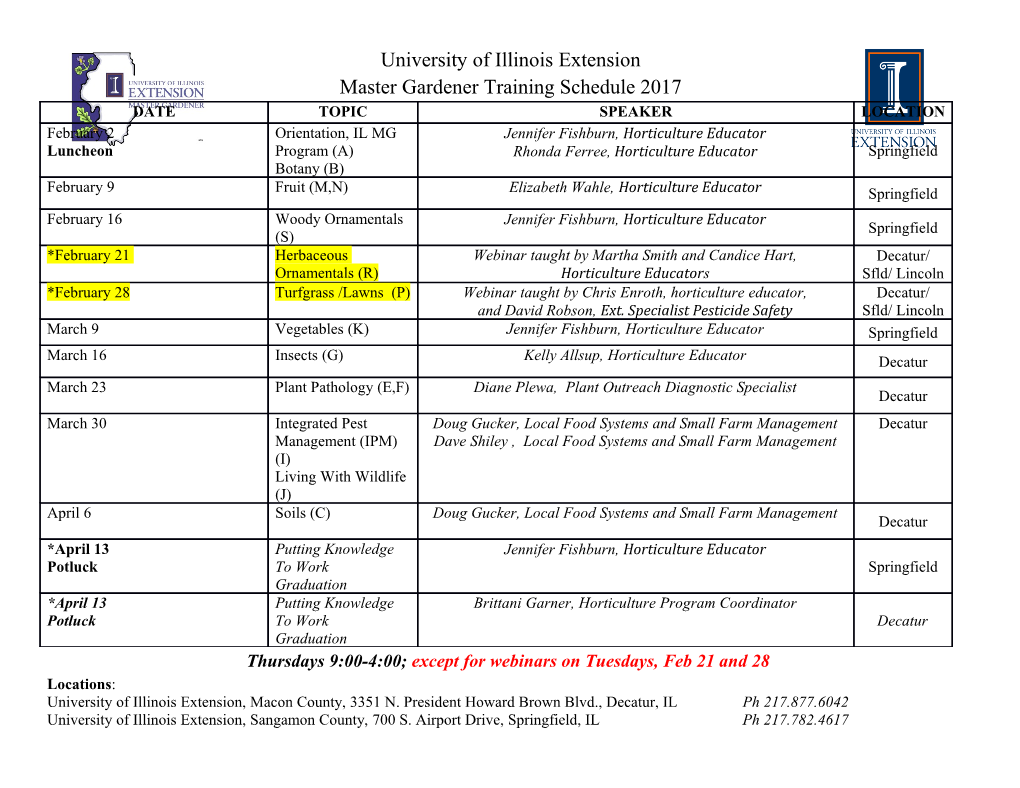
Symbolic Lagrangian Multibody Dynamics Brian Guenter∗ Sung-Hee Lee† Microsoft Research Honda Research Institute Abstract in the Euler-Lagrange equations2 have prevented wide accep- tance of the approach [Herbert Goldstein 2001]. To over- Symbolic Lagrangian formulations of the equations of mo- come this difficulty, researchers have manually derived ef- tion of tree structured constrained mechanical systems have ficient differentiation methods of the Euler-Lagrange equa- the potential to be both more efficient and more numerically tions (see [Featherstone and Orin 2000] for an overview), but robust than formulations which use nonlinear kinematic con- most algorithms assume a small set of simple constraint types straint equations. We derive a simple recursive factorization such as revolute or prismatic joints and thus, not surprisingly, of the Lagrangian equations of motion which, along with our most of the current dynamics packages support only simple extended implementation of the D* symbolic differentiation types of constraints.3Notably, an improved version of the Ar- algorithm, yields empirically measured O(n) inverse dynam- ticulated Body Algorithm [Featherstone 1987] supports gen- ics and O(n3) forward dynamics. Complex kinematic con- eral scleronomic constraints, but the complexity of the algo- straints such as point on surface, point on curve, and surface rithm makes it difficult to understand and implement [Baraff on surface are easily handled by the new algorithm. Numer- 1996]. If arbitrary parametric kinematic constraints are al- ous examples demonstrate the wide range of mechanical sys- lowed then computing the derivatives is still problematic. tems the algorithm can be applied to. Computer graphics researchers have used symbolic or auto- matic differentiation to compute derivatives of complex func- CR Categories: I.3.7 [COMPUTER GRAPHICS ]: Three- tions [Kass 1992; Cohen 1992; Popovic´ et al. 2000; Grin- Dimensional Graphics and Realism—Animation spun et al. 2003]. In this paper, we create symbolic equa- tions of motion by using symbolic differentiation. Specifi- 1 Introduction cally, we derive a simple recursive factorization of the La- grangian equations of motion which, along with our extended Lagrangian mechanics is a reformulation of classical me- implementation of the D* symbolic differentiation algorithm [Guenter 2007], yields empirically measured O(n) inverse chanics which is particularly useful for modeling constrained 3 mechanical systems. For tree structured systems, with holo- dynamics and O(n ) forward dynamics. (Note to review- nomic constraints that can be expressed as differentiable ers: D* symbolic differentiation software will be available to parametric functions of the generalized coordinates1, it is al- public in late 2009.) ways possible to find a set of symbolic differential equations In mechanics, symbolic or automatic differentiation have for the Lagrangian equations of motion. By contrast, non- been employed mostly to augment multibody dynamics such Lagrangian formulations require differential equations and as computing the derivatives of constraint equations or the nonlinear algebraic equations (abbreviated DAE) [Ascher mass matrix [Shi and McPhee 2000; Olsson et al. 2005; and Petzold 1998] to enforce kinematic constraints. Solv- Samin and Fisette 2003]. Not only is symbolic differenti- ing DAE systems is