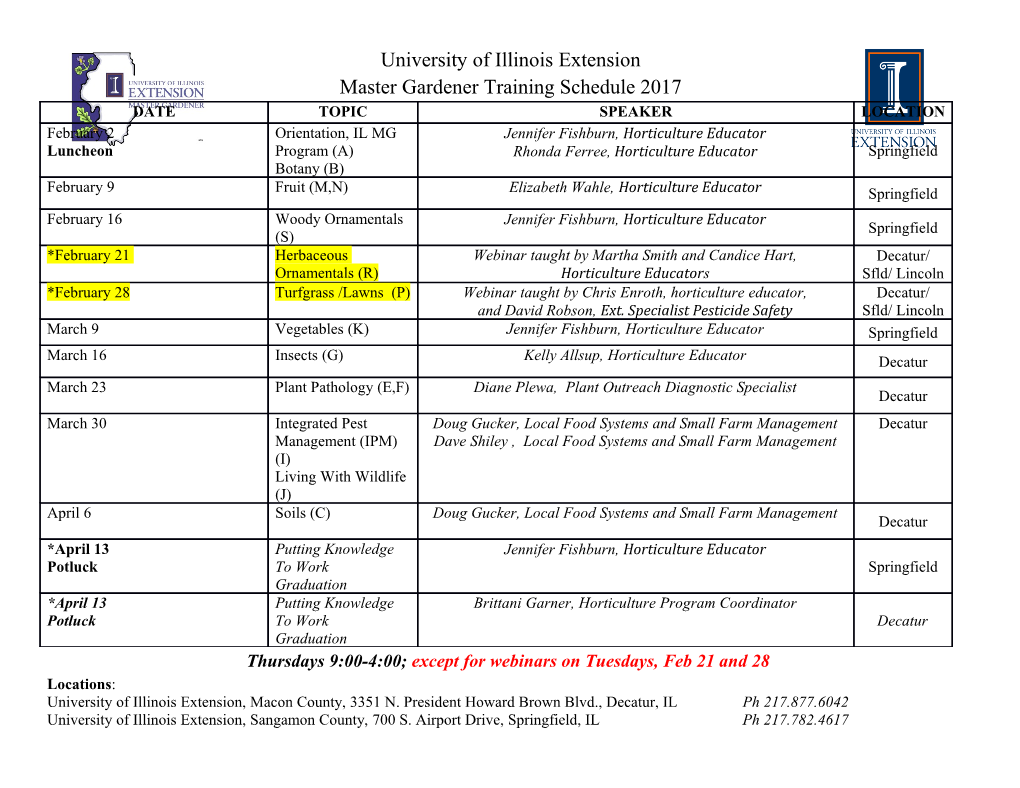
Analyzing Spatial Longitudinal Incidence Patterns Using Dynamic Multivariate Poisson Models Presented in Partial Fulfillment of the Requirements for the Degree Doctor of Philosophy in the Graduate School of The Ohio State University By Yihan Sui, B.S., M.S. Graduate Program in Biostatistics The Ohio State University 2018 Dissertation Committee: Dr. Grzegorz A. Rempala, Advisor Dr. Chi (Chuck) Song, Advisor Dr. Oksana Chkrebtii c Copyright by Yihan Sui 2018 Abstract Methods of multivariate analysis for continuous data have been applied extensively in epidemiology, economics, engineering, and other fields. However, multivariate models for count data have not been applied to a similar extent, and there is relatively little work published on models, especially when accounting for both spatial and temporal dependence. In the first part of the thesis, we propose a hierarchical multivariate Poisson (MVP) model that simultaneously models spatial and temporal correlation of observed data counts in a fairly general setting. In particular, MVP allows for modeling the spatially/temporally dependent counts as a function of location-specific and time-varying covariates. To characterize temporal trends, we propose a broken- line regression model within MVP and apply it to joinpoint detection. Bayesian inference is conducted using Markov chain Monte Carlo (MCMC) methods to ap- proximate required posterior summaries. We use a backward selection algorithm coupled with the shaver method to obtain joinpoint coefficients via Bayesian Lasso. We apply the proposed model to spatial temporal pertussis incidence data from 2000 to 2015 from several midwestern states in the U.S. To evaluate the appropriateness of the model, in the second part of this thesis we develop a goodness-of-fit (GOF) ii statistic for fitting discrete generalized linear models (GLMs) based on the sum of standardized residuals (SSRs). This work is an extension of our earlier work (Chen L. et al. [1]) which proposed a GOF test for binary responses. We derive the asymptotic distribution of the test statistic and show how it can be applied to popular count regression models such as Poisson regression, Negative Binomial regression and Bino- mial regression using different link functions. Using numeric examples we show that the proposed test is substantially more powerful than most of the currently available GOF tests under various model misspecification scenarios applied to various discrete GLMs like logistic, Poisson, and negative binomial. iii Acknowledgments First, I would like to thank my wonderful parents, Daifu and Guiping for their love, trust and encouragement along the way. I would not be where I am today were it not for their love and guidance. I am grateful to my co-advisors, Professor Grzegorz A. Rempala and Professor Chi Song, both of whom have devoted a substantial amount of time and effort in train- ing me to be a better researcher over the past few years. Without their guidance and persistent help this work would not have been possible. Professor Grzegorz A. Rempala is the reason I first became interested in multivariate count