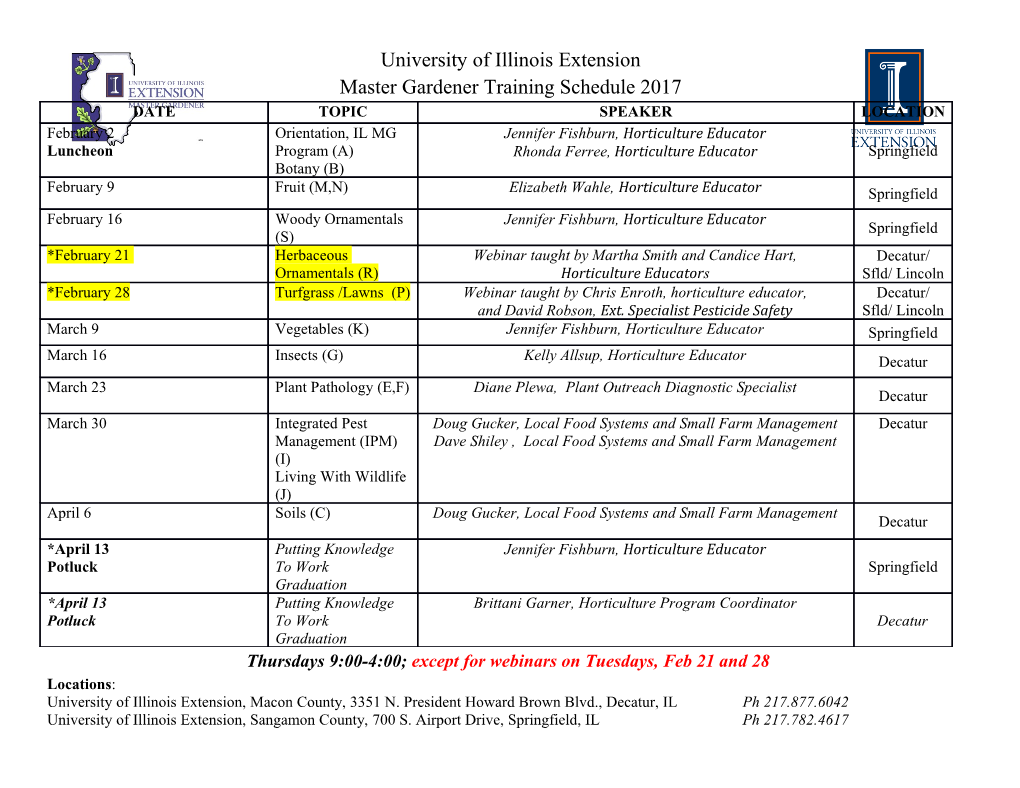
Biometrika Trust Assessing the Accuracy of the Maximum Likelihood Estimator: Observed Versus Expected Fisher Information Author(s): Bradley Efron and David V. Hinkley Source: Biometrika, Vol. 65, No. 3 (Dec., 1978), pp. 457-482 Published by: Oxford University Press on behalf of Biometrika Trust Stable URL: http://www.jstor.org/stable/2335893 Accessed: 12-02-2016 22:02 UTC Your use of the JSTOR archive indicates your acceptance of the Terms & Conditions of Use, available at http://www.jstor.org/page/ info/about/policies/terms.jsp JSTOR is a not-for-profit service that helps scholars, researchers, and students discover, use, and build upon a wide range of content in a trusted digital archive. We use information technology and tools to increase productivity and facilitate new forms of scholarship. For more information about JSTOR, please contact [email protected]. Biometrika Trust and Oxford University Press are collaborating with JSTOR to digitize, preserve and extend access to Biometrika. http://www.jstor.org This content downloaded from 165.91.114.21 on Fri, 12 Feb 2016 22:02:21 UTC All use subject to JSTOR Terms and Conditions Biometrika (1978), 65, 3, pp. 457-87 457 With 11 text-figures Printed in Great Britain Assessingthe accuracyof the maximumlielihood estimator: Observedversus expected Fisher information BY BRADLEY EFRON Departmentof Statistics, Stanford University,California AND DAVID V. HINKLEY School of Statistics, University of Minnesota, Minneapolis SUMMARY This paper concernsnormal approximationsto the distribution of the maximum likelihood estimator in one-parameterfamilies. The traditional variance approximation is 1/1.I, where 0 is the maximum likelihood estimator and fo is the expected total Fisher information. Many writers, including R. A. Fisher, have argued in favour of the variance estimate I/I(x), where I(x) is the observed information, i.e. minus the second derivative of the log likelihood function at # given data x. We give a frequentist justification for preferring I/I(x) to 1/.Io. The former is shown to approximate the conditional variance of # given an appropriate ancillary statistic which to a first approximation is I(x). The theory may be seen to flow naturally from Fisher's pioneering papers on likelihood estimation. A large number of examples are used to supplement a small amount of theory. Our evidence indicates preference for the likelihood ratio method of obtaining confidencelimits. Some key words: Ancillary; Asymptotics; Cauch-y distribution; Conditional inference; Confidence limits; Curved exponential family; Fisher information; Likelihood ratio; Location parameter; Statistical curvature. 1. INTRODUCTION In 1934, Sir Ronald Fisher's work on likelihood reached its peak. He had earlier advocated the maximum likelihood estimator as a statistic with least large sample information loss, and had computed the approximate loss. Now, in 1934, Fisher showed that in certain special cases, namely the location and scale models, all of the informationin the sample is recoverable by using an appropriately conditioned sampling distribution for the maximum likelihood estimator. This marks the beginning of exact conditional inference based on exact ancillary