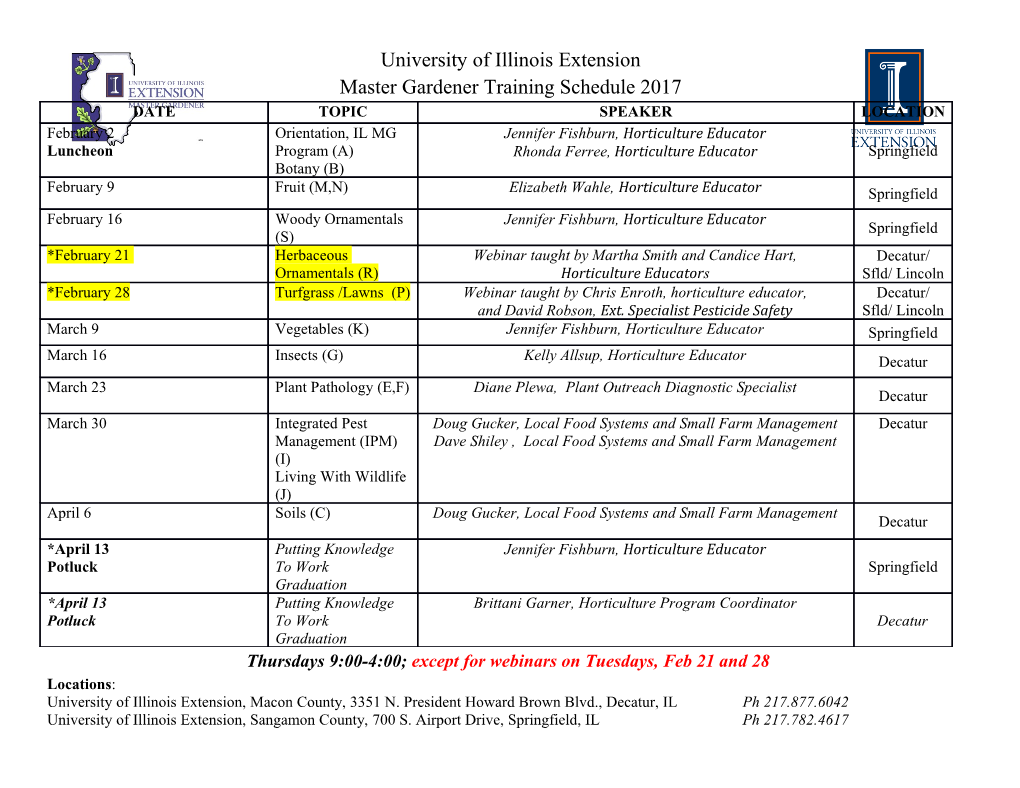
Manifold-Adaptive Dimension Estimation Amir massoud Farahmand [email protected] Csaba Szepesv¶ari [email protected] Department of Computing Science, University of Alberta, Edmonton, AB T6G 2E8 Canada Jean-Yves Audibert [email protected] CERTIS - Ecole des Ponts, 19, rue Alfred Nobel - Cit¶eDescartes, 77455 Marne-la-Vall¶eeFrance Abstract larity is present in the data. Intuitively, learning should be easier when One such regularity that has attracted much attention the data points lie on a low-dimensional sub- lately is when the samples lie in a low-dimensional sub- manifold of the input space. Recently there manifold of the possibly high-dimensional input space. has been a growing interest in algorithms Consider for example the case when the data points that aim to exploit such geometrical prop- are images taken of a scene or object, from di®erent erties of the data. Oftentimes these algo- angles. Although the images may contain millions of rithms require estimating the dimension of pixels, they all lie on a manifold of low dimensionality, the manifold ¯rst. In this paper we propose such as 3. Another example is when the input data is an algorithm for dimension estimation and enriched by adding a huge number of feature compo- study its ¯nite-sample behaviour. The algo- nents computed from the original input components in rithm estimates the dimension locally around the hope that these additional features will help some the data points using nearest neighbor tech- learning algorithm (generalized linear models or the niques and then combines these local esti- \kernel trick" implement this idea). mates. We show that the rate of conver- Manifold learning research aims at ¯nding algorithms gence of the resulting estimate is indepen- that require less data (i.e., are more data e±cient) dent of the dimension of the input space and when the data happens to be supported on a low- hence the algorithm is \manifold-adaptive". dimensional submanifold of the input-space. We call a Thus, when the manifold supporting the data learning algorithm manifold-adaptive when its sample- is low dimensional, the algorithm can be ex- complexity depends on the intrinsic dimension of the ponentially more e±cient than its counter- manifold only.1 A classical problem in pattern recog- parts that are not exploiting this property. nition is the estimation of the dimension of the data Our computer experiments con¯rm the ob- manifold. Dimension estimation is interesting on its tained theoretical results. own, but it is also very useful as the estimate can be fed into manifold-aware supervised learning algorithms that need to know the dimension to work e±ciently 1. Introduction (e.g., Hein 2006; Gine and Koltchinskii 2007). The curse of dimensionality in machine learning refers In this paper we propose an algorithm for estimating to the tendency of learning algorithms working in high- the unknown dimension of a manifold from samples dimensional spaces to use resources (time, space, sam- and prove that it is manifold-adaptive. The new algo- ples) that scale exponentially with the dimensionality rithm belongs to the family of nearest-neighbor meth- of the space. Since most practical problems involve ods. Such methods have been considered since the late high-dimensional spaces, it is of uttermost importance 70s. Pettis et al. (1979) suggested to average distances to identify algorithms that are capable of avoiding this to k-nearest neighbors for various values of k and use exponential blow-up, exploiting when additional regu- the obtained values to ¯nd the dimension using an iter- th Appearing in Proceedings of the 24 International Confer- 1Of course, the sample-complexity may and will typi- ence on Machine Learning, Corvallis, OR, 2007. Copyright cally depend on the properties of the manifold and thus 2007 by the author(s)/owner(s). the embedding. Manifold-Adaptive Dimension Estimation ative method.2 Another more recent method is due to the ball B(x; r), while the other approach is to cal- Levina and Bickel (2005) who suggested an algorithm culate the radius of the smallest x-centered ball that based on a Poisson approximation to the process ob- encloses some ¯xed number of points. Either way, one tained by counting the number of neighbors of a point ends up with an estimate of both ln(P (Xi 2 B(x; r))) in its neighborhood. In a somewhat heuristic man- and ln(r). Taking multiple measurements, we may get ner they argued for the asymptotic consistency of this an estimate of d by ¯tting a line through these mea- method. Grassberger and Procaccia (1983) suggested surements, by