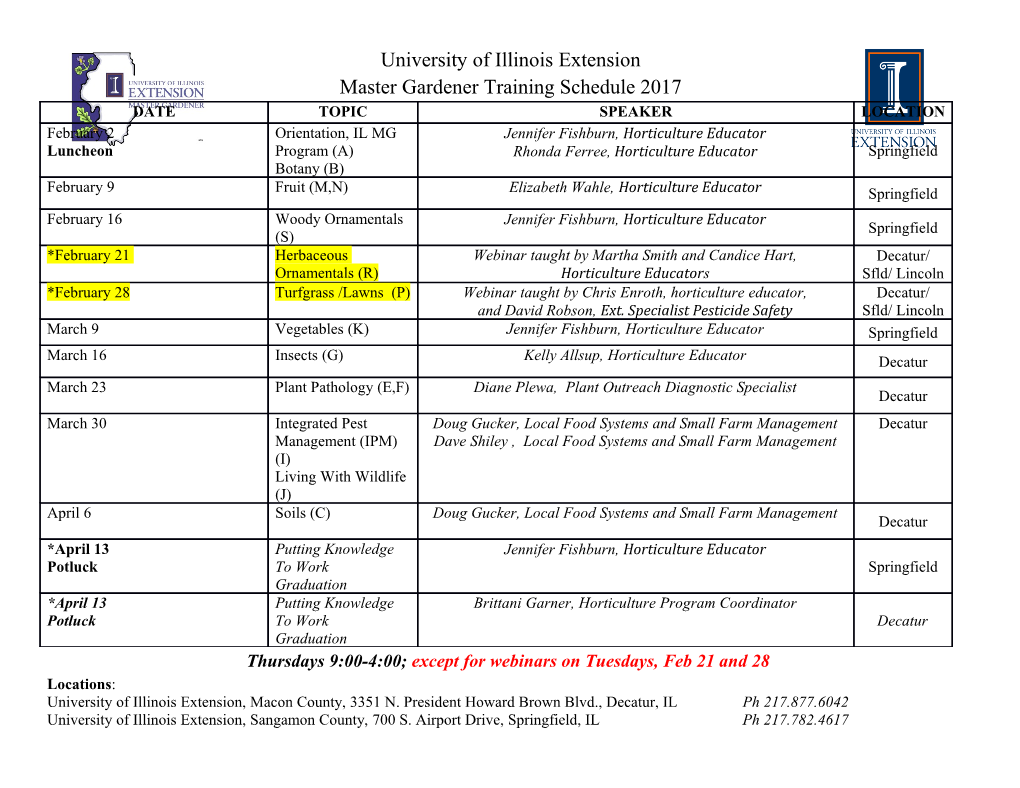
Chapter 9 Analysis of Amphibian Biodiversity Data Lee-Ann C. Hayek Introduction tion of a situation and its definition in opera- tional terms can lead to a correct choice for data In this chapter I discuss problems of use, misuse, analysis. When in doubt about correct statistical and problematical interpretation of data, spe- procedures, or when irregularities arise, investi- cifically for amphibian biologists. I focus on gators should always seek the advice of a mathe- statistical procedures that are appropriate for matical statistician with experience m biological amphibian sampling and monitoring and con- applications. Theory is always well behaved; sider limiting factors and assumptions of statisti- data never are. cal models in terms suitable for observational and other studies. The number of formulas I present is negligible, and they involve only sim- Species richness ple algebraic statements. This chapter is not all- encompassing. Presence-Absence Data No technique is universally appropriate, and standardization, as used here, does not imply Amphibian data may be recorded on a nominal, that only a single method of analysis exists. In- ordinal, interval, or ratio scale (see "Measure- deed, in some cases a good amount of work will ment Scales and Statistical Analysis," Chap- have to be done before any choice can be made. ter 4). Data measured against the first two scale Only a mathematical and probabilistic evalua- types are called nonmetric; those measured 207 208 CHAPTER 9 against the latter two are called interval, metric, Table 19. Generalized 2x2 Frequency Table or continuous. Single inventories can lead di- Using Counts rectly to nonmetric data by providing a total State, State, habitat, or species count with a designation of species as habitat, species A abundant, common, or rare; a list of species by or name; or a record of presence or absence of each species B Present Absent Total species within a selected habitat or sample. In some instances, the results of a monitoring effort Present a b a + b also may be presented as nonmetric data because Absent C d c + d of the nontrivial reduction in effort relative to a Total a + c b + d n study design calling for interval data. Results can be obtained quickly or inexpensively with a preliminary analysis of the research effort, and a given area. Fourfold (or 2 y. 2) contingency problems with sampling techniques can be tables are especially convenient for summariz- detected. ing data regarding the relationship between two Nonmetric data also may be the only possible species, populations, habitats, or localities (e.g., or reasonable choice when the precision with Table 19), because the number of categories which the data will be collected is in question• (four) is less than the sample size (number of for example, if collector reliability is low. In observations). Pairwise comparisons for all addition, with some sampling methods (e.g., combinations of species over an entire data set audio strip transects and night driving, discussed are a realistic means by which to summarize a in Chapters 6 and 7, respectively), measurement large set of observations and to examine inter- or observation error can be high, and the record- specific relationships. When associations among mg of precise continuous variables may be prob- species are not obvious and a priori assumptions lematic, unnecessary, or even deleterious to the are neither desirable nor possible, methods such study. Alternatively, for certain studies a contin- as Ä-mode and g-mode cluster analyses (e.g., uous variable may be categorized after it has Gower 1967; Hazel 1970; Anderberg 1973; been recorded, as when age data for juveniles or Sneath and Sokal 1973) can be used to identify adults are grouped. relationships. Presence-absence data can be coded using the The amount of information in data sets that numbers i and 0 for computer input. Whether a merely record presence and absence is consid- species is rare or abundant, its simple presence is ered to be minimal, and information is presumed indicated by a 7. Such data are termed two-state, to be lost when a continuous variable has its binary, categorical, or nonmetric, as well as scale categorized. Presumably, the smaller the nominal, number of categories, the greater the loss of A list of presence-absence data for species in information. Several authors have addressed this a particular area provides information on geo- question (Bonham-Carter 1965; Buzas 1967, graphic or habitat distribution of those species, 1972a; Erez and Gill 1977). Gill and Tipper but little else. These data can, however, provide (1978) tested the hypothesis that the results ob- information on species interactions that may be tained firom a study with metric variables can be particularly useful in explaining observed distri- obtained