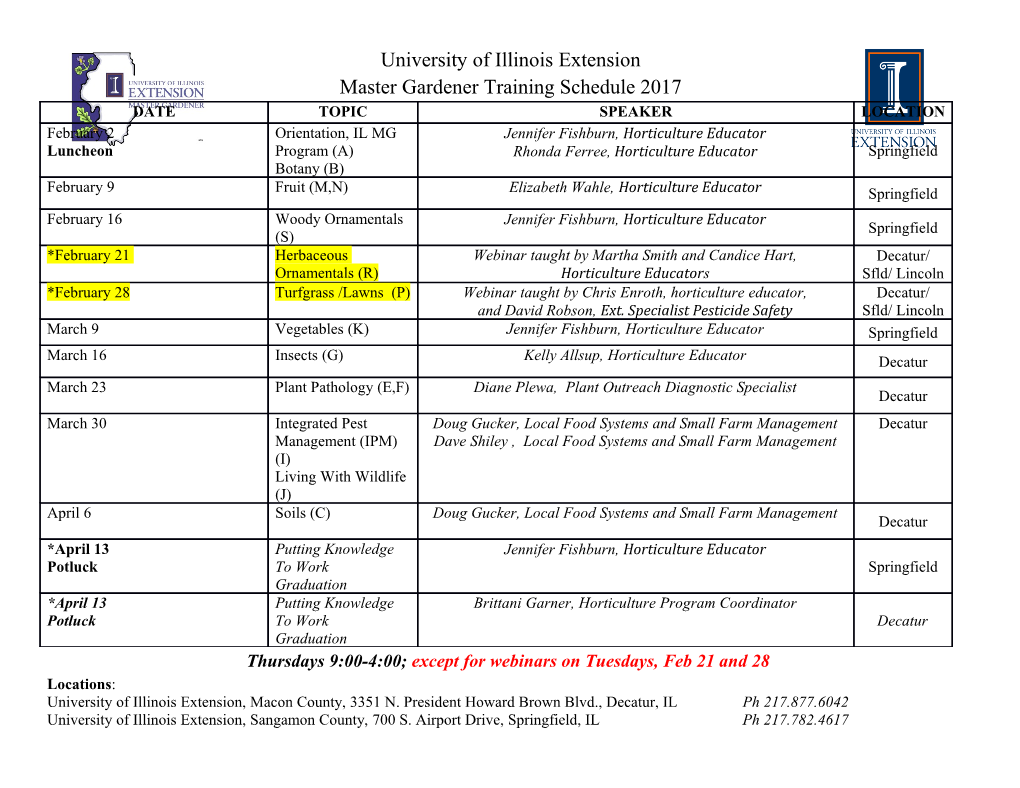
Proceedings of the Twenty-Seventh International Joint Conference on Artificial Intelligence (IJCAI-18) Automatic Opioid User Detection From Twitter: Transductive Ensemble Built On Different Meta-graph Based Similarities Over Heterogeneous Information Network Yujie Fan, Yiming Zhang, Yanfang Ye ∗, Xin Li Department of Computer Science and Electrical Engineering, West Virginia University, WV, USA fyf0004,[email protected], fyanfang.ye,[email protected] Abstract 13,000 people died from heroin overdose, both reflecting sig- nificant increase from 2002 [NIDA, 2017]. Opioid addiction Opioid (e.g., heroin and morphine) addiction has is a chronic mental illness that requires long-term treatment become one of the largest and deadliest epidemics and care [McLellan et al., 2000]. Although Medication As- in the United States. To combat such deadly epi- sisted Treatment (MAT) using methadone or buprenorphine demic, in this paper, we propose a novel frame- has been proven to provide best outcomes for opioid addiction work named HinOPU to automatically detect opi- recovery, stigma (i.e., bias) associated with MAT has limited oid users from Twitter, which will assist in sharp- its utilization [Saloner and Karthikeyan, 2015]. Therefore, ening our understanding toward the behavioral pro- there is an urgent need for novel tools and methodologies to cess of opioid addiction and treatment. In HinOPU, gain new insights into the behavioral processes of opioid ad- to model the users and the posted tweets as well diction and treatment. as their rich relationships, we introduce structured In recent years, the role of social media in biomedical heterogeneous information network (HIN) for rep- knowledge mining has become more and more important resentation. Afterwards, we use meta-graph based [Hawn, 2009; Alkhateeb et al., 2011]. Due to the increas- approach to characterize the semantic relatedness ing use of the Internet, never-ending growth of data is gener- over users; we then formulate different similari- ated from the social media which offers opportunities for the ties over users based on different meta-graphs on users to freely share opinions and experiences in online com- HIN. To reduce the cost of acquiring labeled sam- munities. For example, Twitter, as one of the most popular ples for supervised learning, we propose a trans- social media platforms, has averaged at 328 million monthly ductive classification method to build the base clas- active users as of the second quarter of 2017 [Neha, 2017]. sifiers based on different similarities formulated by Many Twitter users are willing to share their experiences of different meta-graphs. Then, to further improve the using opioids (e.g., “It started with anorexia as I was 11, with detection accuracy, we construct an ensemble to 13 I was on heroin. At every time I leave heroin I fall into combine different predictions from different base anorexia”), and perceptions toward MAT (e.g., “I stopped classifiers for opioid user detection. Comprehen- with heroin bc I’m having the methadone, that is the most sive experiments on real sample collections from effective treatment.”). Thus, the data from social media may Twitter are conducted to validate the effectiveness contribute information beyond the knowledge of domain pro- of HinOPU in opioid user detection by compar- fessionals (e.g., psychiatrists and epidemics researchers) and isons with other alternate methods. could assist in sharpening our understanding toward the be- havioral process of opioid addiction and treatment. 1 Introduction To combat the opioid addiction epidemic and promote the practice of MAT, in this paper, we propose a novel frame- Opioids are a class of drugs including the illegal drug heroin work named HinOPU, a transductive ensemble classification and powerful pain relievers by legal prescription, such as model built on different meta-graph based similarities over morphine and oxycodone [NIDA, 2014]. Opioid addiction heterogeneous information network (HIN), to automatically has become one of the largest and deadliest epidemics in the detect opioid users from Twitter. To model the users and the U.S. [NIDA, 2017], which has also turned into a serious na- posted tweets as well as their rich semantic relationships, in tional crisis that affects public health as well as social and HinOPU, we first introduce a HIN [Sun et al., 2011] for rep- economic welfare. There was a skyrocketing increase of opi- resentation. To capture the complex relationship (e.g. two oid related death in the past decade: according to Centers for users are relevant if they have posted tweets that discussed Disease Control and Prevention (CDC), in 2015, more than the same topic, mentioned same people, and also reposted 32,000 Americans died from opioid drugs overdoses and over same tweets from others), we use meta-graphs [Zhao et al., 2017] to incorporate higher-level semantics to build up relat- ∗Corresponding author. edness over users. Different