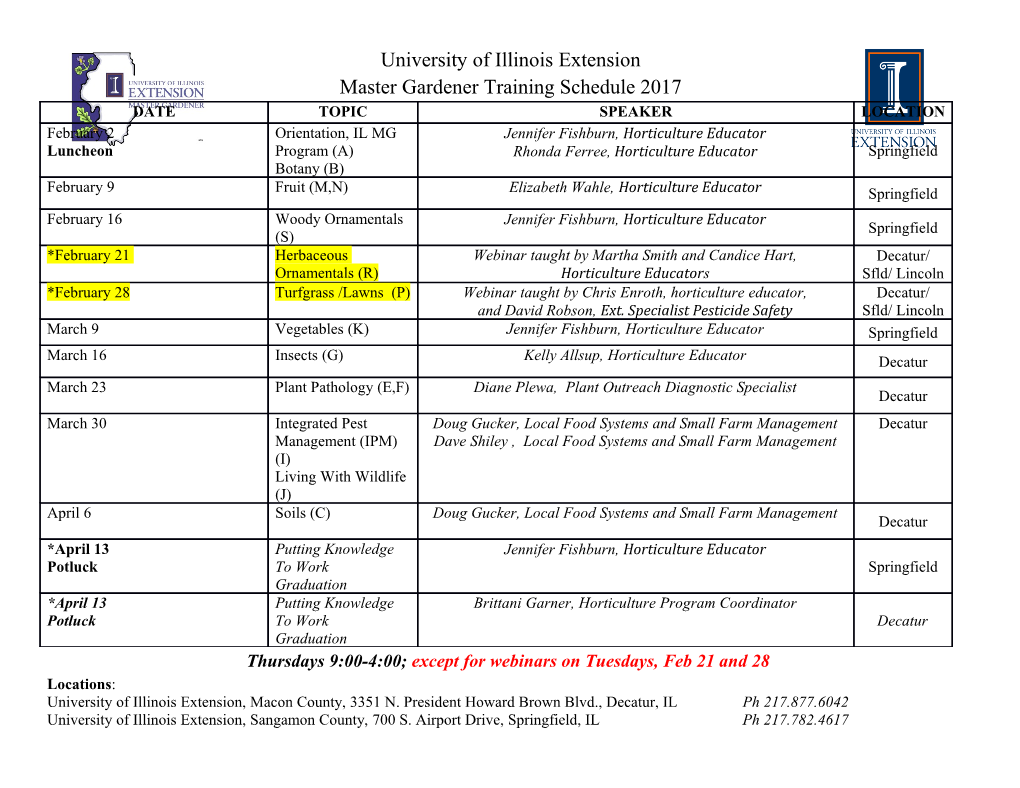
Proceedings of the 50th Hawaii International Conference on System Sciences | 2017 Collaborative Software Performance Engineering for Enterprise Applications Hendrik Müller, Sascha Bosse, Markus Wirth, Klaus Turowski Faculty of Computer Science, Otto-von-Guericke-University, Magdeburg, Germany {hendrik.mueller, sascha.bosse, markus.wirth, klaus.turowski}@ovgu.de Abstract address these challenges from a software engineering perspective under the term DevOps [2], [3]. In the domain of enterprise applications, Corresponding research activities aim at increased organizations usually implement third-party standard flexibility through shorter release cycles in order to software components in order to save costs. Hence, support frequently changing business processes. application performance monitoring activities Therefore, DevOps enables a culture, practices and constantly produce log entries that are comparable to automation that support fast, efficient and reliable a certain extent, holding the potential for valuable software delivery [4]. However, especially for collaboration across organizational borders. Taking enterprise applications, IT departments usually make advantage of this fact, we propose a collaborative use of existing standard software components instead knowledge base, aimed to support decisions of of developing solutions entirely in-house [5]. In such performance engineering activities, carried out cases, activities referred to as Dev, rather include during early design phases of planned enterprise requirements engineering, architectural design, applications. To verify our assumption of cross- customization, testing, performance-tuning and organizational comparability, machine learning deployment [6]. Here, key service levels typically algorithms were trained on monitoring logs of 18,927 include performance objectives, expressed in terms of standard application instances productively running average response times, throughput or latency, in at different organizations around the globe. Using order to ensure deployment options that are aligned random forests, we were able to predict the mean with actual business requirements. Since enterprise response time for selected standard business applications support vital corporate business transactions with a mean relative error of 23.19 functions, their performance is critical for success of percent. Hence, the approach combines benefits of business tasks and must never be degraded existing measurement-based and model-based significantly [7]. As corporations tend to grow performance prediction techniques, leading to depending on an increased customer base, new competitive advantages, enabled by inter- product releases, new divisions and acquisitions [6], organizational collaboration. enterprise application performance needs to be monitored and managed continuously and proactively [8]. In order to evaluate and test software performance in early design stages, development 1. Introduction teams carry out software performance engineering (SPE) activities [9]. In addition, operations teams As of today, development and operations conduct application performance monitoring (APM) activities are not integrated tightly enough within one [10] to control the status of running systems. In a organization [1] and in most cases do not collaborate recent technical report, [1] summarizes that the across organizations, although similar and challenges of these two performance domains are comparable standard software components are often considered independently from each other. consistently deployed. The lack of integration and the Hence, they identified the interoperability between distribution of responsibilities across organizational tools and techniques of SPE and APM as a key units lead to increasing difficulties in accessing success factor in order to support a level of technical information that is required to deploy, operate and and organizational flexibility that is required for maintain applications cost-effectively and in constant DevOps. According to this review, performance data alignment to agreed service levels [1]. As a collected during operations provides various insights consequence, the end-user experience may suffer, for development teams and replaces assumptions e.g., from poor software performance caused by with knowledge. However, managing performance at inadequate capacity planning. Recent approaches design-time (SPE) or at run-time (APM) leads to URI: http://hdl.handle.net/10125/41196 ISBN: 978-0-9981331-0-2 CC-BY-NC-ND 391 different challenges since, for instance, more degrees than 18,000 productively running application of freedom exist at design-time for the system instances of a global market leading enterprise architecture [11]. In both domains, application resource planning software. Thus, the