modeling, and since then I have thoroughly enjoyed working in this area with him. He has pro- vided me with numerous opportunities to learn and grow through research, teaching, mentoring, and collaboration. I also would like to express appreciation to Professor Chi Song. He is a patient mentor and one of the smartest people I know. Some of my fondest memories in graduate school were hours of research discussions with him in his office. He has also become a good friend, whom I admire professionally and personally. iv I thank the other member of my dissertation committee − Professor Oksana Chkrebtii, and the member of my candidacy committee − Professor Kellie J. Archer for their time and effort in evaluating my work and providing with me feedbacks. Finally, I would also like to thank all the professors and staffs in the program of biostatistics who have provided guidance and support during my time at The Ohio State University. v Vita June 5, 1990 . Born - Jilin, China 2013 . B.S. Biological Science 2015 . M.S. Statistics 2013-present . Graduate Associate, The Ohio State University. Publications Research Publications Chen Lu, Sui Yihan, Song Chi, Grzegorz A. Rempala (2017). The Sum of Stan- dardized Residuals: Goodness of Fit Test for Binary Response Model Statistics in Medicine, doi: 10.1002/sim.7644. Fields of Study Major Field: Biostatistics vi Table of Contents Page Abstract . ii Acknowledgments . iv Vita......................................... vi List of Tables . .x List of Figures . xii 1. Introduction . .1 1.1 Mathematical Modeling of Epidemics . .1 1.1.1 Multivariate Models . .3 1.1.2 Multivariate Poisson (MVP) Distribution . .4 1.2 Generalized Linear Models (GLMs) . .6 1.2.1 Estimating Equations . .7 1.2.2 Goodness of Fit (GOF) Test for GLM . .8 1.3 Thesis Outline . .8 2. Dynamic Multivariate Poisson (DMVP) Model . 11 2.1 Modeling Framework . 11 2.1.1 Non-Dynamic Multivariate Poisson Model . 12 2.1.2 Dynamic Multivariate Poisson (DMVP) Model . 14 2.2 Multivariate Poisson Joinpoint Model . 17 vii 2.3 Bayesian Estimation . 21 2.3.1 The Posterior Distribution for AR(1) Model . 22 2.3.2 The Posterior Distribution for ARIMA(1,1,0) Model . 24 2.3.3 The Estimation of Joinpoint Parameters b ......... 27 2.3.4 The update of Y and ξ .................... 28 2.4 Simulation Studies . 29 2.5 Analysis of Pertussis Data . 32 2.5.1 Background . 33 2.5.2 Modern Resurgence of Pertussis . 36 2.5.3 Modeling Pertussis Trends . 38 2.5.4 Analysis Details . 39 2.5.5 Conclusion . 45 3. The Goodness of Fit (GOF) in Generalized Linear Model (GLM) . 50 3.1 Summary of Previous Research on GOF . 50 3.1.1 GOF Tests for Binary Outcomes . 52 3.1.2 GOF Tests for Discrete Outcomes . 56 3.2 Proposed Test Statistic - Standardized Residuals . 59 3.3 Numerical Examples . 66 3.3.1 Examples for Binary Variables . 67 3.3.2 Poisson Regression Example 1 . 75 3.3.3 Poisson Regression Example 2 . 78 3.3.4 Negative Binomial Example 1 . 79 3.3.5 Negative Binomial Example 2 . 82 3.4 Model Selection Example . 86 3.4.1 Model Selection Example for Binary Variable . 86 3.4.2 Model Discrimination Example for Count Variable . 87 3.5 Discussion . 89 4. Future Work . 91 Bibliography . 