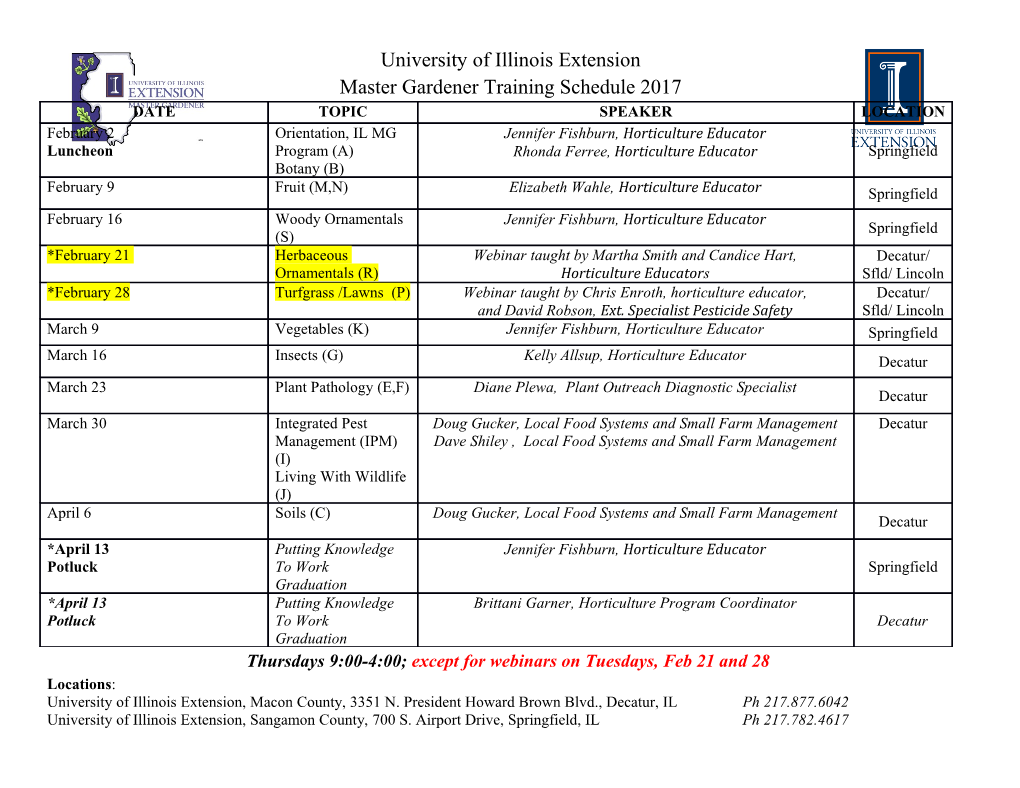
Economic experiments and inference Norbert Hirschauer (a), Sven Grüner (b), Oliver Mußhoff (c), and Claudia Becker (d) Abstract: Replication crisis and debates about p-values have raised doubts about what we can statisti- cally infer from research findings, both in experimental and observational studies. The goal of this paper is to provide an adequate differentiation of experimental studies that enables researchers to bet- ter understand which inferences can and – perhaps more important – cannot be made from particular designs. Keywords: Economic experiments, ceteris paribus, confounders, control, inference, randomization, random sampling, superpopulation JEL: B41, C18, C90 (a) Martin Luther University Halle-Wittenberg, Institute of Agricultural and Nutritional Sciences, Chair of Agribusiness Management, Karl-Freiherr-von-Fritsch-Str. 4, 06120 Halle (Saale), Germany. Corresponding author and presenter ([email protected]) (b) Martin Luther University Halle-Wittenberg, Institute of Agricultural and Nutritional Sciences, Chair of Agribusiness Management, Karl-Freiherr-von-Fritsch-Str. 4, 06120 Halle (Saale), Germany ([email protected]) (c) Georg August University Göttingen, Department for Agricultural Economics and Rural Develop- ment, Farm Management, Platz der Göttinger Sieben 5, 37073 Göttingen, Germany ([email protected]) (d) Martin Luther University Halle-Wittenberg, Institute of Business Studies, Chair of Statistics, Große Steinstraße 73, 06099 Halle (Saale), Germany. ([email protected]) 1 Economic experiments and inference Abstract: Replication crisis and debates about p-values have raised doubts about what we can statisti- cally infer from research findings, both in experimental and observational studies. The goal of this paper is to provide an adequate differentiation of experimental designs that enables researchers to bet- ter understand which inferences can and – perhaps more important –cannot be made from particular designs. Keywords: Economic experiments, ceteris paribus, confounders, control, inference, randomization, random sampling, superpopulation JEL: B41, C18, C90 1 Introduction Starting with CHAMBERLIN (1948), SAUERMANN and SELTEN (1959), HOGGATT (1959), SIEGEL and FOURAKER (1960), and SMITH (1962), economists have increasingly adopted experimental designs in empirical research over the last decades. Their motivation to do so was to obtain – compared to obser- vational studies – more reliable information about the causalities that govern the behavior of economic agents. Unfortunately, it seems that in the process of adopting the experimental method, no clear-cut and harmonized definition has emerged of what constitutes an economic experiment. Some scholars seem to use randomization as the defining quality of an experiment and equate “experiments” with “randomized controlled trials” (ATHEY and IMBEN 2017). Others include non-randomized designs into the definition as long as behavioral data are generated through a treatment manipulation (HARRISON and LIST (2004). One might speculate that economists tend to conceptually stretch the term “experi- ment” because the seemingly attractive label suggests that they have finally adopted “trustworthy” research methods that are comparable to those in the natural sciences. Whatever the reason, confusion regarding the often fundamentally different types of research designs that are labeled as experiments entails the risk of inferential errors. In general, the inferences that can be made from controlled experiments based on the ceteris paribus approach where “everything else but the item under investigation is held constant” (SAMUELSON and NORDHAUS 1985: 8) are different from those that can be made from observational studies. The former rely on the research design to ex ante ensure ceteris paribus conditions that facilitate the identification of the causal effects of a treatment. Observational studies, in contrast, rely on an ex post control of confounders through statistical modeling that, despite attempts to move from correlation to causation, does not provide a way of ascertaining causal relationships which is as reliable as a strong ex ante research design (ATHEY and IMBEN 2017). But even within experimental approaches, different designs facilitate different inferences. In this paper, we address the question of scientific and statistical induction and, more particularly, the role of the p-value for making inferences beyond the confines of a particular experimental study. What we aim at is an adequate differentiation of experimental designs that enables researchers to better un- derstand which inferences can and – perhaps more important –cannot be made from particular designs. 