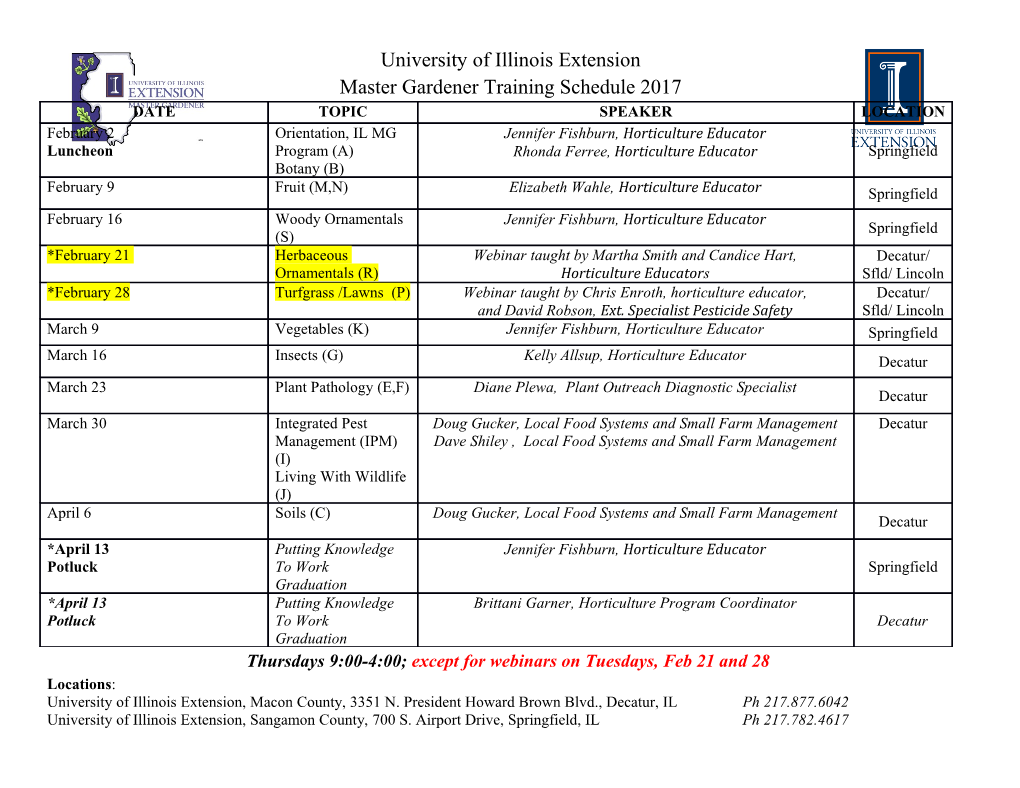
Good and bad properties of the Kelly criterion∗ Leonard C. MacLean, Herbert Lamb Chair (Emeritus),School of Business, Dalhousie University, Halifax, NS Edward O. Thorp, E.O. Thorp and Associates, Newport Beach, CA Professor Emeritus, University of California, Irvine William T. Ziemba, Professor Emeritus, University of British Columbia, Vancouver, BC Visiting Professor, Mathematical Institute, Oxford University, UK ICMA Centre, University of Reading, UK University of Bergamo, Italy January 1, 2010 Abstract We summarize what we regard as the good and bad properties of the Kelly criterion and its variants. Additional properties are discussed as observations. The main advantage of the Kelly criterion, which maximizes the expected value of the logarithm of wealth period by period, is that it maximizes the limiting exponential growth rate of wealth. The main disadvantage of the Kelly criterion is that its suggested wagers may be very large. Hence, the Kelly criterion can be very risky in the short term. In the one asset two valued payoff case, the optimal Kelly wager is the edge (expected return) divided by the odds. Chopra and Ziemba (1993), reprinted in Section 2 of this volume, following earlier studies by Kallberg and Ziemba (1981, 1984) showed for any asset allocation problem that the mean is much more important than the variances and co-variances. Errors in means versus errors in variances were about 20:2:1 in importance as measured by the cash equivalent value of final wealth. Table 1 and Figure 1 show this and illustrate that the relative importance depends on the degree of risk aversion. The ∗Special thanks go to Tom Cover and John Mulvey for helpful comments on an earlier draft of this paper. 1 Good and bad properties MacLean, Thorp, Ziemba lower is the Arrow-Pratt risk aversion, RA = −u00(w)=u0(w), the higher are the relative errors from incorrect means. Chopra (1993) further shows that portfolio turnover is larger for errors in means than for variances and for co-variances but the degree of difference in the size of the errors is much less than the performance as shown in Figure 2. Table 1: Average Ratio of Certainty Equivalent Loss for Errors in Means, Variances and Covariances. Source: Chopra and Ziemba (1993) Errors in Means Errors in Means Errors in Variances Risk Tolerance* vs Covariances vs Variances vs Covariances 25 5.38 3.22 1.67 50 22.50 10.98 2.05 75 56.84 21.42 2.68 ### 20 10 2 Error Mean Error Var Error Covar 20 2 1 ∗ 100 u00(w) Risk tolerance=RT (w) = 1 where RA(w) = − Price (U.S. dollars) RA(w) u0(w) 2 15 900.00 14 PNP 13 800.00 % Cash S&P 500 Equivalent Loss 12 U.S. T-Bill 11 11 700.00 Means Nav Index 10 10 600.00 9 9 8 8 500.00 7 7 Index 400.00 6 6 5 5 300.00 4 4 3 200.00 3 S&P500 2 2 100.00 1 Variances Covariances 1 0 0 0.00 0 0.05 0.10 0.15 0.20 11/68 10/73 11/77 10/81 10/85 12/88 01 -02 -02 -02 02 -03 04 -04 04 -05 05 06 Magnitude of error (k) ar ar -03 -03 ar -04 -04 ar -05 -05 Dec- M Jun Sep