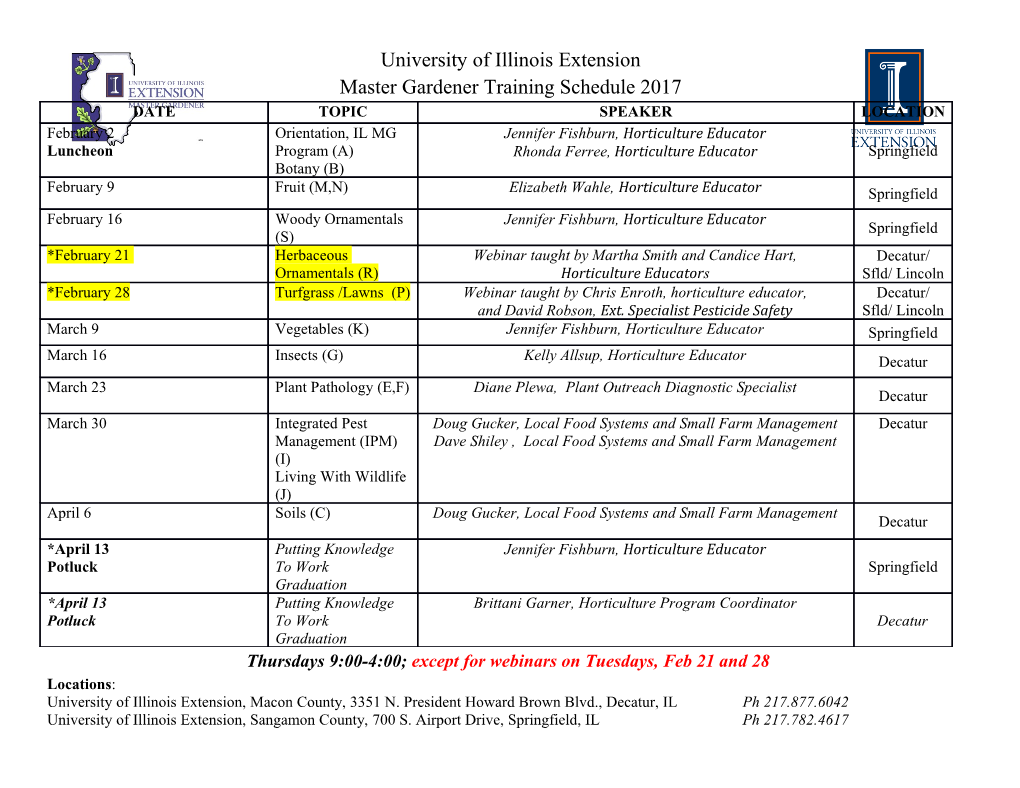
Recommending Music with Waveform-based Architectures ORIOL NIETO GLOBAL BIG DATA CONFERENCE SANTA CLARA, CA JAN 21, 2019 @urinieto Pandora Confidential OUTLINE Background: Collaborative Filtering Music Recommendation Demo OUTLINE Background: Collaborative Filtering Music Recommendation Demo Collaborative Filtering RECOMMENDING “POPULAR” ITEMS ? ? [ ? ? ? ? Items (Tracks) ? ? [ ? ? Users Collaborative Filtering PROBLEM OVERVIEW [ [ k ? ? [ ? ? ? ? k Items (Tracks) ? ? ⇡ Items (Tracks) ? ? [ [ [ Users Users Koren, Y., Bell, R., & Volinsky, C. (2009). Matrix Factorization Techniques for Recommender Systems. Computer, 42(8), 42–49. Collaborative Filtering PROBLEM OVERVIEW [ [ k ? ? [ ? ? ? ? k Items (Tracks) ? ? ⇡ Items (Tracks) ? ? [ [ [ Users Users Koren, Y., Bell, R., & Volinsky, C. (2009). Matrix Factorization Techniques for Recommender Systems. Computer, 42(8), 42–49. Collaborative Filtering PROBLEM OVERVIEW [ [ k ? ? [ ? ? ? ? k Items (Tracks) ? ? ⇡ Items (Tracks) ? ? [ [ [ Users Users Koren, Y., Bell, R., & Volinsky, C. (2009). Matrix Factorization Techniques for Recommender Systems. Computer, 42(8), 42–49. Collaborative Filtering LATENT FACTORS Complex Harmony Calm Aggressive Simple Harmony Collaborative Filtering THE GOOD AND THE BAD Rich preference-driven similarity space Latent space is generally not interpretable Can only recommend items tHat Powerful at MatcHing tHe rigHt song have already been rated witH tHe right listener (what about long tail content?) OUTLINE Background: Collaborative Filtering Music RecoMMendation Demo Music Recommendation WITH COLLABORATIVE SONG FACTORS [ [ k ? ? [ ? ? ? ? k Items (Tracks) ? ? ⇡ Items (Tracks) ? ? [ [ [ Users Users Music Recommendation WITH COLLABORATIVE SONG FACTORS [ [ k ? ? [ ? ? ? ? Songs Songs k ? ? ⇡ ? ? [ [ [ Seeds Seeds Collaborative Filtering EXAMPLE ArSst Title Query Track Journey Don’t Stop Believing Collaborative Filtering EXAMPLE ArSst Title Query Track Journey Don’t Stop Believing Ranked 1 The Ouield Your Love Ranked 2 Eagles Hotel California Ranked 3 Survivor Eye Of The Tiger Ranked 4 Queen We Will Rock You Collaborative Filtering EXAMPLE ArSst Title Query Track Journey Don’t Stop Believing Ranked 1 The Ouield Your Love Ranked 2 Eagles Hotel California Ranked 3 Survivor Eye Of The Tiger Ranked 4 Queen We Will Rock You Collaborative Filtering EXAMPLE ArSst Title Query Track Journey Don’t Stop Believing