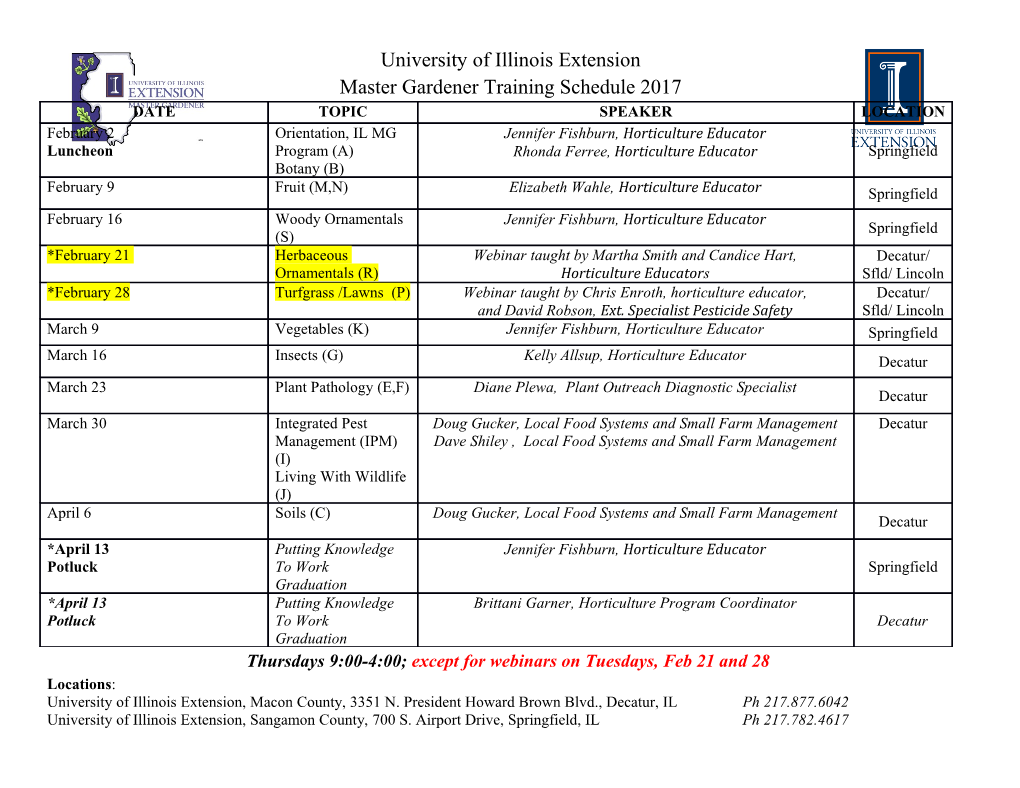
Geosci. Model Dev., 11, 4577–4602, 2018 https://doi.org/10.5194/gmd-11-4577-2018 © Author(s) 2018. This work is distributed under the Creative Commons Attribution 4.0 License. The NASA Eulerian Snow on Sea Ice Model (NESOSIM) v1.0: initial model development and analysis Alek A. Petty1,2, Melinda Webster1, Linette Boisvert1,2, and Thorsten Markus1 1Cryospheric Sciences Laboratory, NASA Goddard Space Flight Center, Greenbelt, MD, USA 2Earth System Science Interdisciplinary Center, University of Maryland, College Park, MD, USA Correspondence: Alek A. Petty ([email protected]) Received: 23 March 2018 – Discussion started: 7 May 2018 Revised: 14 October 2018 – Accepted: 18 October 2018 – Published: 16 November 2018 Abstract. The NASA Eulerian Snow On Sea Ice Model (NE- rived from NASA’s Operation IceBridge (OIB) snow radar SOSIM) is a new, open-source snow budget model that is cur- data from 2009 to 2015, showing moderate–strong correla- rently configured to produce daily estimates of the depth and tions and root mean squared errors of ∼ 10 cm depending density of snow on sea ice across the Arctic Ocean through on the OIB snow depth product analyzed, similar to the com- the accumulation season. NESOSIM has been developed in parisons between OIB snow depths and the commonly used a three-dimensional Eulerian framework and includes two modified Warren snow depth climatology. Potential improve- (vertical) snow layers and several simple parameterizations ments to this initial NESOSIM formulation are discussed in (accumulation, wind packing, advection–divergence, blow- the hopes of improving the accuracy and reliability of these ing snow lost to leads) to represent key sources and sinks simulated snow depths and densities. of snow on sea ice. The model is forced with daily inputs of snowfall and near-surface winds (from reanalyses), sea ice concentration (from satellite passive microwave data) and sea ice drift (from satellite feature tracking) during the accu- 1 Introduction mulation season (August through April). In this study, we present the NESOSIM formulation, calibration efforts, sen- Snow on sea ice is a crucial component of the polar cli- sitivity studies and validation efforts across an Arctic Ocean mate system. Its low thermal conductivity modulates sea ice domain (100 km horizontal resolution). The simulated snow growth through the cold winter months (e.g., Maykut and depth and density are calibrated with in situ data collected Untersteiner, 1971; Sturm et al., 2002), while its high surface on drifting ice stations during the 1980s. NESOSIM shows albedo limits solar radiation absorption and thus inhibits sea strong agreement with the in situ seasonal cycles of snow ice melt in spring and summer (e.g., Warren, 1982; Grenfell depth and density, and shows good (moderate) agreement and Perovich, 1984; Perovich et al., 2002). Conversely, fresh- with the regional snow depth (density) distributions. NE- water production from snowmelt facilitates melt pond forma- SOSIM is run for a contemporary period (2000 to 2015), tion in spring–summer which lowers the surface albedo and with the results showing strong sensitivity to the reanalysis- promotes sea ice melt (Eicken et al., 2002, 2004). The ac- derived snowfall forcing data, with the Modern-Era Retro- cumulation of snow