Dec- M Jun Sep Dec- M Jun Sep Dec- M Jun Sep Dec- Dec- Date Figure 1: Mean Percentage Cash Equivalent Loss Due to Errors in Inputs. Since log has RA(w) = 1=w, which is close to zero, The Kelly bets may be exceedingly large and risky for favorable bets. In MacLean, Thorp, Zhao and Ziemba (2009) in this section of this volume, we present simulations of medium term Kelly, fractional Kelly and propor- tional betting strategies. The results show that with favorable investment opportunities, Kelly bettors attain large final wealth most of the time. But, because a long sequence of bad scenario outcomes is possible, any strategy can lose substantially even if there are many independent investment opportunities and the chance of losing at each investment 2 The Stochastic Programming Approach to Asset, Liability, and Wealth Management Figure 1.7. Mean Percentage Cash-Equivalent Loss because of Errors in Inputs Cash-Equivalent Loss (%) 11 10 9 8 7 6 5 4 3 2 1 0 0 0.05 0.10 0.15 0.20 Magnitude of Error (k) Means Variances Covariances Source: Based on data from Chopra and Ziemba (1993). Good and bad properties MacLean, Thorp, Ziemba Figure 1.8. Average Turnover for Different Percentage Changes in Means, Variances, and Covariances Average Turnover (% per month) 50 40 Means 30 Variances 20 10 Covariances 10 5 10 15 20 25 30 35 40 45 50 Change (%) Source: Based on data from Chopra (1993). Figure 2: Average turnover for different percentage changes in means, variances and co- variances. Source: Based on data from Chopra (1993) 12 ©2003, The Research Foundation of AIMR™ decision point is small. The Kelly and fractional Kelly rules, like all other rules, are never a sure way of winning for a finite sequence. In Section 6 of this volume, we describe the use of the Kelly criterion in many applications and by many great investors. Two of them, Keynes and Buffett, were long term investors whose wealth paths were quite rocky but with good long term outcomes. Our analyses suggest that Buffett seems to act similar to a fully Kelly bettor (subject to the constraint of no borrowing) and Keynes like a 80% Kelly bettor with a negative power utility function −w−0:25, see Ziemba (2003). See the wealth graphs reprinted in section 6 from Ziemba (2005). Graphs such as Figure 3 show that growth is traded off for security with the use of fractional Kelly strategies and negative power utility functions. Log maximizes the long run growth rate. Utility functions such as positive power that bet more than Kelly have more risk and lower growth. One of the properties shown below that is illustrated in the graph is that for processes which are well approximated by continuous time, the growth rate becomes zero plus the risk free rate when one bets exactly twice the Kelly wager. Hence it never pays to bet more than the Kelly strategy because then risk increases (lower security) and growth decreases, so Kelly dominates all these strategies in geometric risk- return or mean-variance space. See Ziemba (2009) in