94 Appendices 103 viii A. The Sum of Standardized Residuals: Goodness of Fit Test for Binary Response Model . 103 ix List of Tables Table Page 2.1 Simulation results for parameter β and γ in P = 1, Q = 1 . 30 2.2 Simulation results for parameter β and γ in P = 2;Q = 1 . 31 2.3 History of pertussis vaccines . 35 2.4 History of acellular pertussis vaccines . 36 2.5 Pertussis Vaccination Coverage (%) . 48 3.1 Selected variables description in the Boston Marathon data . 68 3.2 Proportion H0 rejected at the α = 0:05 using sample size of 445 with 10000 replications in quadratic models using the Boston Marathon data 69 3.3 Selected variables description in Cleveland data . 71 3.4 Proportion H0 rejected at the α = 0:05 using sample size of 303 with 10000 replications in interaction models using the Cleveland data . 72 3.5 Selected variables description in NHANES data . 73 3.6 Proportion H0 rejected at the α = 0:05 using sample size of 373 with 10000 replications in interaction models using the NHANES data . 74 x 3.7 Selected variables description in the Affairs data (n=601) . 76 3.8 Proportion H0 rejected at the α = 0:05 using sample size of 601 with 10000 replications using the Affairs data . 76 3.9 Selected variables description in the German Credit data (n=1,000) . 80 3.10 Proportion H0 rejected at the α = 0:05 using sample size of 1000 with 10000 replications using the German Credit data . 80 3.11 Selected variables description in the Bad Health data . 83 3.12 Proportion H0 rejected at the α = 0:05 using sample size of 1127 with 10000 replications using the Bad Health data . 83 3.13 Selected variables description in the Fishing data . 85 3.14 Proportion H0 rejected at the α = 0:05 using sample size of 147 with 10000 replications in sqrt link model using the Fishing data . 86 3.15 Selected variables description in HINTS data . 87 3.16 The results of Cn and its p-values under each model using the HINTS data . 88 3.17 Pertussis data . 89 3.18 The results of Cn and its p−values under Poisson and negative bimo- nial model using Pertussis data . 89 xi List of Figures Figure Page 1.1 An overview of mathematical models for infectious diseases generated by Siettos and Russo[2] . .3 2.1 Group sampling illustration . 28 2.2 Pertussis Incidence by Age Group . 37 2.3 Pertussis Incidence Cases . 38 2.4 Pertussis Rate(per 100,000) 2008 . 39 2.5 Pertussis Rate(per 100,000) 2009 . 40 2.6 Pertussis Rate(per 100,000) 2010 . 41 2.7 Pertussis Rate(per 100,000) 2011 . 42 2.8 Pertussis Rate(per 100,000) 2012 . 43 2.9 Pertussis Rate(per 100,000) 2013 . 44 2.10 Pertussis Rate(per 100,000) 2014 . 45 2.11 Pertussis Rate(per 100,000) 2015 . 46 xii 2.12 Pertussis Rate(per 100,000) 2016 . 47 2.13 9 States . 47 2.14 DMVP and Simplified Poisson Model Fitting . 49 3.1 Proportion H0 rejected at the α = 0:05 using sample size of 601 with 10000 replications in interaction models using the Affair data . 77 3.2 Proportion H0 rejected at the α = 0:05 over increase sample size with 10000 replications using the German Credit data .
Details
-
File Typepdf
-
Upload Time-
-
Content LanguagesEnglish
-
Upload UserAnonymous/Not logged-in
-
File Pages127 Page
-
File Size-