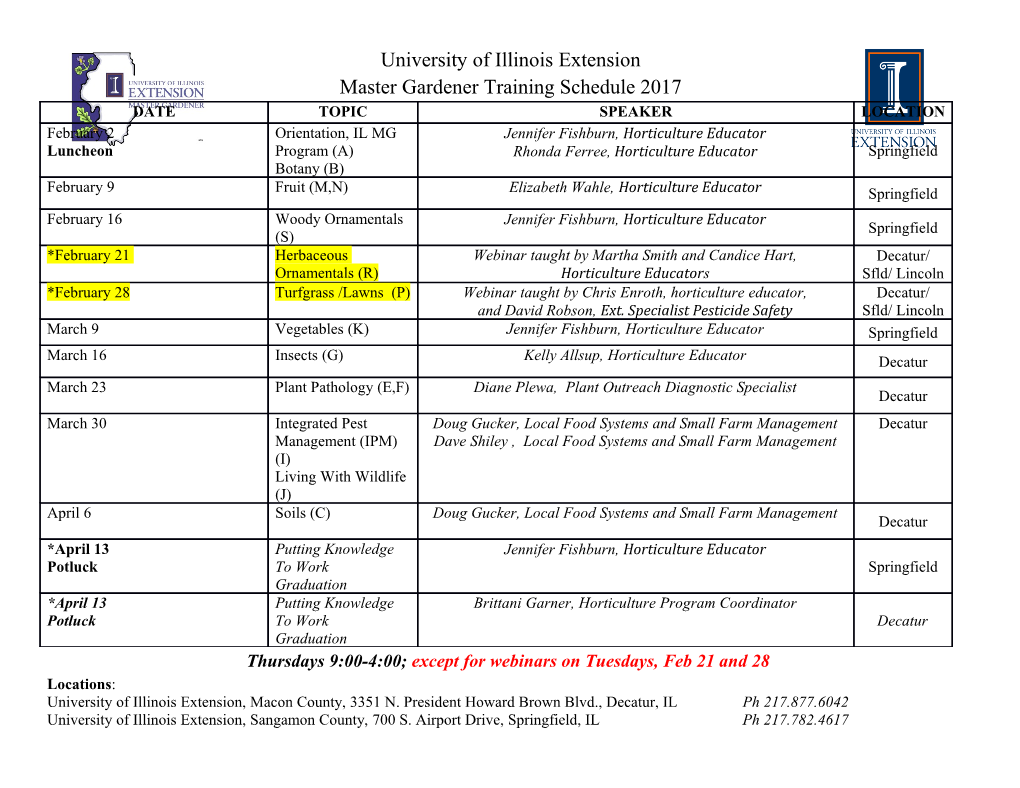
The Fractal Flame Algorithm The Fractal Flame algorithm is a member of the Iterated Function System (IFS) class of fractal algorithms. A two-dimensional IFS creates images by plotting the output of a chaotic system directly on the image plane. The fractal flame algorithm is distinguished by three innovations over text-book IFS: non-linear functions, log-density display, and structural coloring. In combination with standard techniques of anti-aliasing and motion blur the result is striking image variety and quality. Some examples appear in Figure 1. The guiding principle of the design of the algorithm is to expose and preserve as much of the information content of the attractor as possible. I found that preserving information maximizes aesthetics. The paper begins by defining classic, linear iterated function systems and hence grounding our notation and terminology. The classic formulation is then extended with non-linear variations in Section 2. Section 3 describes how log-density display works and why it's important, and Section 4 covers the coloring algorithm. These three sections cover the core innovations. Sections 5 and 7 explain other important properties of the implementation, and Section 6 shows how to create symmetric flames. We conclude with Section 8 on the history and availability of the implementation. 1. Classic Iterated Function Systems 2 2 A two-dimensional Iterated Function System (IFS) is a finite collection of n functions Fi from R to R . The solution of the system is the set S in R2 (and hence an image) that is the fixed point of the recursive set equation: S = Fi(S) i n 0 ≤9< In the formulation defined by Hutchinson [XXX] and popularized by Barnsley [XXX] the functions Fi are linear (technically they are affine as each is a two by three matrix capable of expressing scale, rotate, translate, and shear): Fi(x, y) = (ai x + bi y + ci, di x + ei y + fi) In order to facilitate the proofs and guarantee the algorithms, the functions are constained to be contractive, that is, to bring points closer together. In fact the normal algorithm works under the much weaker condition that the whole system is contractive on average, though we have not formalized this notion. In my practice, no guarantee is given or extracted. Some parameter sets result in better images than others, and some result in degenerate images. The random parameter set generator has a heuristic that avoids the worst, and the rest is left to human discrimination. The normal algorithm for approximating S is called the chaos game. In pseudocode it is: (x, y) = a random point in the biunit square iterate { i = a random integer from 0 to n 1 inclusive . (x, y) = Fi(x, y) plot (x, y) except during the first 20 iterations } The biunit square are those points where x and y are in [-1, 1]. This works because if (x, y) S then Fi(x, y) S too. Though we start out with a random point, because the functions are on average X X contractive the distance between the solution set and the point decreases exponentially. After 20 iterations with a typical contraction factor of 0.5 the point will be within 10-6 of the solution, much less than a pixel's width. Every point of the solution set (or one arbitrarily close to it) will be generated eventually because an infinite string of random symbols contains every finite substring of symbols. This proof is given in more complete form in Section XXX.YYY of [ZZZ]. No fixed number of iterations is given by the algorithm. Because the chaos game operates by stochastic sampling, the more iterations one makes the closer the result will be to the exact solution. The judgement of how close is close enough remains for the user. In my implementation, the number of samples is specified with the more abstract parameter quality, so that image quality as measured by decibels of noise remains constant in face of changes in resolution, zooming, or oversampling. For example, if the functions are x y x + 1 y x y + 1 F (x, y) = , F (x, y) = , F (x, y) = , 0 2 2 1 2 2 2 2 2 é n ï t ï t the result is Sierpinski's Gasket, as seen in Figure 2. 