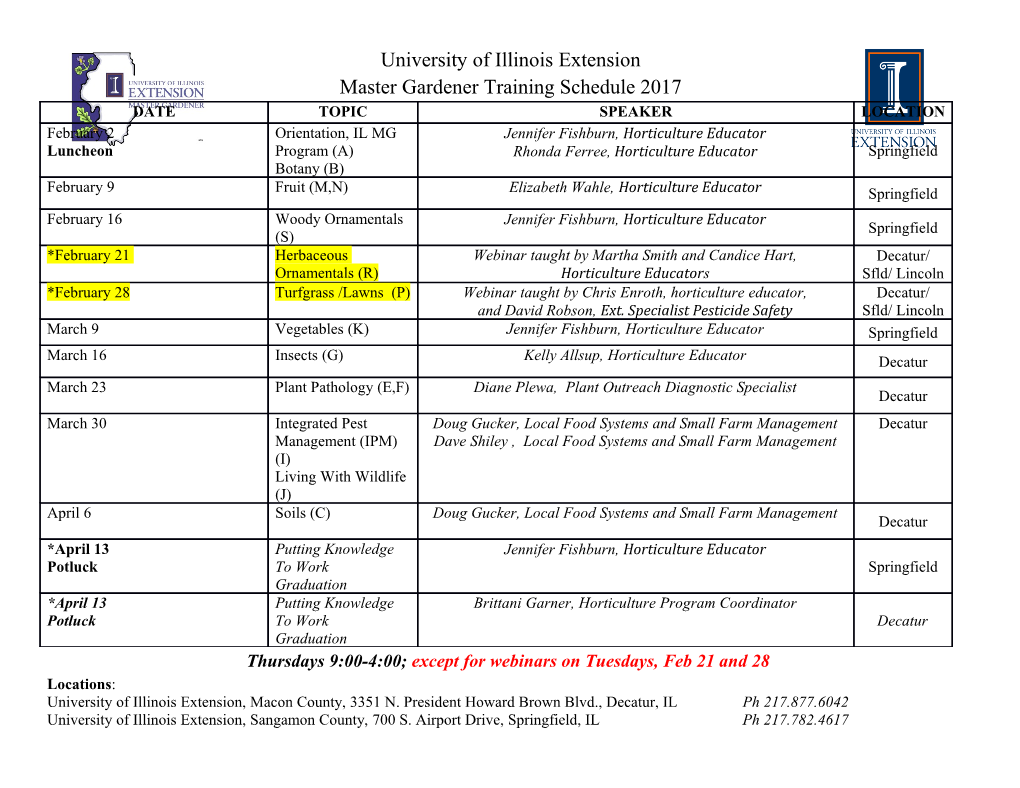
Nearest Neighbor Search in Google Correlate Dan Vanderkam Robert Schonberger Henry Rowley Google Inc Google Inc Google Inc 76 9th Avenue 76 9th Avenue 1600 Amphitheatre Parkway New York, New York 10011 New York, New York 10011 Mountain View, California USA USA 94043 USA [email protected] [email protected] [email protected] Sanjiv Kumar Google Inc 76 9th Avenue New York, New York 10011 USA [email protected] ABSTRACT queries are then noted, and a model that estimates influenza This paper presents the algorithms which power Google Cor- incidence based on present query data is created. relate[8], a tool which finds web search terms whose popu- This estimate is valuable because the most recent tradi- larity over time best matches a user-provided time series. tional estimate of the ILI rate is only available after a two Correlate was developed to generalize the query-based mod- week delay. Indeed, there are many fields where estimating eling techniques pioneered by Google Flu Trends and make the present[1] is important. Other health issues and indica- them available to end users. tors in economics, such as unemployment, can also benefit Correlate searches across millions of candidate query time from estimates produced using the techniques developed for series to find the best matches, returning results in less than Google Flu Trends. 200 milliseconds. Its feature set and requirements present Google Flu Trends relies on a multi-hour batch process unique challenges for Approximate Nearest Neighbor (ANN) to find queries that correlate to the ILI time series. Google search techniques. In this paper, we present Asymmetric Correlate allows users to create their own versions of Flu Hashing (AH), the technique used by Correlate, and show Trends in real time. how it can be adapted to fit the specific needs of the product. Correlate searches millions of candidate queries in under We then develop experiments to test the throughput and 200ms in order to find the best matches for a target time recall of Asymmetric Hashing as compared to a brute-force series. In this paper, we present the algorithms used to search. For \full" search vectors, we achieve a 10x speedup achieve this. over brute force search while maintaining 97% recall. For The query time series consists of weekly query fractions. search vectors which contain holdout periods, we achieve a The numerator is the number of times a query containing a 4x speedup over brute force search, also with 97% recall. particular phrase was issued in the United States in a partic- ular week. The denominator is the total number of queries issued in the United States in that same week. This nor- General Terms malization compensates for overall growth in search traffic Algorithms, Hashing, Correlation since 2003. For N weeks of query data, the query and target time series are N dimensional vectors and retrieval requires a search for nearest neighbors. Keywords A brute force search for the nearest neighbors is typically Approximate Nearest Neighbor, k Means, Hashing, Asym- too expensive (we discuss exactly how expensive below), and metric Hashing, Google Correlate, Pearson Correlation so extensive work has gone into developing ANN algorithms which trade accuracy for speed. Our problem is similar to 1. INTRODUCTION image clustering problems[6], and we examined many of the known tree- and hash-based approaches[5, 4, 7]. In 2008, Google released Google Flu Trends [3]. Flu Trends Google Correlate presents some unique challenges and op- uses query data to estimate the relative incidence of In- portunities for nearest neighbor search: fluenza in the United States at any time. This system works by looking at a time series of the rate of 1. We compare vectors using Pearson correlation. Be- Influenza-Like Illness (ILI) provided by the CDC1, and com- cause vectors may not contain directly comparable quan- paring against the time series of search queries from users tities (i.e. normalized query volume and user-supplied to Google Web Search in the past. The highest correlating data), we prefer to use a distance metric which is in- variant under linear transformations. Pearson correla- 1 Available online at http://www.cdc.gov/flu/weekly/ tion has this property. 