2 Experiments aimed at identifying causal treatment effects A study is generally considered to be experimental when a researcher deliberately introduces a well- defined treatment (also known as intervention) into a controlled environment. Identifying the effects of 2 the treatment on the units (subjects) under study requires a comparison; often a no-treatment group of subjects is compared to a with-treatment group. Two different designs are used to ensure control and thus ceteris paribus conditions: (i) Randomized controlled trials rely on a between-subject design and randomization to generate equivalence between compared groups; i.e. we randomly assign subjects to treatments to ensure that known and unknown confounders are balanced across treatment groups (sta- tistical independence). (ii) Non-randomized controlled trials, in contrast, rely on a within-subject de- sign and before-and-after comparisons; i.e. we try to hold everything but the treatment constant over time1 and compare the before-and-after-treatment outcomes for each subject of the experimental popu- lation.2 Table 1 sketches out experimental designs based on treatment comparisons. The label “experiment” is first of all used for studies that, instead of using survey data or pre-existing observational data, are based on a deliberate intervention and a design-based control over confounders. The persuasiveness of causal claims depends on the credibility of the alleged control. Comparing randomized treatment groups is generally held to be a more convincing device to identify causal relationships than before- and-after treatment comparisons (CHARNESS et al. 2012).3 This is due to the fact that randomization balances known and unknown confounders across treatment groups and thus ensures statistical inde- pendence.4 In contrast, efforts to hold everything else but the treatment constant over time in before- and-after comparisons are limited by the researcher’s capacity to identify and fix confounders. Table 1: Design-based control for confounding influences in experimental treatment comparisons Randomized-treatment-group com- Before-and-after-treatment compari- parison (between-subject design) son (within-subject design) Intervention by researcher Randomized controlled experi- Non-randomized controlled experi- (experimental study) ments/trials ments/trials No intervention by researcher “Natural experiments” - (observational study) Due to the particular credibility of randomization as a means to establish control over confounders, the use of the term “experiment” – accompanied by the label “natural” – has even been extended to obser- vational settings (see gray print in Table 1) where, instead of a treatment manipulation by a researcher, the socio-economic or natural environment has randomly assigned “treatments” among some set of units. Regarding this terminology, DUNNING (2013: 16) notes “that the label ‘natural experiment’ is perhaps unfortunate. […], the social and political forces that give rise to as-if random assignment of interventions are not generally ‘natural’ in any ordinary sense of that term. […], natural experiments 1 Trying to hold “everything” but the treatment constant over time can be difficult. A particular threat to causal inference arises when subjects’ properties change through treatment exposure. In other words, sequentially ex- posing subjects to multiple treatments may cause order effects that violate the ceteris paribus condition (CHARNESS et al. 2012). 2 The term “non-randomized controlled trial” is also used for between-subject designs when the technique of assigning subjects to treatments, e.g. alternate assignment, is not truly random but claimed to be as good as a random. 3 CZIBOR et al. (2019) emphasize that within-subject designs also have advantages. Besides the fact that they can more effectively make use of small experimental populations, they facilitate the identification of higher moments of the distribution. Whereas between-subject designs are limited to estimating average treatment effects, within- subject designs enable researchers to look at quantiles and assess heterogeneous treatment effects among sub- jects. 4 DUFLO et al. (2007) note that randomization only achieves that confounders are balanced across treatments “in expectation;” i.e. balance is only ensured if we have a “sufficiently” large experimental population to start with (law of large numbers). In the case of a very small experimental population, an observed difference between two randomized treatment groups could easily be contaminated by unbalanced confounders. 3 are observational studies, not true experiments, again, because they lack an experimental manipula- tion. In sum, natural experiments are neither natural nor experiments.”5 3 Inferences in experimental
Details
-
File Typepdf
-
Upload Time-
-
Content LanguagesEnglish
-
Upload UserAnonymous/Not logged-in
-
File Pages10 Page
-
File Size-