206, spherical 149, sinusoidal 102, swirl 191, horseshoe Figure 1. Example fractal flame images. The numbers are just for identification and indicate the order in which they were discovered. The name is of the variation as described in Section 2. These images were selected for their esthetic properties. Figure 2. Sierpinski's Gasket, a prototypical linear IFS. The axes increase towards the lower-right of the image. It is useful to be able to weight the functions so they are not chosen with equal frequency in line 3 of the chaos game. We assign a weight, or relative probability wi to each function Fi . This is useful for interpolation between function systems with different numbers of functions: the additional functions can be phased in by starting their weights at zero. Differently weighted functions are also necessary for symmetric flames as shown in Section 6. Some implementations of the chaos game make each function's weight proportional to its contraction factor. When drawing one-bit per pixel images this has the advantage of converging faster than equal weighting because it avoids redrawing pixels. But in our context with mutiple bits per pixel and non-linear functions (where the contraction factor is not constant) this device is best avoided. 2. Non-Linear Variations We now generalize this algorithm. The first step is to use a larger class of functions than just affine functions. Composing a non-linear function 2 2 Vj from R to R with the affine functions changes the shape and character of the solution: Fi(x, y) = Vj(ai x + bi y + ci, di x + ei y + fi) We call each such function a variation. The current flame implementation has 14 variations. See Appendix A for a complete description of all the variations. Variation 0 is the identity function. Four more examples are: V1(x, y) = (sinx, siny) sinusoidal 2 2 V2(x, y) = x r , y r spherical a à à á V3(x, y) = (rcos(θ + r), rsin(θ + r)) swirl V4(x, y) = (rcos(2 θ), rsin(2 θ)) horseshoe where r = x2 + y2 θ =2atan(y / x) It is very useful to be able to animate one fractal transforming into another. Such continuous interpolation between arbitrary parameter sets requires another generalization: replacing the integer parameter j with a blending vector of length j . Then Fi(x, y) = vi j Vj(ai x + bi y + ci, di x + ei y + fi) j 0 ≤P<14 where vi j = 1 j P 3. Log-Density Display The chaos game produces a series of (x, y) points which are plotted on the image plane. The collection of these points approximates the solution S to the iterated function system. S is a subset of the plane, and hence membership is a binary function, and the image is therefore black and white, lacking even shades of gray. See Figure 3a for an example. Information is lost every time we plot a point that has already been plotted. A more interesting image can be produced if we render a histogram of the chaotic process, that is, increment a counter at each pixel instead of merely plotting. These counters can be visualized by mapping them to shades of gray or by using a color-map that represents different densities (larger count values are more dense) with different colors. A linear mapping of counters to gray values results in Figure 3b. The result is unsatisfying because of the large dynamic range of densities. Like many natural systems, the densities are often distributed according to a power law (frequency is inversely proportional to value). Figure 4 has two histograms demonstrating this. The densest points are much denser than the average density, hence with a linear map most of the image is very dark, and information is lost. Figure 4. Plots showing that the distribution of densities in an IFS follows the power law. The density (on the horizontal axis) is the number of hits by the system in a pixel, the frequency (on the vertical axis) is the number of pixels with that density (or up to the next power of two). The line is from the image in Figure 3, the other is the composite of 19 favorite systems including Figure 3. In each case, after a plateau the graph is nearly a straight line in log-log space. This is the definitive characteristic of a power law distribution. The flame algorithm solves the problem by using a logarithmic map from density to brightness. See Figure 3c for the result. The logarithm allows one to visually distinguish between densities of 3,000 and 5,000 in one part of the image and 30 and 50 in another part. This log-density mapping is the source of a 3D illusion. On sight people often guess that fractal flames are rendered in 3D, but as just described the algorithm works strictly with a 2D buffer. However, where one branch of the fractal crosses another, one may appear to occlude the other if their densities are different enough because the lesser density is inconsequential in sum. For example branches of densities 1000 and 100 might have brightnesses of 30 and 20. Where they cross the density is 1100, whose brightness is 30.4, which is hardly distinguishable from 30. The display of high dynamic range images is a sub-field of computer graphics unto itself [XXX].
Details
-
File Typepdf
-
Upload Time-
-
Content LanguagesEnglish
-
Upload UserAnonymous/Not logged-in
-
File Pages15 Page
-
File Size-