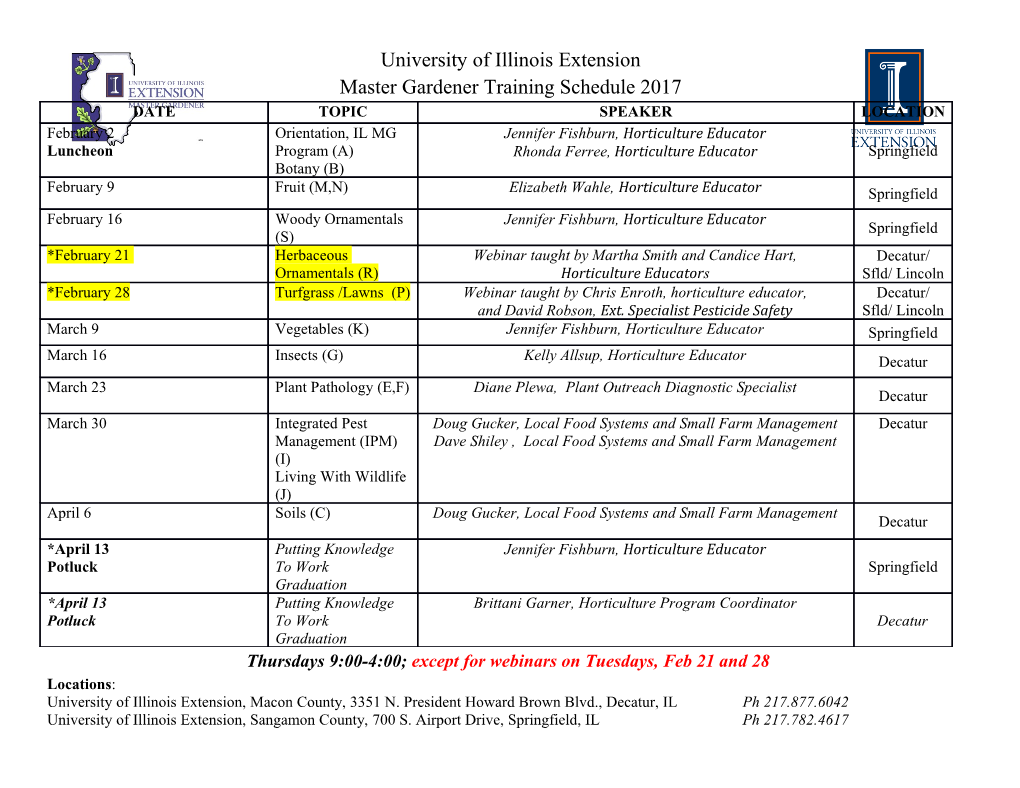
European Journal of Epidemiology https://doi.org/10.1007/s10654-018-0447-z (0123456789().,-volV)(0123456789().,- volV) METHODS A comparison of different methods to handle missing data in the context of propensity score analysis 1 1,2 1,3 Jungyeon Choi • Olaf M. Dekkers • Saskia le Cessie Received: 21 May 2018 / Accepted: 25 September 2018 Ó The Author(s) 2018 Abstract Propensity score analysis is a popular method to control for confounding in observational studies. A challenge in propensity methods is missing values in confounders. Several strategies for handling missing values exist, but guidance in choosing the best method is needed. In this simulation study, we compared four strategies of handling missing covariate values in propensity matching and propensity weighting. These methods include: complete case analysis, missing indicator method, multiple imputation and combining multiple imputation and missing indicator method. Concurrently, we aimed to provide guidance in choosing the optimal strategy. Simulated scenarios varied regarding missing mechanism, presence of effect modification or unmeasured confounding. Additionally, we demonstrated how missingness graphs help clarifying the missing structure. When no effect modification existed, complete case analysis yielded valid causal treatment effects even when data were missing not at random. In some situations, complete case analysis was also able to partially correct for unmeasured confounding. Multiple imputation worked well if the data were missing (completely) at random, and if the imputation model was correctly specified. In the presence of effect modification, more complex imputation models than default options of commonly used statistical software were required. Multiple imputation may fail when data are missing not at random. Here, combining multiple imputation and the missing indicator method reduced the bias as the missing indicator variable can be a proxy for unobserved confounding. The optimal way to handle missing values in covariates of propensity score models depends on the missing data structure and the presence of effect modification. When effect modification is present, default settings of imputation methods may yield biased results even if data are missing at random. Keywords Missing data Á Propensity score analysis Á Multiple imputation Á Missing indicator Á Effect modification Á Missingness graph Introduction & Jungyeon Choi Observational studies potentially suffer from confounding. [email protected] Propensity score methods, first introduced by Rosenbaum Olaf M. Dekkers and Rubin [1], are increasingly being used in medical [email protected] research to handle confounding [2–5]. When the observed Saskia le Cessie baseline characteristics are sufficient to correct for con- [email protected] founding bias and the propensity model is correctly spec- ified, propensity score analysis creates conditional 1 Department of Clinical Epidemiology, Leiden University Medical Center, Albinusdreef 2, C7-P, 2333 ZA Leiden, The exchangeability between persons with the same propensity Netherlands score. Numerous studies provide illustrations and discus- 2 Department of Endocrinology and Metabolism, Leiden sions on the performance of different propensity score University Medical Center, Albinusdreef 2, C7-P, approaches [3, 4, 6–11]. 2333 ZA Leiden, The Netherlands Besides confounding, observational studies often have 3 Department of Biomedical Data Sciences, Leiden University missing values in covariates. Missing values can occur by Medical Center, Albinusdreef 2, C7-P, 2333 ZA Leiden, The different mechanisms: values are missing completely at Netherlands 123 J. Choi et al. random (MCAR) when the probability that a value is Simulation description missing is independent from observed and unobserved information (e.g. a lab measurement is missing, because a We generated simulated data with missing values in one of technician dropped a tube), missing at random (MAR) the confounders and compared effect estimates obtained by where the probability of missing depends only on observed using several different strategies to deal with missing data. information (e.g. lab measurements are only performed In Sect. 2.1 we considered a situation without unmeasured when other measured variables were abnormal), or missing confounding and with the equal treatment effect for all not at random (MNAR) where the probability of missing subjects. In Sect. 2.2, we introduced a heterogeneous depends on unobserved information (e.g. lab measurements treatment effect. In Sect. 2.3, the simulations were exten- are only performed when a doctor judged that a patient was ded by adding unmeasured confounding. in a severe condition, while the severity is not well-regis- tered.) [12]. However, it is difficult to decide on the type of Simulation setting 1: No unmeasured missing mechanism, especially when distinguishing whe- confounding and a homogeneous treatment ther the data are missing at random or not at random effect [13, 14]. Especially in routinely collected data, variables are often selectively measured based on a patient’s char- In this simulation series, for each subject we generated two acteristics which are often not well-specified [15]. If those continuous covariates X1 and X2.X1 follows a normal ill-defined characteristics are associated with the variable distribution of mean 0 and standard deviation of 1. X2 with missing values, data is missing not at random. depended on X1, where for subject i, External knowledge or assumptions about the clinical set- X i ¼ 0:5X i þ ei with ei NðÞ0; 0:75 ting are required to distinguish whether the missing is at 2 1 random or not at random. In this way the standard deviation of X2 is also 1 and the How to estimate propensity scores when there are correlation between X1 and X2 is equal to 0.5. The treat- missing values is a challenge when studying causal asso- ment T was generated from the binomial distribution, with ciations [16]. There are different strategies to handle the probability for subject i to receive the treatment being missing data in a propensity score analysis. The simplest equal to: approach is to discard all observations with missing data, a logitðÞ¼À PðÞ Ti ¼ 1jX1i; X2i 0:8 þ 0:5X1i þ 0:5X2i so-called complete case analysis [12, 17]. Including a missing indicator in a statistical model is another simple In this way about 33% of the generated subjects received method. However, various studies showed that the method treatment. A continuous outcome was generated with the in general introduce bias [18–21]. Multiple imputation is a mean linearly related to X1 and X2: standard method to deal with missing data. Many studies Yi ¼ X1i þ X2i þ ei; with ei NðÞ0; 1 have shown the advantage of multiple imputation and its superiority over other methods [12, 19, 22]. In combination For ease of interpretation of the results, we assumed that with propensity scores, however, several questions arise: the treatment T had no effect on the outcome for any of the Should we include the outcome in the imputation model? subjects. Missing data were generated for 50% of the X2 Can we use the imputation methods implemented in stan- values in three different ways: dard software? How should we combine the results of the • A missing completely at random (MCAR) scenario: different propensity scores estimated in each imputed 50% of values are randomly set to missing in X2. dataset? • A missing at random (MAR) scenario: The higher the The aim of this simulation study is to investigate how value of X1, the more likely for the X2 value to be different strategies of handling missing values of covariates missing. Denoting R as a missing indicator of X2, the in a propensity score model can yield valid causal treat- probability of a missing X2 value was equal to: ment effect estimates. To limit the scope of the study, we deal only with missing values in the baseline characteris- logitðÞ¼ PðÞ Ri ¼ 1 X1i tics, which is a rather common situation happens in rou- • A missing not at random (MNAR) scenario: The higher tinely collected data. We create simulation scenarios the value of X2, the more likely that the value was varying in their missing data mechanisms, presence of missing. The probability of a missing X2 value was: heterogeneous treatment effect and unmeasured con- logitðÞ¼ PðÞ R ¼ 1 X founding. Subsequently, the results are used to provide i 2i guidance in choosing an optimal strategy to handle missing data in the context of propensity score analysis. Missingness-graphs (m-graph, for short) of each missing scenario are depicted in Fig. 1. The missingness graph is a 123 A comparison of different methods to handle missing data in the context of propensity score… X X1 1 X1 T Y T Y T Y X X2 2 X2 X2* X2* X2* R R R (a) (b) (c) Fig. 1 M-graphs for Simulation setting 1: MCAR scenario (a), MAR scenario (b), and MANR scenario (c) graphical tool to represent missing data, proposed by treatment assignment (T) to the outcome (Y), because for Mohan et al. [23]. Guidance for practical users is given in some subjects the treatment has an effect on their outcome. Thoemmes and Mohan [24]. These graphs are extensions to causal directed acyclic graphs (DAGs) where nodes indi- Simulation setting 3: Unmeasured confounding cate covariates and arrows indicate causal relations. When and a homogeneous treatment effect a covariate contains missing values (X2 in our simulations), it is expressed by a dashed rectangle around the node. The In this series of simulations, we assumed an additional node R represents the missingess of X , and can be referred 2 unobserved confounder U, normally distributed with a to as a missing indicator of X . The observed portion of X 2 2 mean of 0 and standard deviation of 1 and independent is represented as X2*. When R = 0, X2* is identical to X2, from X1.X2 depended on X1 and U, where for subject i, and when R = 1, X2* is missing.
Details
-
File Typepdf
-
Upload Time-
-
Content LanguagesEnglish
-
Upload UserAnonymous/Not logged-in
-
File Pages14 Page
-
File Size-