considerably more difficult than solving ation used for dynamics simulation, but it is also used for differential equations alone. linearizing the equations of motion and optimizing design parameters. However, for the core simulation of the dynam- However, the difficulty of computing the complex derivatives ics, they employed existing, manually derived dynamics al- gorithms that do not require symbolic differentiation. ∗email:[email protected] † email:[email protected] In contrast, we derive symbolic equations of motion by us- ing symbolic differentiation. [Villard and Arnaldi 1996] ap- plied symbolic differentiation to compute the equations for the constraint forces, which are numerically calculated using either the penalty method or the Lagrange mutltiplier tech- 2For tree structured systems naïve direct approaches for evaluating these derivatives can have computational complexity as high as O(n4), where n is the number of degrees of freedom of the system. 3SD/FAST [Hollars et al. 1994], a multibody dynamics package based on Lagrangian formulation, supports complex, user-defined constraints only by 1This may sound restrictive but a wide variety of mechanisms can be using the Lagrange multipler technique, which is not related to our symbolic modeled this way, as we will show in Section 5. Lagrangian approach. nique. By contrast, our algorithm generates minimal coor- dinate motion equations that eliminate all constraint forces. p [Turner 2003] applied their automatic differentiation algo- w tcm rithm to Lagrange’s equation to generate motion equations r rigid body frame rcm for very simple mechanisms, but they did not generalize their t method to handle a broad scope of mechanisms. W One advantage of using symbolic differentiation for dynam- ics simulation is that one can easily incorporate complex world frame constraints. In general, one needs to compute derivatives of the constraint equations up to the second order. Com- Figure 1: rcm and tcm is the center of mass with respect to plex constraints have very complex derivatives, which are the world and body frame, respectively. rw and p refer to difficult to derive. By employing symbolic differentiation, a point of a rigid body with respect to the world and body one need only provide the constraint equations; the deriva- frame, respectively. tives are computed by the symbolic differentation. Section 5 demonstrates that some constraints whose derivatives are very complex to derive manually can be easily simulated by 2 Lagrangian Equations of Motion our method. Some of our example mechanical systems are similar to those presented in [Kry and Pai 2003] and [Lee We start by deriving the Langrangian equations of mo- and Terzopoulos 2008] but the dynamics algorithms to cre- tion of a multibody system. We assume a tree structured ate the examples are very different in that the former manu- multibody system in which n generalized coordinates, q = ally derived derivatives of the general surface constraints and (q0;:::;qn−1), completely specify the configuration of every the latter employed easily differentiable constraint functions, rigid body in the system. Each local transformation for rigid both under the framework of existing dynamics algorithms. body i, Ai, with respect to its parent is determined by some number of generalized coordinates qi, and its transformation W in world coordinates is expressed as the product of local 1.1 Contributions