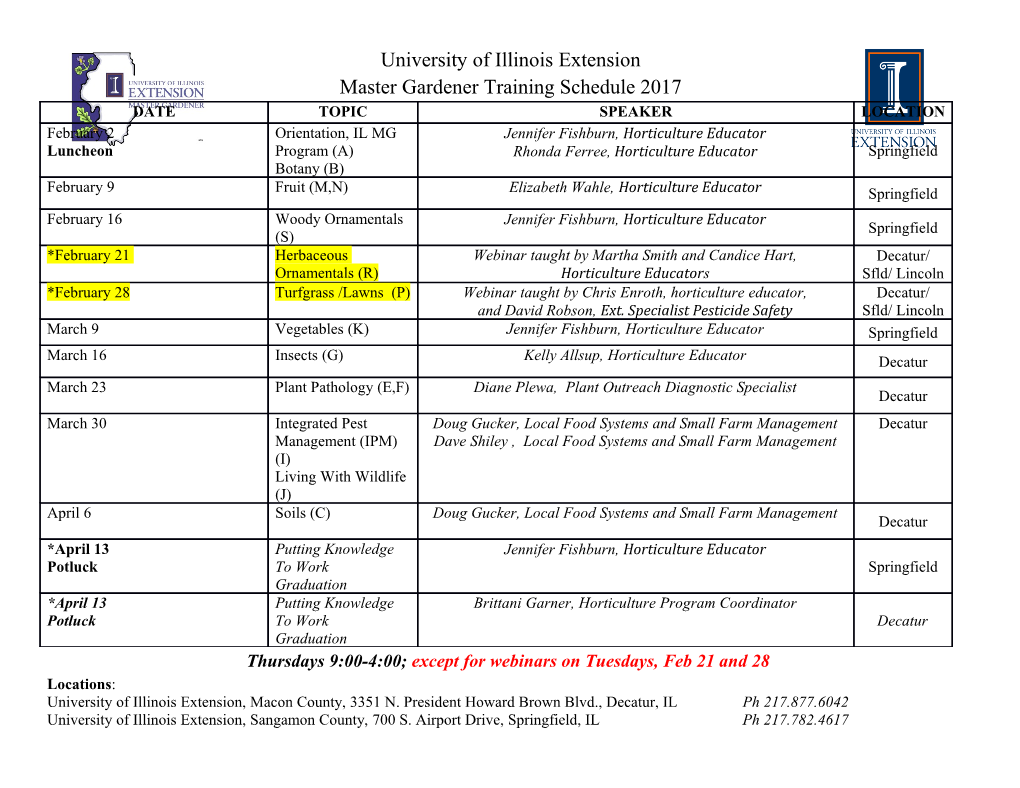
ARTICLE https://doi.org/10.1038/s41467-019-11443-y OPEN TeraVR empowers precise reconstruction of complete 3-D neuronal morphology in the whole brain Yimin Wang 1,2,3,14,QiLi2, Lijuan Liu1, Zhi Zhou1,4, Zongcai Ruan1, Lingsheng Kong2, Yaoyao Li5, Yun Wang4, Ning Zhong6,7, Renjie Chai8,9,10, Xiangfeng Luo2, Yike Guo11, Michael Hawrylycz4, Qingming Luo12, Zhongze Gu 13, Wei Xie 8, Hongkui Zeng 4 & Hanchuan Peng 1,4,14 1234567890():,; Neuron morphology is recognized as a key determinant of cell type, yet the quantitative profiling of a mammalian neuron’s complete three-dimensional (3-D) morphology remains arduous when the neuron has complex arborization and long projection. Whole-brain reconstruction of neuron morphology is even more challenging as it involves processing tens of teravoxels of imaging data. Validating such reconstructions is extremely laborious. We develop TeraVR, an open-source virtual reality annotation system, to address these challenges. TeraVR integrates immersive and collaborative 3-D visualization, interaction, and hierarchical streaming of teravoxel-scale images. Using TeraVR, we have produced precise 3-D full morphology of long-projecting neurons in whole mouse brains and developed a collaborative workflow for highly accurate neuronal reconstruction. 1 Southeast University – Allen Institute Joint Center, Institute for Brain and Intelligence, Southeast University, Nanjing 210096, China. 2 School of Computer Engineering and Science, Shanghai University, Shanghai 200444, China. 3 Shanghai Institute for Advanced Communication and Data Science, Shanghai University, Shanghai 200444, China. 4 Allen Institute for Brain Science, Seattle 98109, USA. 5 School of Optometry and Ophthalmology, Wenzhou Medical University, Wenzhou 325027, China. 6 Beijing University of Technology, 100124, Beijing, China. 7 Department of Life Science and Informatics, Maebashi Institute of Technology, Maebashi 371-0816, Japan. 8 Institute of Life Sciences, Southeast University, Nanjing 210096, China. 9 Key Laboratory for Developmental Genes and Human Disease, Ministry of Education, Institute of Life Sciences, Jiangsu Province High-Tech Key Laboratory for Bio-Medical Research, Southeast University, Nanjing 210096, China. 10 Co-Innovation Center of Neuroregeneration, Nantong University, Nantong 226019, China. 11 Data Science Institute, Imperial College London, London SW7 2AZ, UK. 12 Britton Chance Center for Biomedical Photonics, Wuhan National Laboratory for Optoelectronics, Huazhong University of Science and Technology, Wuhan 430074, China. 13 School of Biological Science and Medical Engineering, Southeast University, Nanjing 210096, China. 