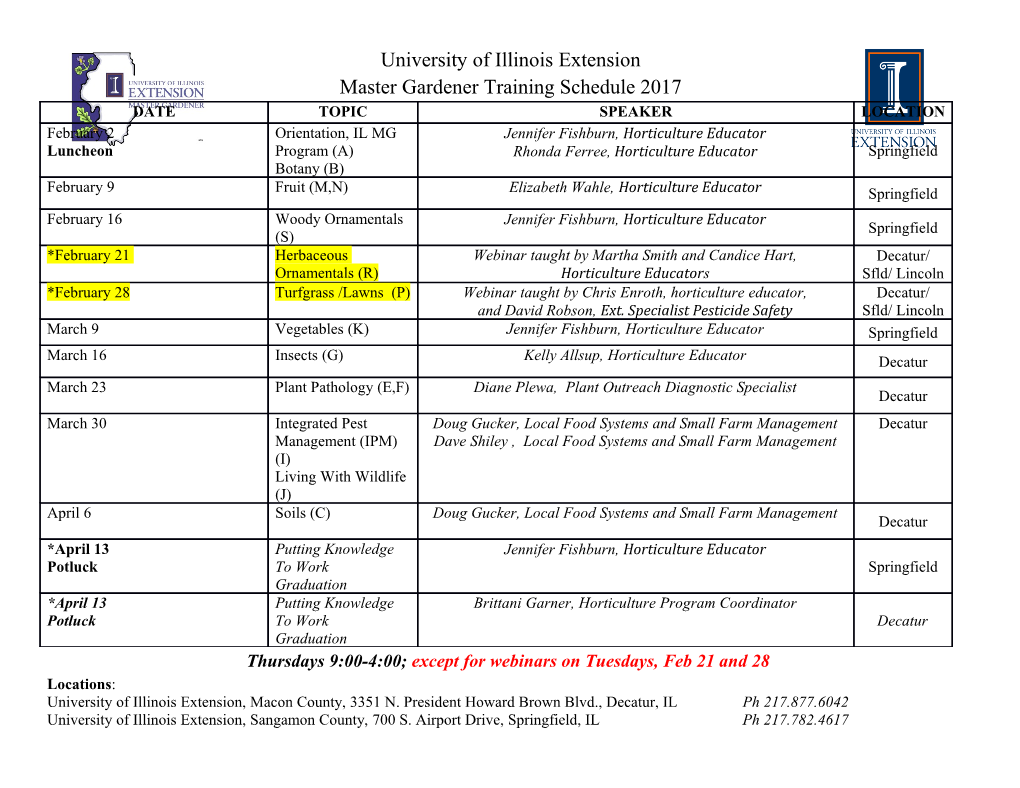
bioRxiv preprint doi: https://doi.org/10.1101/2020.07.15.176933; this version posted July 15, 2020. The copyright holder for this preprint (which was not certified by peer review) is the author/funder, who has granted bioRxiv a license to display the preprint in perpetuity. It is made available under aCC-BY-NC-ND 4.0 International license. Alignment-free machine learning approaches for the lethality prediction of potential novel human-adapted coronavirus using genomic nucleotide Rui Yin1,*, Zihan Luo2, Chee Keong Kwoh1 1 Biomedical Informatics Lab, Nanyang Technological University, Singapore, Singapore 2 School of Electronic Information and Communications, Huazhong University of Science and Technology, Wuhan, Hubei Province, China * yinr0002@e.ntu.edu.sg Abstract A newly emerging novel coronavirus appeared and rapidly spread worldwide and World 1 Health Organization declared a pandemic on March 11, 2020. The roles and 2 characteristics of coronavirus have captured much attention due to its power of causing 3 a wide variety of infectious diseases, from mild to severe on humans. The detection of 4 the lethality of human coronavirus is key to estimate the viral toxicity and provide 5 perspective for treatment. We developed alignment-free machine learning approaches for 6 an ultra-fast and highly accurate prediction of the lethality of potential human-adapted 7 coronavirus using genomic nucleotide. We performed extensive experiments through six 8 different feature transformation and machine learning algorithms in combination with 9 digital signal processing to infer the lethality of possible future novel coronaviruses 10 using previous existing strains. The results tested on SARS-CoV, MERS-Cov and 11 SARS-CoV-2 datasets show an average 96.7% prediction accuracy. We also provide 12 preliminary analysis validating the effectiveness of our models through other human 13 coronaviruses. Our study achieves high levels of prediction performance based on raw 14 RNA sequences alone without genome annotations and specialized biological knowledge. 15 The results demonstrate that, for any novel human coronavirus strains, this 16 alignment-free machine learning-based approach can offer a reliable real-time estimation 17 for its viral lethality. 18 Introduction 19 Coronaviruses are positive, single-stranded RNA virus and have been identified in 20 humans and animals. They are categorized into four genera: α, β, γ and θ [1]. Previous 21 phylogenetic analysis revealed a complex evolutionary history of coronavirus, suggesting 22 ancient origins and crossover events that can lead to cross-species infections [2] [3]. Bats 23 and birds are a natural reservoir for coronavirus gene pool [4]. The mutation and 24 recombination play critical roles that enable cross-species transmission into other 25 mammals and humans [5]. Human coronavirus (HCoV) was first identified in the 26 mid-1960s [6], and up to now, seven types of coronavirus can infect people. Four of 27 them, i.e., HCoV-229E, HCoV-NL63, HCoV-OC43 and HCoV-HKU1, usually cause 28 mild to moderate upper-respiratory tract illness like common cold when infecting 29 1/18 bioRxiv preprint doi: https://doi.org/10.1101/2020.07.15.176933; this version posted July 15, 2020. The copyright holder for this preprint (which was not certified by peer review) is the author/funder, who has granted bioRxiv a license to display the preprint in perpetuity. It is made available under aCC-BY-NC-ND 4.0 International license. humans [7]. The other three members include severe acute respiratory syndrome 30 coronavirus (SARS-CoV) and middle east respiratory syndrome coronavirus 31 (MERS-CoV) and the most lately severe acute respiratory syndrome coronavirus 2 32 (SARS-CoV-2), also known as COVID-19 coronavirus. They all belong to 33 Betacoronavirus that led to the epidemics or pandemics [8] [9] [10]. 