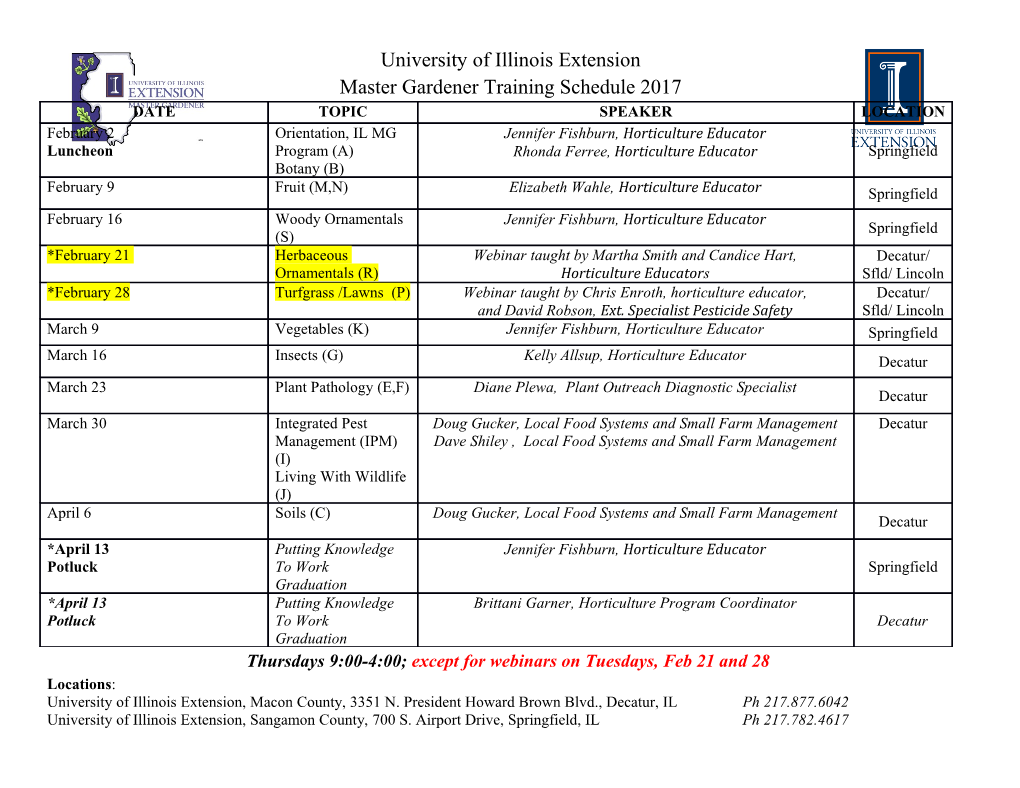
Combined Detection and Segmentation of Archeological Structures from LiDAR Data Using a Deep Learning Approach RESEARCH ARTICLE ALEXANDRE GUYOT MARC LENNON THIERRY LORHO LAURENCE HUBERT-MOY *Author affiliations can be found in the back matter of this article ABSTRACT CORRESPONDING AUTHOR: Alexandre Guyot Until recently, archeological prospection using LiDAR data was based mainly on expert- Laboratoire LETG – UMR 6554, based and time-consuming visual analyses. Currently, deep learning convolutional Université Rennes 2, FR neural networks (deep CNN) are showing potential for automatic detection of objects in alexandre.guyot@univ- many fields of application, including cultural heritage. However, these computer-vision rennes2.fr based algorithms remain strongly restricted by the large number of samples required to train models and the need to define target classes before using the models. Moreover, the methods used to date for archaeological prospection are limited to detecting KEYWORDS: objects and cannot (semi-)automatically characterize the structures of interest. In archaeological prospection; this study, we assess the contribution of deep learning methods for detecting and airborne laser system; transfer characterizing archeological structures by performing object segmentation using learning; object segmentation; remote sensing a deep CNN approach with transfer learning. The approach was applied to a terrain visualization image derived from airborne LiDAR data within a 200 km² area in Brittany, France. Our study reveals that the approach can accurately (semi-)automatically TO CITE THIS ARTICLE: Guyot, A, Lennon, M, Lorho, detect, delineate, and characterize topographic anomalies, and thus provides an T and Hubert-Moy, L. 2021. effective tool to inventory many archaeological structures. These results provide new Combined Detection and perspectives for large-scale archaeological mapping. Segmentation of Archeological Structures from LiDAR Data Using a Deep Learning Approach. Journal of Computer Applications in Archaeology, 4(1), pp. 1–19. DOI: https://doi. org/10.5334/jcaa.64 Guyot et al. Journal of Computer Applications in Archaeology DOI: 10.5334/jcaa.64 2 1. INTRODUCTION segmentation, which prevents them from being applied to complex structures. In recent years, deep learning The past decade has seen an increasing interest in remote- Convolutional Neural Networks (deep CNNs) have resulted sensing technologies and methods for monitoring in a new paradigm in image analysis and provided ground- cultural heritage. One of the most relevant changes is breaking results in image classification (Krizhevsky, the development of airborne light detection and ranging Sutskever and Hinton 2012) or object detection (Girshick (LiDAR) systems (ALS). With the ability to measure 2015). Deep CNNs are composed of multiple processing topography accurately and penetrate the canopy, layers that can learn representations of data with multiple ALS has been a key tool for important archaeological levels of abstraction (LeCun, Bengio and Hinton 2015). In discoveries and a better understanding of past human the context of LiDAR-based archaeological prospection, activities by analyzing the landscape (Bewley, Crutchley they were first applied in 2016 (Due Trier, Salberg and and Shell 2005; Chase et al. 2011; Evans et al. 2013; Holger Pilø 2016) to detect charcoal kilns and were Inomata et al. 2020) in challenging environments. further evaluated in different archaeological contexts Most archaeological mapping programs based on and configurations (Caspari and Crespo 2019; Gallwey et ALS do not use LiDAR 3D point clouds directly, but use al. 2019; Kazimi et al. 2018; Trier, Cowley and Waldeland instead derived elevation models that represent bare soil 2018; Verschoof-van der Vaart et al. 2020; Verschoof-van in the topographic landscape. Perception of the terrain is der Vaart and Lambers 2019). These studies focused on usually enhanced by specific visualization techniques (VT) image classification (predicting a label/class associated (Bennett et al. 2012; Devereux, Amable and Crow 2008; with an image) (Figure 1a) or object detection (predicting Doneus 2013; Hesse 2010; Štular et al. 2012) that are the location (i.e. bounding box (BBOX)) of one or several used to visually interpret landforms and archaeological objects of interest within the image) (Figure 1b). While structures (Kokalj and Hesse 2017). These visualizations these deep CNN methods have detected archaeological have resulted in better understanding of the human past structures adequately, they could not provide information in different periods and different regions of the world. that (semi-)automatically characterized them because For example, LiDAR-derived terrain combined with VT structures must be delineated to move from detection