this volume. As you exceed the Kelly bets more and more, risk increases and long term growth falls, eventually becoming more and more negative. Long Term Capital is one of many real 3 6 Good and bad properties MacLean, Thorp, Ziemba Figure 2: Probability of doubling and quadrupling before halving and relative growth rates versus fraction of wealth wagered for Blackjack (2% advantage, p=0.51 and q=0.49). Source: McLean and Ziemba (1999) Figure 3: Probability of doubling and quadrupling before halving and relative growth rates versus fraction of wealth wagered for Blackjack (2% advantage, p=0.51 and q=0.49). Source: MacLean and ZiembaTable (1999) 3: Growth Rates Versus Probability of Doubling Before Halving for Blackjack Kelly Fraction P[Doubling before Relative of Current Halving] Growth Rate world instances in which overbetting led to disaster.Wealth See Ziemba and Ziemba (2007) for additional examples. ì 0.1 0.999 0.19 | 0.2 0.998 0.36 Thus long term growth maximizingRange investors| should0.3 bet_ Kelly or less.0.98 We call betting_ 0.51 for | 0.4 Safer 0.94 Less Growth 0.64 less than Kelly \fractional Kelly,"Blackjack whichí is simply0.5 a blend of Kelly0.89 and cash. Consider 0.75 the negative power utility functionTeams δ!|δ for δ <0.60. ThisRiskier utility function0.83 is concaveMore Growth and 0.84 when δ ! 0 it converges to log utility.| As δ becomes0.7 more_ negative,0.78 the investor is_ less 0.91 î 0.8 0.74 0.96 aggressive since his absolute Arrow-Pratt risk aversion0.9 index is also0.70 higher. For the case 0.99 δ of a stationary lognormal process and a given δ for1.0 utilityKelly function δw0.67and α = 1=(1 − δ) 1.00 between 0 and 1, they both will provide the same1.5 optimal portfolio when0.56 α is invested in 0.75 Overkill à 2.0 0.50 0.00 the Kelly portfolio and 1 −Tooα is Risky invested in cash. This handy formula relatingSource: the MacLean coefficient and Ziemba of (1999) the negative power utility function to the Kelly fraction is correct for lognormal investments and approximately correct for other distributed assets; see MacLean,In the next Ziemba two columns, and Li I will (2005). discuss For three example, topics: good half andKelly bad properties is δ = of− the1 Kelly log strategy and quarter Kelly is δ = −and3. why So this if led you me want to work a with less Len aggressive MacLean on path a through than study Kelly of fractional pick an Kelly strategies and appropriate δ. This formulainvesting does notusing apply unpopular more numbers generally. in lotto games For example, with low probabilities for coin tossing,of success where the returns where P r(X = 1) = p; P r(areX very− 1) large = q; (this p +illustratesq = 1, how bets can be very tiny) next month; and futures and commodity 1 1 1−δ 1−δ α α ∗ p − q p − q fδ = 1 1 = α α p 1−δ + q 1−δ p + q ∗ ∗ which is not αf , where f = p − q > 0 is the Kelly bet.
Details
-
File Typepdf
-
Upload Time-
-
Content LanguagesEnglish
-
Upload UserAnonymous/Not logged-in
-
File Pages11 Page
-
File Size-