34 Emerging in November 2002 in Guangdong province, China, SARS caused 8,096 35 human infections with 774 deaths by July 2003 [11]. MERS was first reported in Saudi 36 Arabia in September 2012 and finally resulted in 2,494 human infections by November 37 2019 [12]. Recently, a novel coronavirus named COVID-19 is emerging and spreading to 38 215 countries or territories on June 12, 2020, leading to 7,390,702 confirmed cases with 39 417,731 deaths according to the World Health Organization [13]. Though precautions 40 such as lockdown of cities and social distance have been taken to curb the transmission 41 of COVID-19, it spreads far more quickly than the SARS-CoV and MERS-CoV 42 diseases [4] [14]. To make matters worse, the number of infected cases still increases 43 rapidly and the global inflection point about COVID-19 is unknown. Like other RNA 44 viruses, e.g. influenza virus [15], coronaviruses possess high mutation and gene 45 recombination rates [16], which makes constant evolution of this virus with the 46 emergence of new variants. From SARS in 2002 to COVID-19 in 2019, coronaviruses 47 have caused high morbidity and mortality, and unfortunately, the fast and untraceable 48 virus mutations take the lives of people before the immune system can produce the 49 inhibitory antibody [17]. Currently, no miracle drug or vaccines are available to treat or 50 prevent the humans infected by coronaviruses [18] [19]. Therefore, there is a desperate 51 need for developing approaches to detect the lethality of coronaviruses not only for 52 COVID-19 but also the potential new variants and species. This would facilitate the 53 diagnosis of coronavirus clinical severity and provide decision-making support. 54 The detection of viral lethality has already been explored in influenza viruses [20]. 55 Through a meta-analysis of predicting the virulence and antigenicity of influenza 56 viruses, we can infer the lethality of the virus timely to improve the current influenza 57 surveillance system [21]. Regarding the risk of novel emerging coronavirus strains, much 58 attention has been captured to investigate the lethality or clinical severity of new 59 emerging coronavirus. Typically, epidemiological models are certainly built to estimate 60 the lethality and the extent of undetected infections associated with the new 61 coronaviruses. Bastolla suggested an orthogonal approach based on a minimum number 62 of parameters robustly fitted from the cumulative data easily accessible for all countries 63 at the John Hopkins University database to extrapolate the death rate [22]. 