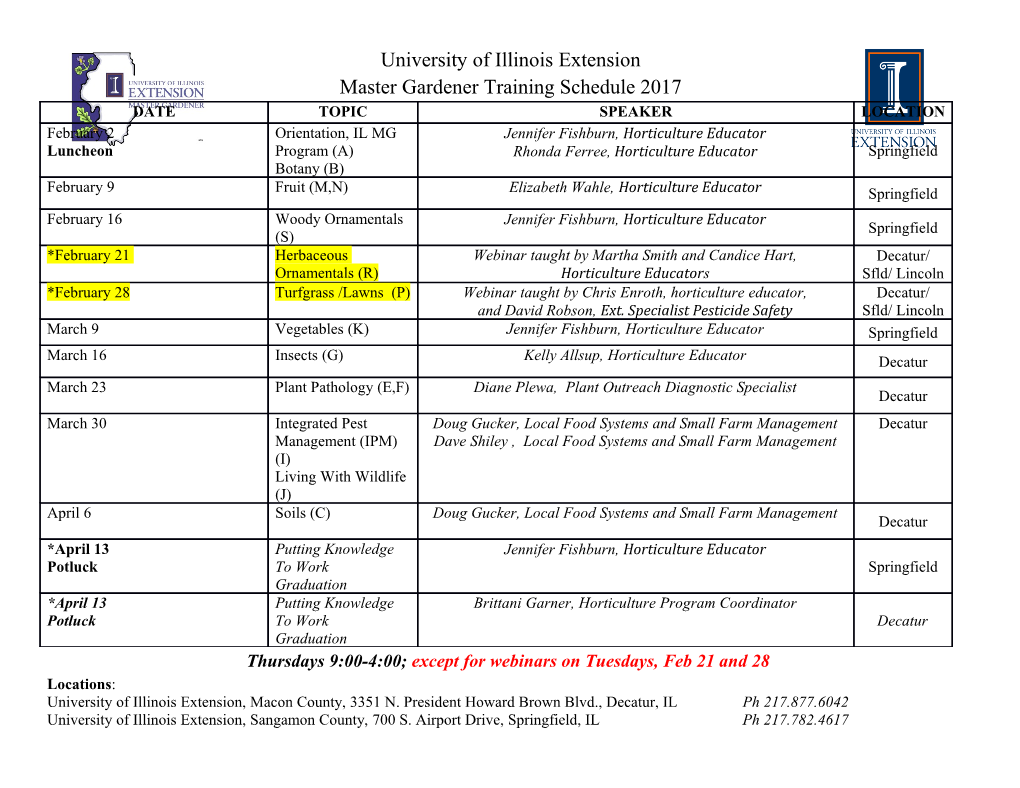
Preprocessing and Descriptor Features for Facial Micro-Expression Recognition Chris House and Rachel Meyer Department of Electrical Engineering, Stanford University {chouse12, r3m3y3r}@stanford.edu June 5, 2015 Abstract sions that reveal true feelings even when someone is attempting to conceal their emotions. These expres- Facial micro-expressions contain signicant informa- sions are extremely dicult to detect due to their tion about how people feel, even when they are at- short time scales and subtlety. Micro-expressions tempting to conceal their emotions. Previously, very were rst reported in psychological literature in the little research has been done to detect and recognize 1960's and have been studied since then[1, 2]. Re- micro-expressions using computer vision methods. cently, a few research groups have attempted micro- In this paper, detection and classication of micro- expression detection and recognition using computer expressions from the Spontaneous Micro-Expression vision techniques. Trained humans can recognize database were implemented, following preprocessing micro-expressions accurately about 47% of the time and cropping of raw images using Haar features, us- [3], but perhaps computer vision methods can achieve ing local binary patterns on three orthogonal planes higher accuracy. The applications of technology (LBP-TOP) and local gray code patterns on three that can successfully detect and recognize micro- orthogonal planes (LGCP-TOP) as descriptors and expressions are varied. It would be valuable in law support vector machines (SVMs) as detection and enforcement interrogations to detect deceit, in mar- recognition algorithms. Results show accuracy com- keting to detect how humans respond to advertising, parable to other work. and in general psychology and articial intelligence research to study human emotion. 1 Introduction 2 Background Facial expressions contain signicant information about how a person feels. Humans are adept at recog- Previous work in micro-expression analysis has taken nizing macro-expressions that occur for time periods mostly a psychological analysis form. The psychol- on the order of a few seconds, but humans are able to ogists Haggard and Isaacs rst proposed the idea manipulate these expressions to hide their true emo- of micro-expressions, or short, involuntary reactions tions. Micro-expressions, on the other hand, are short that are immediately retracted in an attempt at sup- (lasting less than half a second), involuntary expres- pression, in a 1966 study [4]. Paul Ekman, noted 1 for his work in facial expression analysis, published A number of dierent approaches have been pro- his seminal work in 1968 regarding Nonverbal Leak- posed and implemented for detection and recogni- age and Clues to Deception, in which he detailed tion of micro-expressions [9]. Some groups have used his ndings in detecting deception. Ekman used mi- Gabor features and algorithms such as SVM, Ad- croexpression analysis in conjunction with his previ- aboost, and other variations of those to recognize ous work regarding emotion classication based o micro-expressions [10]. Others have divided the face of facial patterns to further understand why hu- into sub-regions and analyzed facial strain in these re- mans lie and how they display deceit nonverbally gions for detection [11], or used 3D gradient descrip- [5]. In 2002, John Gottman, a famous psychologist tors [12]. Temporal interpolation models and multi- for his work in predicting divorce rate based o of ple kernel learning have also been explored [3]. How- a couples' interaction, used micro-expression analy- ever, the most common method by far has been Local sis from short video clips to enhance his understand- Binary Patterns on Three Orthogonal Planes (LBP- ing of couple interaction and his divorce prediction TOP) combined with various learning techniques for ability [6]. All of these psychological experts cer- classication [7, 8]. In the last several years, several tainly gained an ability to detect and recognize micro- groups have achieved detection accuracies on the or- expressions. However, while all of this work helped der of 60-70% (with 50% as the baseline chance detec- increase understanding of micro-expressions from a tion) and recognition accuracy between 3 classes of psychological standpoint, none of the work sought emotions on the order of 50% (3 classes, so 33% as the to gain a generalized ability to detect or recognize baseline chance detection) [7]. Another newly devel- micro-expressions. Thus, in the last few years, sev- oped descriptor, Local Gray Code Patterns (LGCP), eral groups have attempted to create general com- has claims of improving upon the deciencies of the puter vision methods that can detect and recognize descriptors listed above, most notably the memory specic facial micro-expressions. Until recently, the requirements of Gabor features and the inability to biggest challenge in attempting to create a general handle scene illumination variance and noise for LBP method for micro-expression detection and recogni- [13]. tion had been a lack