2. We require high recall for highly correlated terms. Re- . call is the fraction of the optimal N results returned Figure 1: Correlated time series: Influenza-Like Illness (blue) and popularity of the term \treatment for flu" on Google web search (red). by the system. The original Google Flu Trends model, for Pearson correlation between two time series, namely: which served as a template for Google Correlate, con- cov(u; v) sisted of 45 [3] queries. If we only achieved 10% recall, r(u; v) = (1) we would have to build a Flu model using only four or σuσv Pn five of those queries. This would lead to an unaccept- [(ui − µ(u))(vi − µ(v))] = i=1 (2) able degradation in quality. pPn 2pPn 2 i=1(ui − µ(u)) i=1(vi − µ(v)) 3. We require support for holdout periods. When build- 2.2 Pearson Correlation Distance ing a model, one typically splits the time period into a If two vectors u and v are perfectly correlated, then r(u; v) = training and test (or holdout) set. The training set is 1. A distance function should have a value of zero in this used to build the model and the holdout is used to val- case, so we use the standard definition of Pearson correlation idate it. Holdouts are essential for validating models, distance: so we needed to support them in Correlate. If a time dp(u; v) = 1 − r(u; v) (3) period is marked as being part of a holdout, our algo- rithm should not consider any values from that time 2.3 Vectors with Missing Indices period. Here we formalize the idea of a \holdout" period. We define a vector with missing indices as a pair: 4. While the data set is large (tens of millions of queries N with hundreds of weeks of data each), it is not so large (v; m) where v 2 < ; m ⊂ f1 :::Ng (4) that it cannot be stored in memory when partitioned if an index i 2 m then the value of vi is considered unknown. across hundreds of machines. This is in contrast to a No algorithm which operates on vectors with missing indices service like Google Image Search, where it would not should consider it. be reasonable to store all images on the web in memory. We found this representation convenient to work with, Details on how we choose the tens of millions of queries since it allowed us to perform transformations on v. For can be found in the Correlate Whitepaper[8]. example, we can convert between sparse and dense repre- sentations, without changing the semantics of (v; m). The solution used by Correlate is a form of vector quan- We also define the projection π(v; m) obtained by remov- tization[2] known as \Asymmetric Hashing", combined with ing the missing indices from v. If v 2 <N then π(v; m) 2 a second pass exact search, which we experimentally find to <N−|mj. produce search results quickly and with high recall. 2.4 Pearson Correlation Distance for Vectors with Missing Indices 2. DEFINITIONS Let (u; m) and (v; n) be two vectors with missing indices. Then we define the Pearson correlation distance between 2.1 Pearson Correlation them as: For our distance metrics, we use the standard definition dp((u; m); (v; n)) = dp(π(u; m [ n); π(v; m [ n)) (5) While this distance function is symmetric and nonnegative, it does not satisfy the triangle inequality. Additionally, if dp((u; m); (v; n)) = 0, there is no guarantee that u = v or m = n. 2.5 Recall We measure the accuracy of approximate result sets using recall, the fraction of the ideal result set which appears in the approximate result set. If E is the ideal set of the top k results and A is the actual set of the top k approximate results, the we define jA \ Ej Recall (A; E) = (6) k k Note that the ordering of results is not considered here. Cor- Figure 2: Illustration of how vectors are split into relate swaps in exact distances (this procedure is described chunks below), so if results appear in each set, then they will be in the same order. To index a vector v, we find the centroid closest to v in each chunk. Concretely, we set 3. ASYMMETRIC HASHING Here we present Asymmetric Hashing, the technique used j hi(v) = arg min( πi(v) − ci ) (11) by Correlate to compute approximate distance between vec- tors. We begin by considering the simpler case of Pearson h(v) = (h1(v); h2(v); : : : ; hN=k(v)) (12) correlation distance with \full" vectors, i.e. those without N missing indices. We then adapt the technique for target For each vector this results in k integers between 1 and 256, N vectors which do have missing indices. one for each chunk. We combine the integers into a k byte hash code, h(v). 3.1 Mapping onto Squared Euclidean Distance We can reconstitute an approximation of the original vec- We begin by mapping Pearson correlation distance onto tor from its hash code as: N a simpler function. If u; v 2 < , we can normalize them by h N (v) 2 h1(v) h2(v) k their ` norms and rescale so that Approx(v) = (c1 ; c2 ; : : : ; c N ) (13) k u − µ(u) u0 = (7) 2N ju − µ(u)j As an aside, we note that chunks comprised of consecutive weeks (1-10) typically result in better approximations than v − µ(v) v0 = (8) chunks comprised of evenly-spaced weeks (1; 41; 81;:::; 361).
Details
-
File Typepdf
-
Upload Time-
-
Content LanguagesEnglish
-
Upload UserAnonymous/Not logged-in
-
File Pages8 Page
-
File Size-