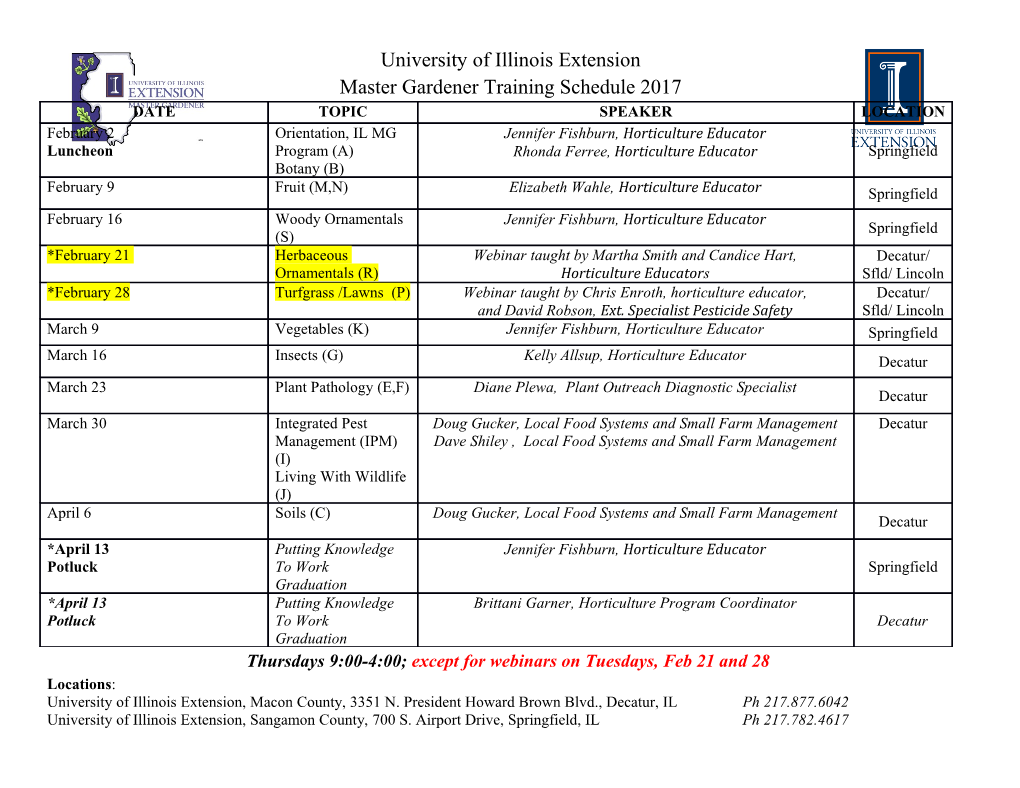
JAISCR, 2017, Vol. 7, No. 3, pp. 171 – 181 10.1515/jaiscr-2017-0012 MIDACO PARALLELIZATION SCALABILITY ON 200 MINLP BENCHMARKS Martin Schlueter and Masaharu Munetomo Information Initiative Center, Hokkaido University Sapporo, Sapporo 060-0811, Japan Submitted: 16th September 2016; accepted: 15th November 2016 Abstract This contribution presents a numerical evaluation of the impact of parallelization on the performance of an evolutionary algorithm for mixed-integer nonlinear programming (MINLP). On a set of 200 MINLP benchmarks the performance of the MIDACO solver is assessed with gradually increasing parallelization factor from one to three hundred. The results demonstrate that the efficiency of the algorithm can be significantly improved by parallelized function evaluation. Furthermore, the results indicate that the scale-up be- haviour on the efficiency resembles a linear nature, which implies that this approach will even be promising for very large parallelization factors. The presented research is espe- cially relevant to CPU-time consuming real-world applications, where only a low number of serial processed function evaluation can be calculated in reasonable time. Keywords: MINLP, optimization, MIDACO, parallelization 1 Introduction This contribution is an extended version of the Minimize f (x,y)(x R, y Z), ∈ ∈ numerical study recently presented in Schlueter and Munetomo [23]. This study discusses the opti- subject to: gi(x,y)=0, i = 1,...,me mization of problems known as mixed-integer non- g (x,y) 0, i = m + 1,...,m, (1) linear programs (MINLP). The considered MINLP i ≥ e is stated mathematically in (1), where f (x,y) de- notes the objective function to be minimized. and bounds: xl x xu (xl, xu R) ≤ ≤ ∈ In (1), the equality constraints are given by yl y yu (yl, yu N). g1,...,me(x,y) and the inequality constraints are given ≤ ≤ ∈ by g (x,y). The solution vector x contains me+1,...,m Optimization of MINLP problems is a young the continuous decision variables and the solution and growing field in the evolutionary computing vector y contains the discrete decision variables community, see e.g. Babu and Angira [1], Cardoso (also called integers). Furthermore, some box con- [2], Costa and Oliveira [3], Deep et al. [4], Glover straints as x ,y (lower bounds) and x ,y (upper l l u u [6], Liang et al. [11], Mohammed [12], Munawar bounds) for the decision variables x and y are con- [13], Wasanapradit et al. [28], Young et al. [30], sidered in (1). Yiqing et al. [29] or Yue et al. [32]. One advantage of evolutionary algorithms is their robustness to- wards the analytical properties of the objective and constraint functions. Therefore, in above MINLP Unauthenticated Download Date | 1/27/18 3:25 PM 172 Martin Schlueter and Masaharu Munetomo (1) the functions f (x,y) and g(x,y) are considered mization problems the algorithm applies the Oracle as general black-box functions without any require- Penalty Method which was introduced in Schlueter ments, such as differentiability or smoothness. An- and Gerdts [18]. While the MIDACO algorithm is other advantage of evolutionary algorithms is their conceptually designed as general black-box solver, capability to (greatly) benefit from parallelization, it has proven its effectiveness especially on chal- see for example Du et al. [14], Laessig and Sud- lenging interplanetary space trajectory design prob- holt [10], Gupta [10], Sakuray [16], Sudholt [27] lems (see Schlueter [21]), where it holds several or Yingyong et al [31]. One of the most popular best known record solutions on benchmarks pro- strategies to use parallelization in evolutionary al- vided by the European Space Agency [5]. It is fur- gorithms is the distributed computing of the prob- thermore the first algorithm that was able to suc- lem function evaluations. This strategy is some- cessfully solve interplanetary trajectory problems times denoted as co-evaluation. formulated as mixed-integer problems, where the Presented here numerical study investigates the sequence of fly-by planets was considered as integer impact of a varying co-evaluation factor on the optimization variables (see Schlueter et. al. [20]). performance of an evolutionary optimization algo- rithm on a set of 200 MINLP benchmarks (see 2.1 Parallelization Approach Schittkowski [24]), which mostly originate from the The parallelization approach considered here well-known GAMS MINLPlib library [9]. Con- aims on distributing the problem function evalua- sidered benchmark instances consist of up to 205 tion. This approach is sometimes referred to as co- variables and 283 constraints, including up to 100 evaluation. The MIDACO solver optimization soft- equality constraints (see the Appendix for details ware easily enables this kind of parallelization due on the benchmark instances). This paper focuses to its reverse communication architecture. Reverse on investigation and measurement of the efficiency communication means here that the call of the ob- of parallelized function evaluation calls on the al- jective and constraint functions happens outside and gorithmic performance. As numerical solver, the independently of the MIDACO source code. MIDACO optimization software is used, which is based on an evolutionary algorithm especially de- Within a single reverse communication loop, veloped for mixed-integer problems and capable MIDACO does accept and returns an arbitrary large of seamless parallelization of function evaluation number of P iterates x (also called ”solution can- calls. didates” or ”individuals”) at once. Hence, those P iterates can be evaluated in parallel, outside and in- This paper is structured as follows: In Section 2 dependently from the MIDACO source code. This a brief overview on the MIDACO algorithm is given idea of passing a block of P iterates at once within with an emphasis on its parallelization approach. In one reverse communication step to the optimization Section 3 the numerical results of 300 individual algorithm was originally introduced by the code test runs on the set of 200 MINLP benchmarks are NLPQLP by Schittkowski [25]. illustrated and discussed. In Section 4 a summary and general conclusions are presented. A compre- hensive Appendix lists detailed individual informa- tion on all considered benchmarks. 2 MIDACO Algorithm MIDACO stands for Mixed Integer Distributed Ant Colony Optimization. The evolutionary al- gorithm within MIDACO is based on the ant Figure 1. Reverse communication loop with block colony optimization metaheuristic for continuous of P iterates (solution candidates). search domains proposed by Socha and Dorigo Figure 1 illustrates the reverse communication [26] and was extended to mixed-integer domains loop where a block of P iterates is evaluated regard- by Schlueter et al. in [17]. For constrained opti- Unauthenticated Download Date | 1/27/18 3:25 PM Martin Schlueter and Masaharu Munetomo MIDACO PARALLELIZATION SCALABILITY ON . 