Ranked 1 The Ouield Your Love Ranked 2 Eagles Hotel California Ranked 3 Survivor Eye Of The Tiger Ranked 4 Queen We Will Rock You Collaborative Filtering EXAMPLE ArSst Title Query Track Journey Don’t Stop Believing Ranked 1 The Ouield Your Love Ranked 2 Eagles Hotel California Ranked 3 Survivor Eye Of The Tiger Ranked 4 Queen We Will Rock You The Music Genome Project LARGE-SCALE HUMAN ANNOTATED DATASET Attribute Examples Breathy Voice Nasal Voice Odd Meter Has Banjo Joyful Lyrics … Up to ~400 attributes per track Music Genome Project EXAMPLE ArSst Title Query Track Journey Don’t Stop Believing Ranked 1 Journey Stone In Love Ranked 2 Jefferson Starship Find Your Way Back Ranked 3 David Bowie Teenage Wildlife Ranked 4 Thriving Ivory On Your Side Music Genome Project EXAMPLE ArSst Title Query Track Journey Don’t Stop Believing Ranked 1 Journey Stone In Love Ranked 2 Jefferson Starship Find Your Way Back Ranked 3 David Bowie Teenage Wildlife Ranked 4 Thriving Ivory On Your Side Music Genome Project EXAMPLE ArSst Title Query Track Journey Don’t Stop Believing Ranked 1 Journey Stone In Love Ranked 2 Jefferson Starship Find Your Way Back Ranked 3 David Bowie Teenage Wildlife Ranked 4 Thriving Ivory On Your Side Music Genome Project EXAMPLE ArSst Title Query Track Journey Don’t Stop Believing Ranked 1 Journey Stone In Love Ranked 2 Jefferson Starship Find Your Way Back Ranked 3 David Bowie Teenage Wildlife Ranked 4 Thriving Ivory On Your Side Estimating Latent Factors [ [ k ? ? [ ? ? ? ? k Items (Tracks) ? ? ⇡ Items (Tracks) ? ? [ [ [ Users Users Estimating Latent Factors FROM THE MUSIC GENOME PROJECT k y<latexit sha1_base64="(null)">(null)</latexit> <latexit sha1_base64="(null)">(null)</latexit> x http://blogs-images.forbes.com/kevinmurnane/files/2016/03/google-deepmind-artificial-intelligence-2-970x0-970x646.jpg f(x; ✓) y <latexit sha1_base64="(null)">(null)</latexit> ⇡ Estimating Latent Factors FROM THE MUSIC GENOME PROJECT N 2048 2048 2048 k Dense Layers Estimating Latent Factors DATA AND OPTIMIZATION • Data set : X, Y { } • ~900k tracks (from “head”) M<latexit sha1_base64="(null)">(null)</latexit> • Loss : (✓) L<latexit sha1_base64="(null)">(null)</latexit> • Cosine Distance • Dropout 10% in Dense Layers 1 f(x; ✓)T y • Batch Normalization in all layers (✓)=1 L − M f(x; ✓) 2 y 2 <latexit sha1_base64="(null)">(null)</latexit> x ,y • Adam optimizer 2XX 2Y || || || || Estimating Latent Factors RESULTS Input