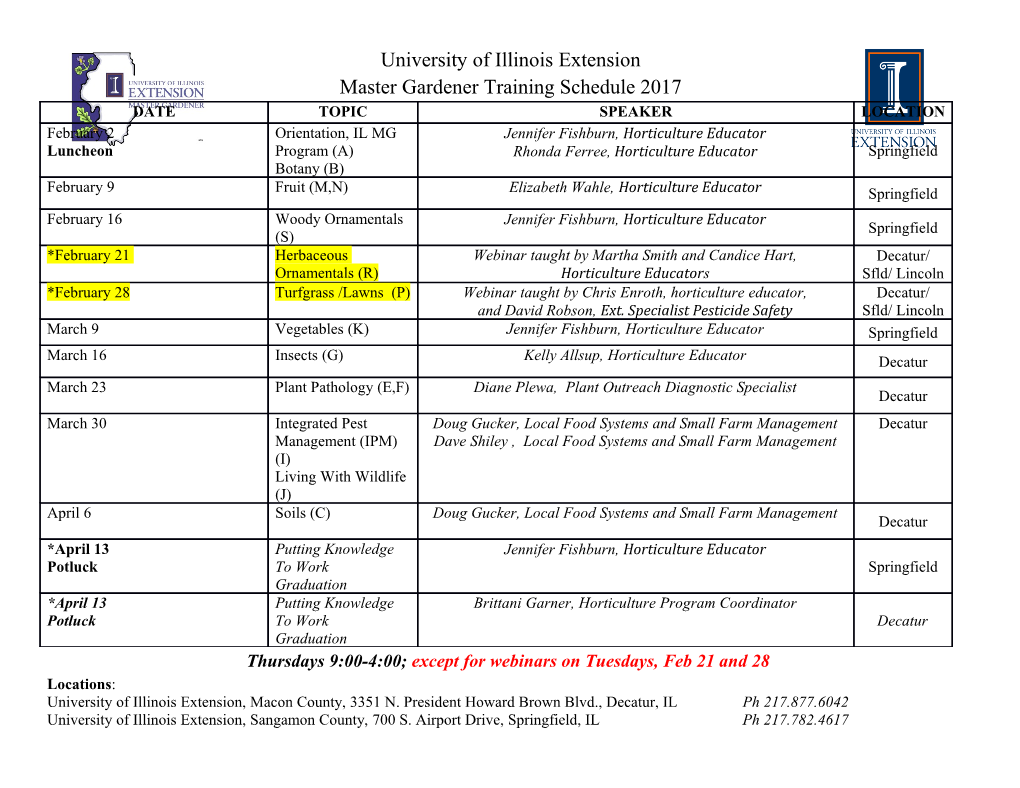
In silico virulence prediction and virulence gene discovery of Streptococcus agalactiae FrankPo-YenLIN Centre for Health Informatics School of Public Health and Community Medicine University of New South Wales A thesis submitted in fulfilment of requirements for the degree of Doctor of Philosophy October 2009 Declaration of originality I hereby declare that this submission is my own work and to the best of my knowl- edge it contains no materials previously published or written by another person, nor material which to a substantial extent has been accepted for the award of any other degree or diploma at UNSW or any other educational institution, except where due acknowledgement is made in this thesis. Any contribution made to the research by others, with whom I have worked at UNSW or elsewhere, is explicitly acknowledged in the thesis. I also declare that the intellectual content of the thesis is the product of my own work, except to the extent that assistance from others in the project’s design and conception or in style, presentation, and linguistic expression is acknowledged. Frank Po-Yen LIN October 2009 To my parents and my aunt Abstract Physicians frequently face challenges in predicting which bacterial subpopulations are likely to cause severe infections. A more accurate prediction of virulence would improve diagnostics and limit the extent of antibiotic resistance. Nowadays, bac- terial pathogens can be typed with high accuracy with advanced genotyping tech- nologies. However, effective translation of bacterial genotyping data into assess- ments of clinical risk remains largely unexplored. The discovery of unknown virulence genes is another key determinant of suc- cessful prediction of infectious disease outcomes. The trial-and-error method for virulence gene discovery is time-consuming and resource-intensive. Selecting can- didate genes with higher precision can thus reduce the number of futile trials. Sev- eral in silico candidate gene prioritisation (CGP) methods have been proposed to aid the search for genes responsible for inherited diseases in human. It remains uninvestigated as to how the CGP concept can assist with virulence gene discovery in bacterial pathogens. The main contribution of this thesis is to demonstrate the value of translational bioinformatics methods to address challenges in virulence prediction and viru- lence gene discovery. This thesis studied an important perinatal bacterial pathogen, group B streptococcus (GBS), the leading cause of neonatal sepsis and meningi- tis in developed countries. While several antibiotic prophylactic programs have successfully reduced the number of early-onset neonatal diseases (infections that occur within 7 days of life), the prevalence of late-onset infections (infections that occur between 7–30 days of life) remained constant. In addition, the widespread use of intrapartum prophylactic antibiotics may introduce undue risk of penicillin allergy and may trigger the development of antibiotic-resistant microorganisms. To minimising such potential harm, a more targeted approach of antibiotic use is required. Distinguish virulent GBS strains from colonising counterparts thus lays the cornerstone of achieving the goal of tailored therapy. There are three aims of this thesis: 1. Prediction of virulence by analysis of bacterial genotype data: To identify markers that may be associated with GBS virulence, statistical anal- ysis was performed on GBS genotype data consisting of 780 invasive and 132 colonising S. agalactiae isolates. From a panel of 18 molecular markers stud- ied, only alp3 gene (which encodes a surface protein antigen commonly associ- ated with serotype V) showed an increased association with invasive diseases (OR=2.93, p=0.0003, Fisher’s exact test). Molecular serotype II (OR=10.0, p=0.0007) was found to have a significant association with early-onset neonatal disease when compared with late-onset diseases. To investigate whether clinical outcomes can be predicted by the panel of geno- type markers, logistic regression and machine learning algorithms were applied to distinguish invasive isolates from colonising isolates. Nevertheless, the pre- dictive analysis only yielded weak predictive power (area under ROC curve, AUC: 0.56–0.71, stratified 10-fold cross-validation). It was concluded that a definitive predictive relationship between the molecular markers and clinical outcomes may be lacking, and more discriminative markers of GBS virulence are needed to be investigated. 