173 (1) the functions f (x,y) and g(x,y) are considered mization problems the algorithm applies the Oracle ing their objective function f (x) and constraints as general black-box functions without any require- Penalty Method which was introduced in Schlueter g(x) and then passed to the MIDACO optimization f (x∗,y∗) ments, such as differentiability or smoothness. An- and Gerdts [18]. While the MIDACO algorithm is algorithm, which then again returns a new block of f (xˆ,yˆ) f (x∗,y∗)+∥ ∥, ≤ 100 other advantage of evolutionary algorithms is their conceptually designed as general black-box solver, P iterates to be evaluated. capability to (greatly) benefit from parallelization, it has proven its effectiveness especially on chal- This concept allows an independent and user (2) g(xˆ,yˆ)i=1,...,m 0.01, see for example Du et al. [14], Laessig and Sud- lenging interplanetary space trajectory design prob- controlled distributed computing of the objective ∥ e ∥≤ holt [10], Gupta [10], Sakuray [16], Sudholt [27] lems (see Schlueter [21]), where it holds several and constraint function. In other words: The or Yingyong et al [31]. One of the most popular best known record solutions on benchmarks pro- g(xˆ,yˆ) 0.01. displayed parallelization option is valid for any i=me+1,...,m ≥− strategies to use parallelization in evolutionary al- vided by the European Space Agency [5]. It is fur- language and any CPU architecture. This in- gorithms is the distributed computing of the prob- thermore the first algorithm that was able to suc- cludes in particular multi-core PC’s, PC-Clusters Equation (2) implies that a test run was con- lem function evaluations. This strategy is some- cessfully solve interplanetary trajectory problems and GPGPU (General Purpose Graphical Process- sidered successful, if the approximative solution times denoted as co-evaluation. formulated as mixed-integer problems, where the ing Unit) based computation. In case of MIDACO, (xˆ,yˆ) reached by MIDACO was as close as 1% to Presented here numerical study investigates the sequence of fly-by planets was considered as integer the parallelization factor P can furthermore be any the global optimal solution objective function value impact of a varying co-evaluation factor on the optimization variables (see Schlueter et. al. [20]). arbitrary large integer value, enabling a seamless f (x∗,y∗) while satisfying all constraints with a pre- performance of an evolutionary optimization algo- and massive parallelization. As this parallelization cision of at least 0.01. Note that the tolerance rithm on a set of 200 MINLP benchmarks (see 2.1 Parallelization Approach approach aims on distributing the function evalu- of 0.01 for the constraint violation is chosen here rather moderate. This is due to the relatively large Schittkowski [24]), which mostly originate from the The parallelization approach considered here ation calls, it is intended for problems where the number of (up to one hundred) equality constraints well-known GAMS MINLPlib library [9]. Con- aims on distributing the problem function evalua- function evaluation are numerically expensive to in several benchmark instances (see Appendix). For sidered benchmark instances consist of up to 205 tion.
Details
-
File Typepdf
-
Upload Time-
-
Content LanguagesEnglish
-
Upload UserAnonymous/Not logged-in
-
File Pages11 Page
-
File Size-