Cos Distance # EpocHs Time / Epoch MGP 0.30 15 ~4m Latent Factor Estimations WITH THE MUSIC GENOME PROJECT ArSst Title Query Track Journey Don’t Stop Believing Ranked 1 Journey Stone In Love Ranked 2 D Drive A Limle Bima Sunshine Ranked 3 John Parr Naughty, Naughty Ranked 4 Kiss Turn On The Night Latent Factor Estimations WITH THE MUSIC GENOME PROJECT ArSst Title Query Track Journey Don’t Stop Believing Ranked 1 Journey Stone In Love Ranked 2 D Drive A Limle Bima Sunshine Ranked 3 John Parr Naughty, Naughty Ranked 4 Kiss Turn On The Night Latent Factor Estimations WITH THE MUSIC GENOME PROJECT ArSst Title Query Track Journey Don’t Stop Believing Ranked 1 Journey Stone In Love Ranked 2 D Drive A Limle Bima Sunshine Ranked 3 John Parr Naughty, Naughty Ranked 4 Kiss Turn On The Night Latent Factor Estimations WITH THE MUSIC GENOME PROJECT ArSst Title Query Track Journey Don’t Stop Believing Ranked 1 Journey Stone In Love Ranked 2 D Drive A Limle Bima Sunshine Ranked 3 John Parr Naughty, Naughty Ranked 4 Kiss Turn On The Night Machine Listening ESTIMATING THE MUSIC GENOME PROJECT Pons, J., et al. ISMIR 2018 Estimating Latent Factors FROM THE MUSIC GENOME PROJECT ESTIMATIONS k http://blogs-images.forbes.com/kevinmurnane/files/2016/03/google-deepmind-artificial-intelligence-2-970x0-970x646.jpg Estimating Latent Factors FROM THE MUSIC GENOME PROJECT ESTIMATIONS N 2048 2048 2048 k Dense Layers Estimating Latent Factors RESULTS Input Cos Distance # EpocHs Time / Epoch MGP 0.30 15 ~4m MGP Esnmanons 0.44 21 ~4m Latent Factor Estimations WITH MACHINE LISTENING ATTRIBUTES ArSst Title Query Track Journey Don’t Stop Believing Ranked 1 Dean Friedman Don’t You Ever Dare Ranked 2 James Taylor Stand And Fight Ranked 3 The Dingoes Starnng Today Ranked 4 Chuck Girad The Days Are Young Latent Factor Estimations WITH MACHINE LISTENING ATTRIBUTES ArSst Title Query Track Journey Don’t Stop Believing Ranked 1 Dean Friedman Don’t You Ever Dare Ranked 2 James Taylor Stand And Fight Ranked 3 The Dingoes Starnng Today Ranked 4 Chuck Girad The Days Are Young Latent Factor Estimations WITH MACHINE LISTENING ATTRIBUTES ArSst Title Query Track Journey Don’t Stop Believing Ranked 1 Dean Friedman Don’t You Ever Dare Ranked 2 James Taylor Stand And Fight Ranked 3 The Dingoes Starnng Today Ranked 4 Chuck Girad The Days Are Young Latent Factor Estimations WITH MACHINE LISTENING ATTRIBUTES ArSst Title Query Track Journey Don’t Stop Believing