similarities over users can then 3357 Proceedings of the Twenty-Seventh International Joint Conference on Artificial Intelligence (IJCAI-18) Figure 1: System architecture of HinOPU. be formulated by different meta-graphs. As obtaining the la- supervised learning, transductive classification method is beled data (either opioid users or not) from Twitter is both proposed to build the base classifiers; then an ensemble time-consuming and cost-expensive, to reduce the cost of ac- approach is presented to combine different results from the quiring labeled samples for supervised learning, we propose a base classifiers to make final predictions. (See Section 3.3.) transductive classification method to build the base classifiers based on different similarities formulated by different meta- 3 Proposed Method graphs; to further improve the detection accuracy, we then In this section, we introduce the detailed approaches of how present an ensemble approach to combine different predic- we represent Twitter users, and how we solve the problem of tions from different base classifiers for opioid user detection. opioid user detection based on this representation. 2 System Architecture 3.1 HIN Construction The system architecture of HinOPU is shown in Figure.1, To detect whether a user is an opioid user or not from Twit- which consists of the following four major components: ter, besides the users’ posted tweets, the following kinds of relationships are also considered: • Data collector and preprocessor. We first develop web crawling tools to collect opioid-related tweets (i.e., the • R1: To describe the relation of a user and his/her posted tweets include opioid names or their common street names) tweet, we build the user-post-tweet matrix P where each as well as users’ profiles from Twitter. For privacy protec- element pi;j 2 f0; 1g denotes if user i posts tweet j. tion, UserID is used to anonymize each user. For the col- • R2: To denote the relation that a user appreciates a tweet, lected tweets, the preprocessor will remove all the links, we generate the user-like-tweet matrix L where each ele- punctuations, stopwords, and conduct lemmatization using ment li;j 2 f0; 1g means if user i likes tweet j. Stanford CoreNLP [Manning et al., 2014]; as street names can be used to imply opioids (e.g., smack is used to refer • R3: Two users can follow each other in Twitter. To rep- heroin) and may also have different meanings when given resent such kind of user-user relationship, we generate different contexts, we will then perform word sense dis- the user-follow-user matrix F where each element fi;j 2 ambiguation (WSD) [Zhong and Ng, 2010] to decide if a f0; 1g denotes whether user i and user j follow each other. tweet containing the street name is an opioid-related tweet. • R4: If a tweet includes symbol @ followed by a user name, • Feature extractor and HIN constructor. A bag-of-words it means that the user is mentioned and talked publicly in feature vector is extracted to represent each user’s posted the tweet. To describe such tweet-user relationship, we tweet(s); then the relationships among users, tweets and build the tweet-mention-user matrix A where each ele- topics will be further analyzed, such as, i) user-post-tweet, ment ai;j 2 f0; 1g indicates if tweet i mentions user j. ii) user-like-tweet, iii) user-follow-user, iv) tweet-mention- • R5: A tweet can be a repost of another tweet. To represent user, v) tweet-RT-tweet, and vi) tweet-contain-topic. Based such relationship between two tweets, we build the tweet- on these extracted features, a structural HIN will be con- RT-tweet matrix X where element xi;j 2 f0; 1g denotes structed. (See Section 3.1.) whether tweet i or tweet j is a repost of the other. • Meta-graph based similarity builder. In this module, dif- • R6: To represent the relation that a tweet contains a specific ferent meta-graphs are first built from HIN to capture the topic, we generate the tweet-contain-topic matrix C whose relatedness between users. Then, we integrate similarity element ci;j 2 f0; 1g indicates whether tweet i contains of users’ posted tweets and relatedness depicted by each topic j. In our case, we use Latent Dirichlet Allocation meta-graph to formulate a set of similarity measures over [Blei et al., 2003] for topic extraction from posted tweets. users. (See Section 3.2.) In order to depict users, tweets, topics and the rich re- • Transductive ensemble. Based on different similarities lationships among them (i.e., user-user, user-tweet, tweet- formulated by different meta-graphs in the previous mod- tweet, tweet-topic relations), it is important to model them in ule, to reduce the cost of acquiring labeled samples for a proper way so that different kinds of relations can be better 3358 Proceedings of the Twenty-Seventh International Joint Conference on Artificial Intelligence (IJCAI-18) and easier handled.
Details
-
File Typepdf
-
Upload Time-
-
Content LanguagesEnglish
-
Upload UserAnonymous/Not logged-in
-
File Pages7 Page
-
File Size-