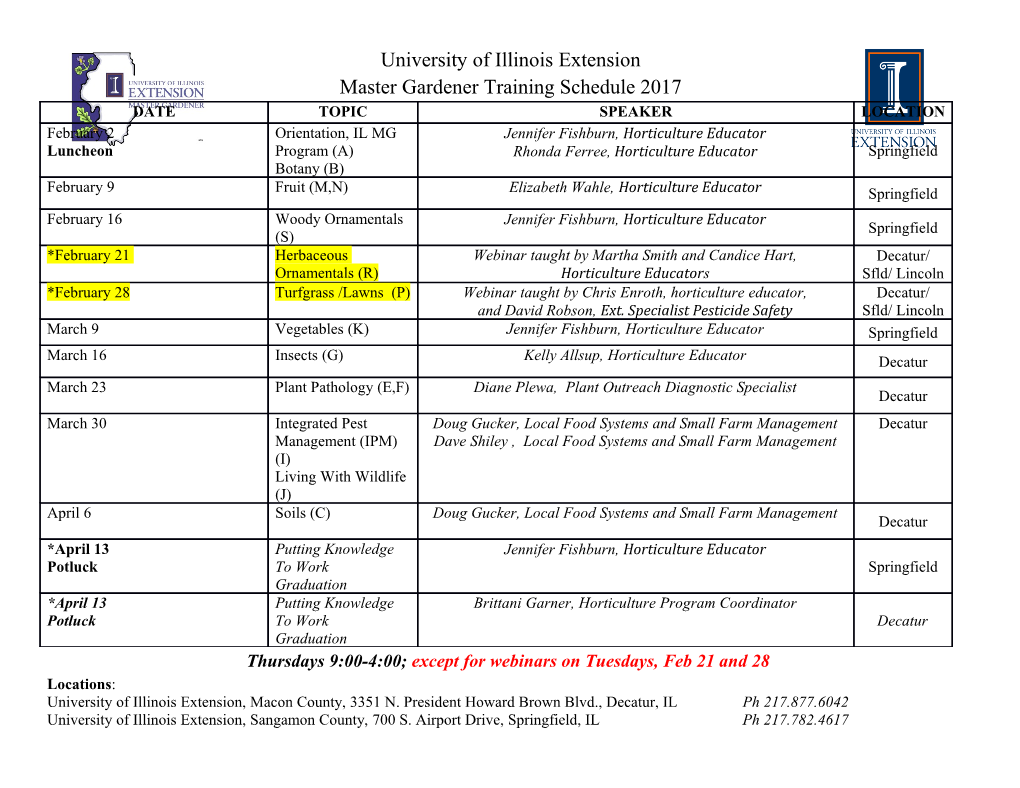
ORIGINAL ARTICLE Identification of Novel Type 1 Diabetes Candidate Genes by Integrating Genome-Wide Association Data, Protein-Protein Interactions, and Human Pancreatic Islet Gene Expression Regine Bergholdt,1 Caroline Brorsson,2 Albert Palleja,3 Lukas A. Berchtold,1 Tina Fløyel,2 Claus Heiner Bang-Berthelsen,2 Klaus Stensgaard Frederiksen,4 Lars Juhl Jensen,3 Joachim Størling,2 and Flemming Pociot2,5 , 3 28 Genome-wide association studies (GWAS) have heralded a new (P 5 10 ) used in GWAS. Thus, it is possible that era in susceptibility locus discovery in complex diseases. For many GWAS single nucleotide polymorphisms (SNPs) type 1 diabetes, .40 susceptibility loci have been discovered. having low or moderate risk in themselves interact to However, GWAS do not inevitably lead to identification of the confer a significant combined effect. Therefore, to under- gene or genes in a given locus associated with disease, and they stand disease pathogenesis from GWAS, it is important to do not typically inform the broader context in which the disease analyze the data in the context of complementary types of genes operate. Here, we integrated type 1 diabetes GWAS data follow-up analyses, such as related protein module analysis with protein-protein interactions to construct biological networks fi of relevance for disease. A total of 17 networks were identified. and expression pro ling, under conditions relevant for the To prioritize and substantiate these networks, we performed ex- disease. pressional profiling in human pancreatic islets exposed to proin- The familial clustering of type 1 diabetes, in contrast to flammatory cytokines. Three networks were significantly enriched most other complex diseases, can be explained almost com- for cytokine-regulated genes and, thus, likely to play an important pletely by multiple common variants, each predisposing a role for type 1 diabetes in pancreatic islets. Eight of the regulated modest risk and most likely affecting certain important mo- genes (CD83, IFNGR1, IL17RD, TRAF3IP2, IL27RA, PLCG2, lecular processes (5). The estimated proportion of heritability MYO1B,andCXCR7) in these networks also harbored single nu- explained by currently identified loci is .80% (6). Thus, it is cleotide polymorphisms nominally associated with type 1 diabetes. Finally, the expression and cytokine regulation of these new can- timely to implement additional approaches to translate gene- didate genes were confirmed in insulin-secreting INS-1 b-cells. Our tic observations into possible disease mechanisms. results provide novel insight to the mechanisms behind type 1 Network- or pathway-based approaches have been used diabetes pathogenesis and, thus, may provide the basis for the to identify multiple disease genes for various diseases design of novel treatment strategies. Diabetes 61:954–962, 2012 (7–12). This includes enrichment in predefined pathways by, for example, Kyoto Encyclopedia of Genes and Genomes (KEGG) (13) (http://www.genome.jp) and Gene Ontology (GO) terms (14) (http://www.geneontology.org). Further- enome-wide association studies (GWAS) have more, data suggest that differentially expressed network been successful in susceptibility locus discov- markers are more accurate disease predictors compared ery in complex diseases. For type 1 diabetes, with single gene markers (11,15). For this reason, it has .40 susceptibility loci have been discovered G– been advocated that analysis at the pathway, network, or (1 3). However, GWAS do not necessarily identify the fi protein complex level is the next step in the process of speci c gene or genes in a given locus responsible for the GWAS data mining (16). In addition, best targets for novel association with disease, and they do not typically inform prevention or treatment strategies may not per se be found the broader context in which the disease genes operate (4). among the disease-associated genes but may be inter- GWAS on their own provide limited insights into the mo- action partners in the “disease networks” and, thus, would lecular mechanisms driving disease. This could be partly not be identified by the use of classical approaches. explained by the stringent genome-wide significance level The hypothesis behind this study was that integration of GWAS data with protein-protein interactions and gene From the 1Hagedorn Research Institute, Gentofte, Denmark; the 2Glostrup expression would facilitate a systems-based understanding Research Institute, Glostrup University Hospital, Glostrup, Denmark; the of type 1 diabetes pathogenetic mechanisms (17). We took 3Novo Nordisk Foundation Center for Protein Research, University of Co- penhagen, Copenhagen, Denmark; 4Novo Nordisk A/S, Bagsværd, Denmark; a focused approach using only proteins from GWAS and the 5Clinical Research Center/University Hospital