64 Bello-Chavolla et al. proposed a clinical score to evaluate the risk for complications and 65 lethality attributable to COVID-19 regarding the effect of obesity and diabetes in 66 Mexico [23]. The results provided a tool for quick determination of susceptibility 67 patients in a first contact scenario. Want et al. leveraged patient data in real-time and 68 devise a patient information based algorithm to estimate and predict the death rate 69 caused by COVID-19 for the near future [24]. Aiewsakun et al. performed a 70 genome-wide association study on the genomes of COVID-19 to identify genetic 71 variations that might be associated with the COVID-19 severity [25]. Moreover, Jiang et 72 al. established an artificial intelligence framework for data-driven prediction of 73 coronavirus clinical severity [26]. The development of computational and physics-based 74 approaches has relieved the labors of experiments by utilizing epidemiological and 75 biological data to construct the model. However, direct evaluation of potential novel 76 coronavirus strains for their lethality is crucial when clinicians are forced to make 77 difficult decisions without past specific experience to guide clinical acumen. Inferring 78 the lethality of novel coronavirus is possible by identifying the patterns from a large 79 number of coronavirus sequences. 80 In this paper, we propose alignment-free machine learning-based approaches to infer 81 2/18 bioRxiv preprint doi: https://doi.org/10.1101/2020.07.15.176933; this version posted July 15, 2020. The copyright holder for this preprint (which was not certified by peer review) is the author/funder, who has granted bioRxiv a license to display the preprint in perpetuity. It is made available under aCC-BY-NC-ND 4.0 International license. the lethality of potential novel human-adapted coronavirus using genomic sequences. 82 The main contribution is that we formulate the problem of estimating the lethality of 83 human-adapted coronavirus through machine learning approaches. By leveraging some 84 appropriate feature transformation, we can encode genomic nucleotides into numbers 85 that allow us to convert it into a prediction task. The experimental results suggest our 86 models deliver accurate prediction of lethality without prior biological knowledge. We 87 also performed phylogenetic analysis validating the effectiveness of our models through 88 other human coronaviruses. 89 Materials and Methods 90 Problem formulation 91 The pandemic of novel coronavirus COVID-19 has caused thousands of fatalities, 92 making tremendous treats to public health worldwide. The society is deeply concerned 93 about its spread and evolution with the emergence of any potential new variants, that 94 would increase the lethality. Typically, lethality refers to the capability of causing death. 95 It is usually estimated as the cumulative number of deaths divided by the total number 96 of confirmed cases. Among all the human-adapted coronaviruses, MERS-CoV caused 97 the highest fatality rate of 37% [12], followed by the SARS-CoV with 9.3% fatality 98 rate [27]. In comparison, COVID-19 indicates a lower mortality rate of 5.5% [13]. The 99 lethality rate of COVID-19 is likely to decrease with better treatment and precautions. 100 In this paper, we mainly focus on these three types of human-adapted coronavirus and 101 define the degree of viral lethality in terms of historical fatality rates. As a result, 102 MERS-CoV strains are high lethal while SARS-CoV and COVID-19 strains are middle 103 and low lethal, respectively.
Details
-
File Typepdf
-
Upload Time-
-
Content LanguagesEnglish
-
Upload UserAnonymous/Not logged-in
-
File Pages18 Page
-
File Size-