statistics, although the notion of ancillary statistics had appeared in Fisher's 1925 paper on statistical estimation. Beyond the explicit details of exact conditional distributions for special cases, the 1934 paper contains on p. 300 the following intriguing claim about the general case WVhenthese [log likelihood] functions are differentiable successive portions of the [information] loss may be recovered by using as ancillary statistics, in addition to the maximum likelihood estimate, the second and higher differential coefficients at the maximum. To this may be coupled an earlier statement (Fisher, 1925, p. 724) The function of the ancillary statistic is analogous to providing a true, in place of an approximate, weight for the value of the estimate. There are no direct calculations by Fisher to clarify the above remarks, other than calculations of information loss. But one may infer that approximate conditional inference based on the maximum likelihood estimate is claimed to be possible using observed properties This content downloaded from 165.91.114.21 on Fri, 12 Feb 2016 22:02:21 UTC All use subject to JSTOR Terms and Conditions 458 BRADLEY EFRON AND DAVID V. HINKLEY of the likelihood function. To be specific, if we take for granted that inferenceis accomplished by attaching a standard error to the maximum likelihood estimate, then Fisher's remarks suggest that we use a conditional variance approximation based on the observed second derivative of the log likelihood function, as opposed to the usual unconditional variance approximation, the reciprocal of the Fisher information. Our main topics in this paper are (i) the appropriatenessand easy calculation of such a conditional variance approximation and (ii) the ramificationsof this for statistical inference in the single parameter case. We begin with a simple illustrative example borrowedfrom Cox (1958). An experiment is conducted to measure a constant 0. Independent unbiased measurementsy of 0 can be made with either of two instruments, both of which measure with normal error: instrument k produces independent errors with a N(O,a2) distribution (k = 0, 1), where u2 and U2 are known and unequal. When a measurement y is obtained, a record is also kept of the instru- ment used, so that after a series of n measurementsthe experimental results are of the form (a1,YO) ..., (a,nYn), where a1 = k if y. is obtained using instrument k. The choice between instruments for the jth measurement is made at random by the toss of a fair coin, pr(a, = 0) = pr(aj = 1) = i. Throughout this paper, x will denote the entire set of experimental results available to the statistician, in this case (al, yl), ..., (an)Yn) The log likelihood function 1,9(x),1,9 for short, is the log of the density function, thought of as a function of 0. In this example n1n 19(x)= const -log Oa,- (yj-)0)2/ao (121) j=1 2j1aj from which we obtain the maximum likelihood estimator as the weighted mean a= ( YjIUaj)(Z 1/2o)-1. If we denote first and second derivatives of 1,9(x)with respect to 0 by 14(x)and 14(x),4, and i, for short, then the total Fisher information for this experiment is -f = var{4,(x)} = Et-1 (x)} = 1n(j/u02+ I/2). Standardtheory shows that &isasymptotically normally distributed with mean 0 and