has been used to provide new insights into a prehistoric to characterization. Recent deep CNN methods, such as hillfort under a woodland canopy in England (Devereux Mask R-CNN (He et al. 2017), have object-segmentation et al. 2005), discover a pre-colonial capital in South Africa abilities (Figure 1c) that delineate objects. These deep (Sadr 2019), supplement large-scale analysis of a human- CNN methods remain strongly restricted by the large modified landscape in a Mayan archaeological site in number of samples required to train models and the Belize (Chase et al. 2011) and explore long-term human- need to define target classes before using the models. environment interactions within the former Khmer Empire While the lack of ground-truth samples (reference data) in Cambodia (Evans 2016). However, these expert-based is a known constraint in remote-sensing archaeological and time-consuming approaches are difficult to replicate prospection, two strategies can address this limitation: in large-scale archaeological prospection projects. transfer learning and data augmentation. The first A variety of (semi-)automatic feature-extraction strategy applies a pre-trained source domain model to methods have been developed to assist or supplement initialize a targeted domain model (Weiss, Khoshgoftaar these visual interpretation approaches. Object-based and Wang 2016), while the second strategy uses image analysis (Freeland et al. 2016) and template- transformers that modify input data for training. These matching (Trier and Pilø 2012) methods, which rely strategies are known to improve model performance on prior definition of purpose-built spatial descriptors for small datasets and to increase model generalization or prototypical patterns, respectively, are difficult (Shorten and Khoshgoftaar 2019). Defining target classes to generalize because they cannot include the high before using a model is based on one-class approaches morphological diversity and heterogeneous backgrounds that define only a generic “archeological structure” class of archaeological structures (Opitz and Herrmann 2018). without dividing it into several sub-classes, assuming Supervised machine-learning methods have been that the object characterization can identify types of assessed to address these limitations (Lambers, Verschoof- archaeological structures. van der Vaart and Bourgeois 2019). Data-driven classifiers Using deep CNN for archaeological prospection (e.g. random-forest, support vector machine) applied to of LiDAR derived-terrain (Caspari and Crespo 2019; multi-scale topographic or morphometric variables have Gallwey et al. 2019; Küçükdemirci and Sarris 2020; provided interesting results for detecting archeological Soroush et al. 2020; Trier, Cowley and Waldeland 2018; structures (Guyot, Hubert-Moy and Lorho 2018; Niculit,ă Verschoof-van der Vaart et al. 2020; Verschoof-van 2020). However, detection was either performed at the der Vaart and Lambers 2019) is in its infancy, and to pixel level without considering the target as an entire our knowledge, these studies have not evaluated the object (archaeological structure) with spatial aggregation object-segmentation abilities of the CNN, except the and internal complexities, or was based on previous image evaluation of Mask R-CNN for simple circular-based Guyot et al. Journal of Computer Applications in Archaeology DOI: 10.5334/jcaa.64 3 Figure 1 Image analysis using deep learning Convolutional Neural Networks for an archaeological site (Tumulus du Moustoir, Carnac, France). (a) Image classification: a class or label associated with the image, (b) Object detection: a labeled bounding box that locates the object of interest within the image, and (c) Object segmentation: a labeled footprint that locates and delineates the object of interest within the image. Figure 2 The study area with the location of the 195 annotated archaeological sites used in the study. Areas mentioned in the text are labeled. landforms (Kazimi, Thiemann & Sester 2019; Kazimi, data, since data are a sparse resource in archaeology, and Thiemann & Sester 2020). In the present study, we ii) after object detection, the utility of object segmentation assess the contribution of deep CNN to the combined for characterizing archaeological structures. detection and segmentation of archeological structures for further (semi-)automatic characterization. More specifically, we aim to provide new insights into 2. MATERIALS AND METHODS object segmentation using deep CNN for archaeological 2.1. STUDY AREA prospection to address two key issues: i) the extent to The study area (Figure 2) is located in southern Morbihan which the approach is sensitive to the amount of sample (Brittany, France)
Details
-
File Typepdf
-
Upload Time-
-
Content LanguagesEnglish
-
Upload UserAnonymous/Not logged-in
-
File Pages19 Page
-
File Size-