Malmö, University of regions as input proteins for generating protein networks. Lund, Malmö, Sweden. For this purpose, we used the STRING database (18), Corresponding author: Flemming Pociot, [email protected]. fi Received 21 September 2011 and accepted 27 December 2011. which is built on data from several sources. The identi ed DOI: 10.2337/db11-1263 networks were subjected to transcript profiling in cytokine- This article contains Supplementary Data online at http://diabetes exposed human islets, a well-established in vitro model of .diabetesjournals.org/lookup/suppl/doi:10.2337/db11-1263/-/DC1. R.B. and C.B., and L.A.B. and T.F., respectively, contributed equally to this type 1 diabetes pathogenesis (19). Finally, we assigned nom- study. inally associated GWAS SNPs to genes in the identified Ó 2012 by the American Diabetes Association. Readers may use this article as protein networks to test association of individual nodes and long as the work is properly cited, the use is educational and not for profit, and the work is not altered. See http://creativecommons.org/licenses/by validated the cytokine regulation of key candidate genes in -nc-nd/3.0/ for details. insulin-secreting INS-1 cells. 954 DIABETES, VOL. 61, APRIL 2012 diabetes.diabetesjournals.org R. BERGHOLDT AND ASSOCIATES RESEARCH DESIGN AND METHODS significantly regulated (P , 0.05, adjusted for multiple testing by FDR) (26). fi Protein networks. A total of 395 positional candidate genes were identified Enrichment scores for signi cantly regulated genes within the networks from non-HLA type 1 diabetes–associated linkage disequilibrium (LD) regions compared with the Affymetrix microarray were calculated by Fisher exact from GWAS. The LD intervals were calculated based on the HapMap CEU test. founders data in snpMatrix (http://www.bioconductor.org/packages/release/ Mapping SNPs to genes. To evaluate whether the networks included non- – bioc/html/snpMatrix.html) using different Dand r2 thresholds, modified from input genes that harbored type 1 diabetes associated SNPs, we used the meta- (1,2). The positional candidate genes were used as input into the STRING analysis GWAS data generated by the Type 1 Diabetes Genetics Consortium fi database (http://string-db.org/) to build protein networks (Fig. 1). In STRING, (T1DGC) (1). The SNPs in the GWAS dataset were rst mapped to Ensembl the functional associations are derived from four sources: genomic context, gene IDs (mapping based on National Center for Biotechnology Information high-throughput experiments, conserved coexpression, and text mining, while build 36) and then mapped to proteins within the 17 networks. We included only the minimal promoter and 3 -untranslated region (i.e., 2 kilobases [kb] up- the physical interactions are derived from only the high-throughput experi- 9 and downstream, respectively, from the coding region) to reduce overlap ments. Protein networks were built running the Markov CLustering (MCL) between genes and, thus, SNPs that mapped onto more than one gene. SNPs algorithm using the functional and physical interaction scores from STRING located .2 kb from a gene or in gene deserts were not considered in the with an inflation parameter of 4.5 and forcing all the nodes to be connected. present analysis. Networks 8 and 9 did not contain any genes outside the type MCL is a fast, scalable algorithm for unsupervised clustering of graphs and 1 diabetes–associated regions, and these networks were therefore not ana- networks, which has been used by many groups to build protein complexes or lyzed further. families based on protein-protein interactions (20,21). To build meaningful GO term and KEGG pathway enrichment. To assess whether the individual networks, only the protein networks that contained input proteins encoded by networks were significantly enriched for specific GO terms or KEGG pathways, genes from at least two different type 1 diabetes–associated loci were con- we used FatiGO (27). For each of the networks 1 to 11, which are based on sidered. We obtained 11 networks of proteins that interact functionally (net- functional interactions, the set of member proteins was compared with the works 1–11) and 6 networks of proteins that interact physically (networks entire set of proteins from those networks (in total, 147 proteins). Likewise, 12–17). These networks were produced in STRING and recolored in Inkscape every set of proteins from networks 12 to 17 was compared with the whole set (www.inkscape.org). The edges that connected proteins that physically in- of proteins that belong to the networks based on only experimental inter- teract were highlighted in the networks that were differentially regulated actions (in total, 88 proteins). (Figs. 2 and 3). All
Details
-
File Typepdf
-
Upload Time-
-
Content LanguagesEnglish
-
Upload UserAnonymous/Not logged-in
-
File Pages9 Page
-
File Size-