2. Development of two computational CGP methods to assist with functional dis- covery of prokaryotic genes: Two in silico CGP methods were developed based on comparative genomics: statistical CGP exploits the differences in gene frequency against phenotypic ii groups, while inductive CGP applies supervised machine learning to identify genes with similar occurrence patterns across a range of bacterial genomes. Three rediscovery experiments were carried out to evaluate the CGP methods: • Rediscovery of peptidoglycan genes was attempted with 417 published bacterial genome sequences. Both CGP methods achieved their best AUC >0.911 in Escherichia coli K-12 and >0.978 Streptococcus agalactiae 2603 (SA-2603) genomes, with an average improvement in precision of >3.2-fold and a maximum of >27-fold using statistical CGP. A median AUC of >0.95 could still be achieved with as few as 10 genome examples in each group in the rediscovery of the peptidoglycan metabolism genes. • A maximum of 109-fold improvement in precision was achieved in the rediscovery of anaerobic fermentation genes. • In the rediscovery experiment with genes of 31 metabolic pathways in SA- 2603, 14 pathways achieved an AUC >0.9 and 28 pathways achieved AUC >0.8 with the best inductive CGP algorithms. The results from the re- discovery experiments demonstrated that the two CGP methods can assist with the study of functionally uncategorised genomic regions and the dis- covery of bacterial gene-function relationships. 3. Application of the CGP methods to discover GBS virulence genes: Both statistical and inductive CGP were applied to assist with the discovery of unknown GBS virulence factors. Among a list of hypothetical protein genes, several highly-ranked genes were plausibly involved in molecular mechanisms in GBS pathogenesis, including several genes encoding family 8 glycosyltrans- ferase, family 1 and family 2 glycosyltransferase, multiple adhesins, strepto- coccal neuraminidase, staphylokinase, and other factors that may have roles in contributing to GBS virulence. Such genes may be candidates for further bio- iii logical validation. In addition, the co-occurrence of these genes with currently known virulence factors suggested that the virulence mechanisms of GBS in causing perinatal diseases are multifactorial. The procedure demonstrated in this prioritisation task should assist with the discovery of virulence genes in other pathogenic bacteria. iv Acknowledgements First of all, I wish to express my gratitude to my supervisor Professor Enrico Coiera for his guidance and encouragements over the last three years. In particular, I could not have finished my work without his constant optimism, experience, and strive for perfection. My gratitude goes equally to Dr Vitali Sintchenko, my co-supervisor, whose vision and the remarkable attention to details have truly inspired me. Both Enrico and Vitali have strengthened my interest in the fields of clinical decision support and genomics. Their encouragements have been an essential element to my candidature. Coming from a non-technical, non-laboratory background, I could not have completed this thesis without the expertise and assistance of the following people: Professor Lyn Gilbert and Dr Fanrong Kong for leading me into the fascinating fields of clinical microbiology and molecular epidemiology; Heather Hiddings for her helpful discussions in biostatistics; Danny Ko for collecting and curating GBS genotyping data; Dr. Ruiting Lan for his expert knowledge on microbial genet- ics; and Drs. Mike Bain, Ashwin Srinivasan and Guy Tsafnat for sharing their knowledge on machine learning and their generous comments in assisting me with experimental design. I am also greatly indebted to Enrico, Vitali, Lyn, Kong, and Ruiting for their assistance for editing the earlier drafts of this thesis. I would also like to thank many anonymous reviewers and editors of BMC Bioinformatics, Journal of Infectious Diseases, Clinical Microbiology and Infec- i tion,andPathology with their invaluable insights on my work. Their constructive criticisms constituted a substantial part of my learning in conducting rigorous sci- entific research (albeit sometimes the hard way!). Over the years, I received numerous useful advice and helpful discussions from my colleagues and seniors at the Centre for Health Informatics, including but not least (in alphabetical order) Stephen Anthony, Farshid Anvari, Grace Chung, Adam Dunn, Blanca Gallego Luxan, Andrew Georgiou, Simon Li, Annie Lau, Farah Ma- grabi, Geoff McDonnell, Hieu Phan, Victor Vickland, Prof. Johanna Westbrook, Tatjana Zrimec, and from my fellow students past and present: Afra, David, Jaron, MeiSing, Nerida, Rosie, Torsten, and Zafar. Also, I could not have done without the dedicated admin team for their assistance: Sarah Behman, Keri Bell, Danielle Del Pizzo, Janice Ooi, Samantha Sheridan, Denise Tsiros, and Gerard Viswasam. Financially, I would like to thank National Health and Medical Research Coun- cil of Australia for funding my scholarship. I wish to thank
Details
-
File Typepdf
-
Upload Time-
-
Content LanguagesEnglish
-
Upload UserAnonymous/Not logged-in
-
File Pages320 Page
-
File Size-