from binary nonmetric data. They di- butions. Such interactions are generally exam- chotomized and reanalyzed the data from six ined by means of pairwise comparisons of large multi varia te studies and showed agreement presence-absence among species or localities in between the pairs of analyses that ranged from Analysis (^ Biodiversity Data 209 75% to 97%. They concluded that for those 1. Similarity-dissimilarity coefficients. These studies, nonmetric binary data were entirely coefficients reflect the proportion of the adequate. sample that represents mutual occurrence (Hohn 1976). Formulas for their calculation do not include specific consideration of Measures of Association joint absences of variables (species). Measures, or coefficients, of association have 2. Matching coefficients. Formulas for these indi- been in existence since at least the late 1800s. ces include joint absences or non-occurrences They are appropriate for use with binary data and do not discriminate between positive and and thus are especially important for fau nal negative association (Hohn 1976). comparisons because the data from such stud- 3. Traditional association coefficients. Usu- ies are often in binary form. Ancillary data on ally, these coefficients are based upon the presence or absence of taxonomic characters, chi-square statistic (Table 20: section C) or environmental states, observer concordance, a statistic computationally related to chi- and chemical properties also can be recorded square, or they have an underlying assump- in binary form and analyzed with measures of tion of normality. These coefficients are association. calculated with formulas that include Joint Measures of association have several impor- absences, and they can be evaluated against tant applications in amphibian studies: expres- a probability distribution. sion of faunal resemblance as similarity or dissimilarity among species observed at two or Coefficients in the first two categories treat more sample sites; assessment of the degree of a sample as if it were devoid of sampling or coexistence in different localities or habitats of measurement error. They can be based loosely particular species of amphibians, based on their or strictly upon a probabilistic model. They frequency of co-occurrence in a series of sam- are not test statistics, however, and have no ples or observations; and measurement of the related probability or sampling distributions. similarity or dissimilarity of habitat or micro- Alternatively, the traditional coefficients as- habitat choices or conditions for selected spe- sess the probability that an observed number cies. Additionally, these measures are useful for of co-occtirrences between two species has condensing data on the resemblance of species, arisen by chance. habitats, or samples and for summarizing large Some argue that before a measure of associa- amounts of species data, tion is calculated, a significance test should be applied. The test would be used to indicate the CLASSES OF COEFFICIENTS existence (at a stated probability level) of an Resemblance, similarity, matching, and asso- underlying relationship between the species, ciation are the adjectives most commonly ap- habitats, or localities being considered. It would plied to the class of coefficients used to describe how likely the observed result is under describe two-state presence-absence data. Dis- the null hypothesis of no association and would tinct disciplines have spawned a vast array of help to determine if an association were "real." such coefficients, many of which are redun- Both the test statistic and its significance level dant. The 46 most popular measures are pre- are influenced by sample size. For any given sented in Table 20. I have grouped them into altemative hypothesis, chi-square (or an equiva- three categories, that are not mutually ex- lent) increases and the significance level de- clusive but that facilitate consideration. creases as sample size increases. The binary 210 CHAPTER 9 Table 20. Measures of Association Relationship to # Coefficient Reference Alternative names Formula others A. Similarity coefTicients 1 Simpson Simpson Simpson coefficient a Equals Mountfotü's (1943) Degree of Faunal a + min(6, c) (#8) if population Resemblance follows a log distribution 2 Kulczynski Kulczynski Second Kulczynski \{ a 1 a'\ Can be written as the (1927) coefficient 2 a+b a+c arithmetic mean Driver and Kroeber \ J ofSinpson's(#l) coefficient andBraun- Blanquet's(#10) 3 Ochiai/ Ochiai Ochiai coefficieni a Same as the cosine Otsuka (1957) Otsuka coefficient coefficient for [(a+ *)(a+ £)]"" binary data Actually attributed to Otsuka in the Ochiai reference 4 Dice Dice (1945) Dice coefficient íSJ a Equivalent
Details
-
File Typepdf
-
Upload Time-
-
Content LanguagesEnglish
-
Upload UserAnonymous/Not logged-in
-
File Pages64 Page
-
File Size-