m, n] lower gray [m, n] (D) In [1], Harris classified the pixel into only three types: level region lower gray level re- corners, edges, and plains. In this paper, with the help of Xm,n(p) +Ym,n(p) i Case table (see Table 1), we classify the pixel into cor- Xm,n(p) ners, edges, ridges, valleys, peaks, saddles, and plains. Peak Ridge + X m , n (~) Ym,n(p) +X (p) (E) From derivations and experiments, we find that, to m,n Y (p) lower gray achieve the best accuracy, it is better to define the cornity lower gray m,n lower gray level re- [ m , n ] level re- as the “integration” of the quadratic function. See Step 6. level re- i i Xm,n(p) i +Ym,n(p) 3. ALGORITHM Xm,n(~) +Ym,n(p) (Step 1) First, find the orthonormal polynomial set that is Fig. 1 Variations of gray levels along four principal directions orthogonal respect to a weighting function w[m, n]. That is, for the pixel at a corner, on an edge, at a peak, and on a ridge. 2 2 M k >@x, y a1,k a2,k x a3,k y a4,k x a5,k xy a6,k y , Table 1 Case table. for k = 1, 2, 3, 4, 5, 6, (10) v1, v2 (p, p)(p, ~) (p, n) (~, ~) (~, n) (n, n) v , v and the parameters aj,k should be chosen properly such that 3 4 (~, p) (n, p) (~, n) . (11) (p, p) Q1 C C E1 E1 Q3 ¦¦M k >@>@>@x, y M h x, y w x, y G k ,h xy (p, ~) (~, p) C E E P CY E2 We can use the Gram-Schmidt algorithm to find aj,k. For (p, n) (n, p) C E E E E C example, if we choose the weighting function as (~, ~) E1 P E P P E2 w[x, y] exp[(x 2 y 2 ) / 20] for (x2 + y2)1/2 < 6.5, (12) E C E P E C 2 2 1/2 (~, n) (n, ~) 1 Y w[x, y] = 0 for (x + y ) > 6.5, (13) (n, n) S E2 C E2 C Q2 then the orthogonal polynomials are C: corner, E: edge, E1: ridge, E2: valley, M1>@x, y 0.1339 , M2 >@x, y 0.0498x , M3 >@x, y 0.0498y , Q1: peak Q2: dip, Q3: saddle, CY: Y-corner, P: plain. M >@x, y 0.0157y 0.1131, M >@x, y 0.0206xy , 4 5 (Step 4) From (19), we can observe the variation along the 2 2 M6 >@x, y 0.0158y 0.00213x 0.1296 . (14) two principal axes. For example, the variation along the (Step 2) Then, we do the inner product for L1[m, n, x, y] is positive part of Xm,n-axis (denoted by +Xm,n) is: t (defined in (5)) and Mk[x, y], where k = 1, 2, 3, 4, 5, 6: v (c X 2 c X )dX 1 ³0 1,m,n m,n 3,m,n m,n m,n bk,m,n M k >@>x, y L1 m, n, x, y @>@w x, y , (15) ¦¦ 3 2 xy c1,m,nt / 3 c3,m,nt / 2 . (20) (Step 3) Then we express the variation around [m, n] by: Similarly, the variations along the negative part of Xm,n- 6 axis (denoted by –Xm,n) and along the positive and nega- Qm,n >@x, y bk,m,nMk >@x, y ¦ tive parts of Ym,n-axis (denoted by +Ym,n and –Ym,n) are: k 1 2 2 for X : v c t3 / 3 c t 2 / 2 . (21) = p1,m,nx +p2,m,nxy +p3,m,ny +p4,m,nx +p5,m,ny +p6,m,n. (16) m,n 2 1,m,n 3,m,n Note that Qm,n[x, y] | L1[m, n, x, y] (defined in (5)).
Details
-
File Typepdf
-
Upload Time-
-
Content LanguagesEnglish
-
Upload UserAnonymous/Not logged-in
-
File Pages4 Page
-
File Size-