of useful data. However, in 2013, two databases were developed and released for pub- 2.1 Our Contributions lic use in research with micro-expressions: the SMIC database (Spontaneous Micro-Expression Database) Our project evaluates previous research by imple- and CASME II. These databases were developed un- menting similar descriptors and algorithms. Our der similar premises: test subjects were lmed using main contributions include a more thorough analysis high speed cameras while being shown various video of the results and implementing a newly developed clips that were intended to induce emotional reac- feature descriptor, LGCP-TOP. tions. The test subjects were also instructed to sup- press an outward reaction so as to properly induce micro-expressions [7, 8]. Other databases have also 3 Methods been constructed but these databases are generally less desirable for analysis since the micro-expressions For this project, we used the Spontaneous Micro- were obtained through posed expressions (test sub- Expression Database (SMIC). The database contains ject told to display facial expression corresponding to both raw images and preprocessed and cropped im- an emotion, but with low muscle intensity) or while ages. The raw images were cropped using Haar fea- the test subject was talking or moving their head tures to detect the subject's face. The descriptors (which makes computer vision analysis more dicult used were local binary patterns on three orthogo- as it essentially introduces noise) [7]. Other more nal planes (LBP-TOP) and local gray code patterns general facial expression databases include CK, MMI, on three orthogonal planes (LGCP-TOP). Support JAFFE, RUFACS, MULTI-PIE, and Oulu-CASIA. vector machines (SVMs) were used for detection and 2 recognition. are then classied using a classication and regres- sion tree (CART). The output of this function was a 3.1 SMIC Database bounding box for the given image. Using the bound- ing box, the image was then cropped (to a uniform The SMIC database, developed by the research group size) and then resized in order to allow for faster led by Xiaobai Li at University of Oulu, was created processing in obtaining the feature descriptors later specically for work with micro-expression recogni- on. In comparison to the preprocessed images in the tion. In the SMIC dataset, the test subjects' facial ex- SMIC database, our cropping method achieved a sim- pressions were lmed while watching 16 various movie ilar result. clips designed to induce strong emotions. Each sub- ject was instructed to try to conceal their reaction to each clip as much as possible, as the testers would at- tempt to guess their reactions to each clip afterwards. As an incentive for the test subjects, the testers added a penalty of having to complete a long, boring ques- tionnaire if they were to accurately guess the sub- Figure 1: Process outlining the cropping of the raw jects' emotions. In this way, the data obtained was images [3]. a more accurate representation of how humans pro- duce micro-expressions. The database contains 164 micro-expression video clips elicited from 16 partic- ipants. These micro-expressions were classied into 3.3 LBP-TOP positive, negative, and surprise categories. We uti- Choosing the features for micro-expressions is a dif- lized the high speed video (100 fps) data from the cult problem, as both spatial and temporal infor- dataset (other options included video sequences at mation should be encoded into them. Additionally, 25 fps and near-infrared images). Table 1 shows the micro-expressions are very subtle and last for a short composition of the dataset. period of time. The local binary patterns on three or- thogonal planes (LBP-TOP) descriptor was selected Table 1: Composition of the SMIC database. Note after an extensive survey of relevant literature [7, that these images are available both as raw images 8]. The local binary pattern (LBP) descriptor, from and cropped images. which LBP-TOP was derived, was developed in 1994 and is used commonly in computer vision applica- tions. Considering just one pixel in the image, a LBP is computed by comparing the pixel value with that of its neighbors, and then converting the binary string representing the local texture into a decimal num- ber. A feature vector can be formed by calculating 3.2 Preprocessing of Images a histogram of LBPs over an entire image. The LBP method is eective for describing 2D textures of static Using the raw images from the SMIC database, images, but to analyze time-dependent textures (i.e. we implemented the CascadeObjectDetectorSystem changing expressions in video), this model needs to be from the MATLAB computer vision toolbox. This extended. To do so, LBP histograms are calculated in object detector has several built-in object detectors, three orthogonal planes. For a video with time length including three face detectors (two using Haar fea- T, LBP are computed for the XY-, XT-, and YT- tures, one using LBP), eye detectors, a nose detector, planes.
Details
-
File Typepdf
-
Upload Time-
-
Content LanguagesEnglish
-
Upload UserAnonymous/Not logged-in
-
File Pages8 Page
-
File Size-