on sea ice also modulates the freshwater spective analysis for Research and Applications (MERRA) flux into the ocean, a key component of the freshwater budget and the Japanese Meteorological Agency 55-year reanalysis of the Arctic (e.g., Serreze et al., 2006). (JRA-55) forced snow depths generally higher than ERA- Estimates of snow depth on sea ice are also a required in- Interim, and the Arctic System Reanalysis (ASR) generally put for deriving sea ice thickness from satellite altimetry, e.g., lower. We also generate and force NESOSIM with a consen- from ESA’s CryoSat-2 (e.g., Laxon et al., 2013) and NASA’s sus “median” daily snowfall dataset from these reanalyses. upcoming Ice, Cloud, and land Elevation Satellite (ICESat) The results are compared against snow depth estimates de- mission (Markus et al., 2017). The altimetry technique in- volves measurements of sea ice freeboard, the extension of Published by Copernicus Publications on behalf of the European Geosciences Union. 4578 A. A. Petty et al.: NESOSIM sea ice above a local sea level, and estimates of snow depth have recently undergone various improvements to their snow to derive sea ice thickness, with snow depth being one of physics (Holland et al., 2011; Lecomte et al., 2015). the primary sources of uncertainty for both laser and radar In this study, we present a new model to derive snow depth altimetry (e.g., Giles et al., 2007). Poor knowledge of snow (and density) across the Arctic Ocean. Our aim is to develop density provides a further source of uncertainty through its a model of physical and computational simplicity to allow influence on the ice freeboard and radar penetration into the for a detailed assessment of the sensitivity of snow depths snowpack (e.g., Giles et al., 2007; Kern et al., 2015). to the various input forcing data needed to produce seasonal Unfortunately, direct observations of snow depth and den- basin-scale snow depths. The spread in reanalysis-derived sity across the polar oceans are very limited, due to diffi- snowfall estimates over the Arctic Ocean is high (Boisvert culties in remotely sensing this relatively thin (O(10 cm)) et al., 2018), while the importance and uncertainty of other and heterogeneous medium and logistical challenges asso- forcing data (e.g., ice concentration and drift) are still largely ciated with in situ data collection. Passive microwave data unknown. We also wanted a model that could be forced with have been used to estimate snow depth over first-year ice observed ice concentration and drift to help accurately con- on a basin scale across both poles (e.g., Markus and Cav- strain the seasonal sea ice cycle – a challenge for the more so- alieri, 1998; Comiso et al., 2003; Maass et al., 2015), al- phisticated sea ice models described above. Our overall goal though these data are arguably more relevant for the first- is that NESOSIM can be used to produce reliable basin-scale year-dominated Antarctic sea ice pack and tend to underes- daily snow depth and density estimates needed for satellite timate snow depth in deformed sea ice regimes (e.g., Worby altimetry calculations of sea ice thickness for both histor- et al., 2008; Brucker and Markus, 2013). Combinations of ical analyses and near-real-time operations across the po- satellite and/or airborne sensors with variable snow penetra- lar