treating ´ as a constant. Because ´ to estimate the dimension based on the so-called cor- cannot be considered constant when r is large (due to relation dimension, while Hein and Audibert (2005) the uneven sampling distribution or the curvature of suggested a method based on the asymptotics of a the manifold), one should ideally work at small scales smoothed version of the correlation dimension esti- (small r). On the other hand, when r is too small mate. Despite the large number of works and long then the measurements' variance will be high. A good history, to our best knowledge no previous rigorous estimator must thus ¯nd a good balance between the theoretical work has been done on the ¯nite-sample bias and the variance, making the estimation of the behavior of dimension-estimation algorithms, let alone intrinsic dimension a non-trivial problem. their manifold adaptivity. In this paper we study an algorithm in which we ¯x the \scale" by ¯xing the number of neighbors, k: the 2. Algorithm dimension is estimated from the distance to the kth nearest neighbor. In the other approach, i.e., when a The core component of our algorithm estimates the di- scale h = h is selected, the typical requirement is that mensionality of the manifold in a small neighborhood n hd n ! 1, or h = ­(n¡1=d). Given that d is unknown of a selected point. This point is then varied and re- n n this suggests to choose h = Cn¡1=D. This choice, sults of the local estimates are combined to give the n however, is too conservative and would not lead to a ¯nal estimate. dimension adaptive procedure.3 On the other hand, The local estimate is constructed as follows: Collect for the consistency of k-nearest neighbor procedures the observed data points into Dn = [X1;:::;Xn]. We one typically requires only kn=n ! 0 and kn ! 1 shall assume that Xi is an i.i.d. sample that comes (these conditions are independent of d). Therefore we from a distribution supported on the manifold M. De- prefer nearest-neighbor based techniques for this task. ¯ne ´(x; r) by In order to be more speci¯c about the method, let (k) d X (x) be the reordering of the data such that P (Xi 2 B(x; r)) = ´(x; r)r ; (1) jjX(k)(x) ¡ xjj · jjX(k+1)(x) ¡ xjj holds for k = or 1; 2; : : : ; n¡1 (ties are broken randomly). Here k¢k de- notes the `2-norm of RD. Hence, X(1)(x) is the nearest (2) ln(P (Xi 2 B(x; r))) = ln(´(x; r)) + d ln(r); (2) neighbor of x in Dn, X is the 2nd nearest neighbor, etc. Letr ^(k)(x) = kX(k)(x)¡xk be the distance to the where B(x; r) ½ RD is a ball around the point x 2 M kth nearest neighbor of x. In our theoretical analysis, in the Euclidean space RD. Our main assumption in for the sake of proofs simplicity, we use the follow- the paper will be that in a small neighborhood of 0 ing simple estimation method: Take k > 2. Denoting the function ´(x; ¢) is slowly varying (the assumptions ´(x; r) ¼ ´0, from (2) we have on ´ will be made precise later). This is obviously (k) satis¯ed in the commonly studied simple case when the ln(k=n) ¼ ln(´0) + d ln(^r (x)); (dk=2e) distribution of the data on the manifold is uniform and ln(k=(2n)) ¼ ln(´0) + d ln(^r (x)); ¡ ¢ the manifold satis¯es standard regularity assumption (k) such as those considered by Hein et al. (2006). since if n is big, P X0 2 B(x; r^ (x)) should be close to k=n. Reordering the above equations for d, we get There are two ways of using Equation (2) for estimat- ^ ln 2 ing the dimension d. Both rely on the observation that d(x) = ln(^r(k)(x)=r^(dk=2e)(x)) : (3) this equation is linear in d. One approach is to ¯x a radius and count the number of data points within When a center is selected from data, this point is naturally removed when calculating the point's near- 2Due to the lack of space, we cannot attempt to give a est neighbors. With a slight abuse of notation, the full review of existing work on dimension estimation. The interested reader may consult the papers of Kegl (2002) 3Of course, other options, such as using splitting or and Hein and Audibert (2005) which contain many further cross-validation to select h are also possible. We leave it pointers. for future work to study such algorithms. Manifold-Adaptive Dimension Estimation estimate when selecting center Xi is also denoted by bility at least 1 ¡ ± ^ d(Xi). Ã µ ¶ 1 r ! d ^ k ln(4=±) When used at a single random data point, the variance jd(X1) ¡ dj · E [C(X1)] d B + ; of the estimate will be high and due to k ¿ n the n k available data is used in a highly ine±cient manner.
Details
-
File Typepdf
-
Upload Time-
-
Content LanguagesEnglish
-
Upload UserAnonymous/Not logged-in
-
File Pages8 Page
-
File Size-