variance var (&) 1/.f0. (1.2) In this particular example X, does not depend on 0, so that the variance approximation (1.2) is known. If this were not so we would use one of the two approximations (Cox & Hinkley, 1974, p. 302) 1>IA lI/I(x), (1.3) where I(x) =-4o = [ 2x] 0-X(x) The quantity I(x) is aptly called the observed Fisher information by some writers, as distinguished from f0, the expected Fisher information. This last name is useful even though E(fo) * f0 in general. In the example above I(x) = a/al + (n-a)/ o, where a = z a>,the number of times instrument 1 was used. This content downloaded from 165.91.114.21 on Fri, 12 Feb 2016 22:02:21 UTC All use subject to JSTOR Terms and Conditions Observedversus expectedFisher information 459 Approximation (1.2), one over the expected Fisher information, would presumably never be applied in practice, because after the experiment is carriedout it is known that instrument 1 was used a times and that instrument 0 was used n - a times. With the ancillary statistic a fixed at its observed value, 0 is normally distributed wvithmean 0 and variance var (Oa) = {a/a2 +(n-a)/ao}-1 (1.4) not (1.2). But now notice that, whereas (1.2) involves an average property of the likelihood, the conditional variance (1.4) is a correspondingproperty of the observed likelihood: (1.4) is equal to the reciprocal of the observed Fisher information I(x). It is clear here that the conditional variance var (OI a) is more meaningful than var (0) in assessing the precisionof the calculated value 6 as an estimator of 0, and that the two variances may be quite different in extreme situations. This example is misleadingly neat in that var(Ola) exactly equals 1/I(x). Nevertheless, a version of this relationshipapplies, as an approximation,to general one parameter estimation problems. A central topic of this paper is the accuracy of the approximation var (8Ja) 1/I(x), (1.5) where a is an ancillary or approximately ancillary statistic which affects the precision of 0 as an estimator of 0. To a first approximation, a will be equivalent to I(x) itself. It is exactly so in Cox's example. The approximation (1.5) was suggested, never too explicitly, by Fisher in his fundamental papers on ancillarity and estimation. In complicated situations, such as that considered by Cox (1958), it is a good deal easier to compute I(x) than 4. There are also philosophical advantages to (1.5). It is 'closer to the data' than 1/.If, and tends to agree more closely with Bayesian and fiducial analyses. In Cox's example of the two measuring instruments, for instance, an improperuniform prior for 0 on (-oo, oo)gives var (6 Ix) = l1/I(x), in agreement with (1.5). To demonstrate that (1.5) has validity in more realistic contexts, consider the estimation of the centre 6 of a standard Cauclhytranslation family. For random samples of size n the Fisher information is X, = in. When n = 20, then 0 has approximate variance 0-1, in accordance with (1.2); the exact variance is about 0-115 according to Efron (1975, p. 1210). In a Monte Carlo experiment 14,048 Cauchy samples of size 20, with 6 = 0, were obtained, and Fig.
Details
-
File Typepdf
-
Upload Time-
-
Content LanguagesEnglish
-
Upload UserAnonymous/Not logged-in
-
File Pages27 Page
-
File Size-