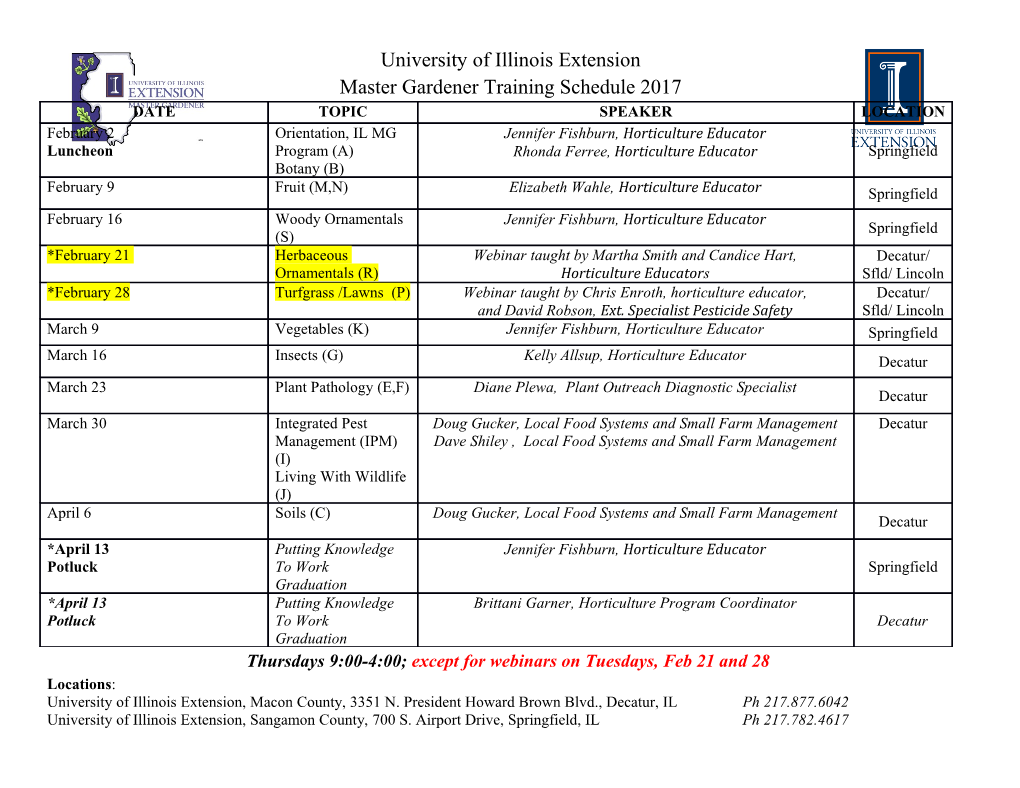
Research Statement Tony Jhwueng 2011 Summary My research focuses on developing and analyzing phylogenetic comparative methods (PCMs), studying their application to biological data sets, and relevant mathematical/statsitcal prop- erties. Breakthroughs require knowledge of phylogenetics, model selection theory, stochastic differential equations, finite difference methods, applied statistics; techniques including pro- gramming and the development of relevant software. My expertise will allow me to continue to make important contributions to these areas. Contributions and Impact Model Selection and Goodness of Fit for PCMs (Thesis): Phylogenetic comparative methods (PCMs) are statistical methods for analyzing the ecological and evo- lutionary data. Many such methods [FIC (Felsenstein 1985); PMM (Housworth et. al 2004); PA (Cheverud et. al 1985; Gittleman and Kot 1990); PGLS (Martin and Hansen 1997; Butler and King 2004)] have been proposed but their fit to data is rarely assessed. I assess the fit of several PCMs to a large collection of data sets gathered from the literature. I compared the corresponding statistical models using model selection criteria [(AIC) Akaike 1977; (AICc)Hurvich and Tsai 1990] in order to determine if the more complicated models containing additional parameters provided a significant improvement for data. Data were collected through a meta analysis on existing published phylogenetic data sets by searching all papers using a keyword search in JEB, Blackwell Synergy and JSTOR using the key- words: ((Comparative methods OR Comparative analysis) AND independent contrasts) from year 2002 to 2005. All data collected for this study are (1) averaged trait values with an associated sample size and standard deviation or with a given standard error and (2) rooted phylogenetic trees molecular clock tree or the dendrogram. I use AICc to compare the PCMs and use bootstrapping technique[Burham and Anderson 2002] to assess whether a simpler 1 Research Statement Tony Jhwueng 2011 model(FIC or ID) or a more complicated model(PMM, PA, or PGLS) with an additional parameter provide a better fit than other models in the candidate set. Due to the unknown distribution of data, I choose to use the D'Agostino normality test [D'Agostino 1990] for the univariate data to test the normal assumption of the PCMs because it combines skewness and kurtosis and is regarded as which has been shown to be informative, with good power properties over a broad range of non-normal distributions. I also perturbed the phylogenies by randomly varying the branch length without changing its topology to study the impact of small errors in the phylogeny on model selection criterion of PCMs. Conclusion. In this study, FIC and the model assuming no phylogenetic effect are the most frequently chosen as the best model. This is due in part due to the penalty AICc places on models with more parameters. While we chose to use AICc for model selection due to the fact that these models do not create a nested sequence of models, many of these models do include the extreme models as a nested component. For instance, when the heri- tability parameter h in the PMM model converges to 0 or 1, PMM is identical to ID or FIC, and thus the likelihood for PMM and one of the extreme models would be identical in this case. However, AICc penalizes PMM for using an additional parameter. Such situations also happen for PA: when ρ = 0 PA is identical to ID. Similarly OU is identical to FIC when α = 0. On the other hand, although we collected 122 data sets, the majority, 77 of them, do not pass a normality test. Thus, it might be that trait values among different species vary in a wide range such that the normality assumption common to PCMs can not be applied to describe the data. Such wide range of data might be either resulted from the heterogeneous rate of evolution occured along different lineage on the phylogeny [O'Meara et al. 2006]. Under the circumstance, developing robust, nonparametric analytical methods and models of trait evolution that do not lead to multivariate normal data seem to call for(see future project D). 2 Research Statement Tony Jhwueng 2011 While there is no evidence that the more complicated models with additional parameters are necessary to describe most comparative data, there is the question whether fitting them to data is detrimental to the analyses most commonly employed by researchers. This question is then investigated through examining the effect on correlation estimates of fitting different models to bivariate data sets. Although similar approaches have been done in literature (From Ricklefs and Starck 1996 to Revell 2010), their work are through the regression anal- ysis, my attempt uses the maximum likelihood estimation in that the MLE estimator are consistent and the bias of estimators decrease as the sample size increases. I also develop two statistical distributions for bivariate PCMs (OU and PA). Since bivariate models con- tains more parameters, an efficient optimization algorithm is also developed [analogously to Housworth et. al 2004] to improve the parameters estimation. I then