i transformations of its ancestors, or in recursive form, as: To address the problem of applying symbolic Lagrangian me- R(qi) t(qi) Wi(q) = = Wp (q)Ai(qi) (1) chanics to tree structured systems we present in this paper a 0 1 i simple and efficient factorization of the symbolic Lagrangian where p denotes i’s parent. equations of motion which directly leads to an O(n) algo- i rithm for inverse dynamics. For the forward dynamics prob- If the inertia matrix is defined in the center of mass coordi- lem we have extended our implementation of the D∗symbolic nate frame then the Newton-Euler equations of motion for a differentiation algorithm [Guenter 2007] to give an O(n3) al- system of n rigid bodies are: gorithm. The new algorithm has several attractive properties: n n ∑ mir¨cmi = ∑ fi (2) i=1 i=1 ∙ Because the symbolic Lagrangian equations always n n ˙ maintain kinematic constraints it is possible, in some ∑ hcmi = ∑ ti (3) cases, to use very large time steps. These large time i=1 i=1 steps would cause convergence problems for nonlinear where mi is the mass of a rigid body i and rcmi = Witcmi is its constraint solvers. center of mass (Fig. 1). hcmi = Iiwi is the angular momentum about the center of mass where Ii is the rotational inertia and ^T ˙ 4 ∙ Mechanisms incorporating complex constraints which wi = Ri Ri . The force fi and torque ti include external and are challenging to simulate using existing techniques, internal forces and torques, respectively; i.e., fi = fexti + finti such as point on surface, point on curve, surface on sur- and ti = texti + tinti . By projecting the Newtonian motion face, are easily and robustly simulated. equations onto the kinematic constraint manifold we obtain the Lagrangian equations of motion, which completely elim- ¶r inate constraint forces ; i.e., we project (2) onto cmi . Con- The remainder of this paper is organized as follows: after de- ¶q j riving the Lagrangian equations of motion from the Newton- straint forces have zero projection on this vector so they all Euler dynamics equation (Section 2), we present a simple re- 4The ~ operator converts between vector and a skew-symmetric matrix cursive factorization of the Lagrangian equation (Section 3). representation; i.e., an angular velocity w relates to the time derivative of the Then we show inverse and forward dynamics methods (Sec- rotation matrix R as follows: 2 3 tion 4) followed by numerous examples (Section 5). Finally, 0 −wz wy ˜ = − ; ]T ˙ = : we report the performance of our methods (Section 6) and w 4 wz 0 wx 5 R R w −wy wx 0 conclude the paper (Section 7). vanish per d’Alembert’s principle of virtual work: To simplify these equations start with two observations. First, because5 n n ¶Ai ¶rcm ¶rcm = 0 i 6= j (7) (m r¨ − f )T i = fT i ∑ i cmi exti ∑ inti ¶q j i=1 ¶q j i=1 ¶q j n we can rewrite ¶Wi as T ¶rcmi ¶q j ∑ ai = Qf j (4) i=1 ¶q j ¶Wi ¶A j = W j−1 A j+1 :::Ai (8) ¶q j ¶q j where ai = mir¨cmi −fexti and Qf j is the generalized force, due to forces, associated with generalized coordinate q j. Like- Second ¶W wise, we project the torques onto the constraint manifold: j = 0 for k ≥ j ¶qk n n T where the expression k ≥ j means that the rigid body k is h˙ − t w = tT w ∑ cmi exti q j ∑ inti q j further toward the leaves of the tree than the rigid body j.
Details
-
File Typepdf
-
Upload Time-
-
Content LanguagesEnglish
-
Upload UserAnonymous/Not logged-in
-
File Pages9 Page
-
File Size-