oceans. We thus expect the model to increase in com- tion depths are also being explored as a means of producing plexity with future model developments, e.g., new parame- basin-scale snow depth estimates (e.g., Armitage and Rid- terizations or improvements to existing parameterizations as out, 2015; Guerreiro et al., 2016; Kwok and Markus, 2017), needed. A secondary utility of the model will be the produc- although this approach is still in its infancy and has limited tion of daily/monthly/seasonal snow depths from reanalysis temporal coverage. NASA’s Operation IceBridge has pro- data that can help guide climate modeling research efforts vided airborne measurements of snow depth on sea ice since addressing the representation and importance of snow on sea 2009 (Kurtz et al., 2013). However, the Arctic snow depth ice in the global climate system. data collected are primarily limited to the western Arctic sea In the following sections, we present and describe the ice cover in spring (the spring 2017 campaign also included model configuration/physics, the sensitivity of the model to a flight over the eastern Arctic Ocean), while the Southern the input forcing data (e.g., reanalyses snowfall, satellite- Ocean data have only been briefly explored to date (e.g., derived ice drifts) and model calibration/validation efforts. Kwok and Maksym, 2014). For the Arctic, a climatology of We focus this initial study solely on the Arctic; however, our snow depth produced from Soviet drifting station data col- plan is for the model to be applied and tested in a Southern lected prior to 1991 (Warren et al., 1999) is still commonly Ocean framework in the near future. We conclude by look- used as a basin-scale snow depth product. The Soviet drift- ing ahead to potential improvements in the model physics ing station data also provide the only observationally based and planned future activities related to our efforts to improve basin-scale assessment of snow density currently available. our understanding of snow on sea ice. This snow climatology is expected to be outdated due to the rapid changes experienced in the Arctic climate system over the last few decades (Webster et al., 2014), although re- 2 Model description cent efforts have been made to modify this climatology based The NASA Eulerian Snow On Sea Ice Model (NESOSIM) is on ice type (halving the climatology over first-year ice, e.g., a three-dimensional, two-layer (vertical) Eulerian snow bud- Laxon et al., 2013; Kwok and Cunningham, 2015). get model developed with the primary aim of producing daily Due to these observational limitations, the sea ice com- estimates of snow depth and density across the polar oceans. munity often utilizes simple models of snow depth forced NESOSIM includes several parameterizations that represent by reanalyses (primarily snowfall data) (e.g., Maksym and key mechanisms of snow variability through the snow accu- Markus 2008; Kwok and Cunningham, 2008; Blanchard- mulation/growth season and two snow layers to broadly rep- Wrigglesworth et al., 2018). More sophisticated snow on sea resent the evolution of both old, compacted snow and new, ice models are available, such as SnowModel, a terrestrial fresh snow.
Details
-
File Typepdf
-
Upload Time-
-
Content LanguagesEnglish
-
Upload UserAnonymous/Not logged-in
-
File Pages26 Page
-
File Size-