approach performance can be evaluated using either integrates data from different sources and utilizes measurement-based or model-based techniques [1]. methods and technologies related to the field of big Depending on the application life cycle, data and statistical analyses. measurement-based performance evaluation is Our approach combines the accuracy of real conducted during regular operations or as part of quality assurance systems with the cost-effectiveness stress tests or performance unit tests inside quality of performance models and data analysis. Hence, we assurance systems [12]. While model-based combine the advantages of model-based (applicable approaches can be used at design-time, at design-time) and measurement-based prediction parametrization is often difficult and involves expert (high accuracy of results) while not suffering from knowledge which may lead to low credibility of the their disadvantages (difficult parameterization, obtained results. Measurement-based approaches, on required expert knowledge, need for an implemented the other hand, require (parts of) an implemented system and sufficient training data size in operation). system and are normally limited in terms of the The following research questions narrow down configuration alternatives to be considered [11] our particular interest addressed in this paper: Do which means these are more suitable in the operation performance-related monitoring logs of enterprise phase. The advantages of both approaches could be standard software contain information that can be exploited if previously conducted measurements and extracted on a global scale in order to serve as a derived knowledge are made available for the design valuable input for new software rollouts? How of new landscapes. accurate are performance models that have been Gartner summarizes the efforts to integrate trained on mass data from different environments but monitoring data from different sources under the term similar software? Which model types are most IT operations analytics [13]. As an example, in [8], a suitable for log-based performance prediction? Our knowledge base is trained to collect performance research methodology follows the design science profiles of services in a SOA environment. These paradigm [14], where the developed artefact efforts aim at the integration of SPE and APM in a comprises the knowledge base including performance single organization (intra-organizational). In order to models that we evaluate in terms of accuracy and utilize knowledge for different organizations, the applicability in multiple iterations. specifics of this organization have to be considered in Therefore, the remainder of this paper is the applied performance models. However, in a structured as follows: In Section 2, we provide an standard application environment there is no need for overview of the state of the art related to model-based individual performance models and monitoring data and measurement-based software prediction. We can potentially be normalized and aggregated across conclude that both approaches have their several systems. Thus, knowledge that has been disadvantages. A knowledge base is proposed in applied to deploy existing landscapes can be Section 3 to combine the advantages of model-based extracted and applied to new scenarios, saving costs and measurement-based prediction as a concept and and time. Furthermore, the risk of errors, for as a technical architecture. In the evaluation, instance, by a service provider that conducts presented in Section 4, a prototype of the knowledge benchmarks, is avoided. To support intra- and inter- base is implemented and applied to monitoring data organizational collaboration for performance of nearly 19,000 business application systems. The activities during design and operations phase, we paper concludes with Section 5 in which the key follow an empirical approach that combines findings of the paper as well as possible future monitoring data from various instantiations of research activities are discussed. running enterprise applications and provides data- driven feedback for new software rollouts. 2. Software Performance Prediction Therefore, we investigate the comparability and applicability of this data to serve as an input for a domain-specific performance knowledge base which Software performance can be predicted using integrates different organizations that utilize the same either model-based or measurement-based standard software components. Within the knowledge techniques. In order to be able to classify our base, we utilize machine learning algorithms to build approach, this section summarizes the state of the art performance prediction models that we train using for
Details
-
File Typepdf
-
Upload Time-
-
Content LanguagesEnglish
-
Upload UserAnonymous/Not logged-in
-
File Pages10 Page
-
File Size-