use parametric boot- strapping to investigate the significance for the correlation between two different traits. My results report that most estimated correlations are concordant. Thus, if there is a significant positive correlation under one model, using a different model would also yield a significant positive correlation. This is a very reassuring result for the practical application of bivariate PCMs. Although the variation of parameters varies in quite wide range, the proportion of concordance between different PCMs in detecting non-zero correlations in actual data is very high. Therefore, researchers should apply the PCM they believe best describes the evolu- tionary mechanisms underlying their data. However, it is unlikely that any given idealized model perfectly describes both traits and their joint evolution in a given bivariate analysis. Current and Future Projects A. Modeling PCMs with Hybridizations (with Elizabeth Housworth): Hybrid species are known for sharing some common phenotypes from their parents. The rate of variation of the trait between hybrid and its parents has not been studied and reported through comparative analysis. Two improved phylogenetic comparative method (PCMs) are proposed to allow for data sets that involve hybrids. Instead of using phylogenetic trees, 3 Research Statement Tony Jhwueng 2011 the new methods analyze comparative data by incorporating phylogenetic networks where ancient hybrids are explicitly identified. The new methods can also be applied to test the heterogeneous hybrid effects when multiple hybrids are presented on the network. Simula- tion studies for accessing the robustness of the new method indicate that the corresponding statistical models are sensitive to the timing of the ancient hybridization. Increased power and decreased bias were found when the hybridization event occurred more recently. Finally, we incorporate the phylogenetic network in [Koblm¨ulleret. al 2008] to analyze the rate of variation for body lengths of cichilds. B. Impact of AICc on Models of DNA Evolution (With Brian O'Meara): We inves- tigate the impact of Akaike Information Criterion (AIC) on selecting the models of nucleotide substitution. Our approach focuses on the sample size correction criterion AICc[Hurvich and Tsai 1990] where three different versions of sample sizes: (i) the number of site used in the alignment, (ii) the number of taxa, (iii) the product of number of site and number of taxa are considered for AICc. We find that when small data set are used, in particular for using only one site in the alignment, version (ii) prefers the simpler model while version (i) choose more complicated models. C. An Statistical Approach of an improved comparative method for studying adaptation to a randomly evolving environment (with Vasileios Maroulas): We improve a phylogenetic comparative method for correcting the mal-adaptation. We compare our new model (OU-OU) to the Hansen's model (BM-OU)[Hansen et al. 2008] by evaluating the ability in estimating the phylogenetic inertia and the performance of evolutionary and optimal regressions. Both models are built around an Ornstein-Uhlenbeck process, the main difference between the OU-OU and BM-OU is the stochastic assumption on the optimumsa. Simulation studies on investigating the bias and power of the estimators for both models will also be assessed. 4 Research Statement Tony Jhwueng 2011 D. A non-Gaussian multivariate distribution of phylogenetic tree dependency (Independent project): Phylogenetic comparative methods(PCMs) incorporate informa- tion on the evolutionary relationships of organisms (phylogenetic trees) to compare species. However, trait values among different species vary in a wide range such that the normality assumption common to PCMs can not be applied to describe the data. Neglecting the nor- mality assumption and proceeding data analysis often lead to error and eventually change the end conclusion. We develop robust parametric analytical methods and models of trait evolution that do not lead to multivariate normal data. We expect that by simulation our model could fit heavy tail data set (log-normal and log-gamma data), persists good power property on the violation of the normality and outperforms other PCMs under the normality assumption to large collection of real comparative data set. E. Optimal Taxa Sampling Problem in Phylogenetic Comparative Methods (In- dependent project): Given a large molecular-clock based phylogeny with N taxa, it is not always possible to sample all N species. Hence the question: if we can sample k species from these N, which should we choose? For a univariate analysis, that typically means finding the sample that minimizing the variation in the estimate of the mean. This problem will be investigated under various PCMs [FIC, PGLS, PMM, and PA]. With different evolutionary assumptions, the structure of the similarity matrices for those PCMs are quite different and the solution may not be the same if the answers depend on more than just the branching order.
Details
-
File Typepdf
-
Upload Time-
-
Content LanguagesEnglish
-
Upload UserAnonymous/Not logged-in
-
File Pages6 Page
-
File Size-