Ranked 1 Dean Friedman Don’t You Ever Dare Ranked 2 James Taylor Stand And Fight Ranked 3 The Dingoes Starnng Today Ranked 4 Chuck Girad The Days Are Young Estimating Latent Factors FROM AUDIO k http://blogs-images.forbes.com/kevinmurnane/files/2016/03/google-deepmind-artificial-intelligence-2-970x0-970x646.jpg Oord, A. Van Den, Dieleman, S., & Schrauwen, B. (2013). Deep Content-based Music Recommendation. Advances in Neural Information Processing Systems, 2643–2651. Estimating Latent Factors FROM RAW WAVEFORMS k y<latexit sha1_base64="(null)">(null)</latexit> <latexit sha1_base64="(null)">(null)</latexit> x http://blogs-images.forbes.com/kevinmurnane/files/2016/03/google-deepmind-artificial-intelligence-2-970x0-970x646.jpg f(x; ✓) y <latexit sha1_base64="(null)">(null)</latexit> ⇡ Estimating Latent Factors FROM RAW WAVEFORMS Conv1D Conv1D MP Conv1D MP Conv1D MP 64 x 3 64 x 3 3 64 x 3 3 128 x 3 3 <latexit sha1_base64="(null)">(null)</latexit> x k Conv1D MP Conv1D MP Conv1D Conv1D 1024 1024 1024 128 x 3 3 128 x 3 3 128 x 3 128 x 3 y<latexit sha1_base64="(null)">(null)</latexit> Conv1D Conv1D Conv1D Conv1D 256 x 3 512 x 7 512 x 7 512 x 7 Dense Layers Auto Pooling https://github.com/jordipons/music-audio-tagging-at-scale-models Lee, J., et al., 2018 McFee, B., et al., 2018 Estimating Latent Factors DATA AND OPTIMIZATION • Data set : X, Y • ~900k tracks (from “head”) { } • 16kHz - 16 bit waveforms M<latexit sha1_base64="(null)">(null)</latexit> • 3 patches of 15 seconds per track (~2.7M patches) • Loss : (✓) L<latexit sha1_base64="(null)">(null)</latexit> • Cosine Distance • Dropout 10% in Dense Layers 1 f(x; ✓)T y • Batch Normalization in all layers (✓)=1 L − M f(x; ✓) 2 y 2 <latexit sha1_base64="(null)">(null)</latexit> x ,y • Adam optimizer 2XX 2Y || || || || Estimating Latent Factors RESULTS Input Cos Distance # EpocHs Time / Epoch MGP 0.30 15 ~4m MGP Esnmanons 0.44 21 ~4m Spectrogram (35s patches) 0.37 22 ~2h Estimating Latent Factors RESULTS Input Cos Distance # EpocHs Time / Epoch MGP 0.30 15 ~4m MGP Esnmanons 0.44 21 ~4m Spectrogram (35s patches) 0.37 22 ~2h Waveform (15s patches) 0.34 9 ~5h Latent Factor Estimations WITH WAVEFORMS ArSst Title Query Track Journey Don’t Stop Believing Ranked 1 The Games Band The Final Countdown Ranked 2 Survivor Backstreet Love Affair
Details
-
File Typepdf
-
Upload Time-
-
Content LanguagesEnglish
-
Upload UserAnonymous/Not logged-in
-
File Pages61 Page
-
File Size-