14These authors contributed equally: Yimin Wang, Hanchuan Peng. Correspondence and requests for materials should be addressed to Y.W. (email: [email protected]) or to H.P. (email: [email protected]) NATURE COMMUNICATIONS | (2019) 10:3474 | https://doi.org/10.1038/s41467-019-11443-y | www.nature.com/naturecommunications 1 ARTICLE NATURE COMMUNICATIONS | https://doi.org/10.1038/s41467-019-11443-y ajor international initiatives are underway to profile and from such images. Reconstructing geometrical objects from 3-D Mcharacterize cell types of the mammalian brain1,2.Asa volumetric images requires overlaying these objects onto the key recognized attribute of cell type since Ramon y imaging data in 3-D space and manipulating them in situ. Since Cajal, high-fidelity reconstruction of neuron morphology is most current computer displays (e.g., computer screens) and data gaining increased attention3–5. The basic building blocks of the interaction tools (e.g., computer mouse) are still restricted to 2-D, brain, neurons and glial cells, are often noted for their remarkable it is usually hard to observe and manipulate higher dimensional three-dimensional (3-D) shapes that distinguish one cell type data via a lower dimensional interface. It is also desirable to from another. While such shapes are critical to understanding cell interact with the data directly using a smooth workflow. Appli- type, function, connectivity, and development6, it is challenging cations such as Virtual Finger18 represent progress toward this to profile these shapes precisely. Sparse labeling and high- goal, but improvement is still necessary for complex and large resolution micro-imaging of a brain cell help visualize the neurons and also for display and interaction hardware. appearance of the cell, yet it remains a major bottleneck how to Finally, it is often necessary but very expensive to involve convert such imaging data into a digital description of mor- multiple annotators to produce gold-standard reconstructions. phology, including the 3-D spatial locations of a cell’s parts and Manual work is time-consuming and tedious, thus in practice their topological connections. This conversion process is often most existing studies can afford only one annotator per neuron. called neuron tracing or neuron reconstruction and it has become To resolve any ambiguity of reconstructions, it is desired to have a an essential and active area of neuroinformatics. way to allow multiple annotators to visualize the same neuron Two complementary reconstruction workflows exist: one for and its underlying imaging data at the same time, and collaborate electron microscopy (EM) images and the other for light on the work. This approach requires collaborative and immersive microscopy (LM) data7–9. EM offers nanometer resolution and annotation of multi-dimensional imaging data at the whole- thus provides a way to reconstruct the entire surface of the shape, brain scale. but it is often constrained to relatively small brain regions. When Here we consider using virtual reality (VR) techniques. While whole-brain scale is the focus and complete neuron morphology there have been commercial VR systems (e.g., arivisVR) and is desired, LM is a more suitable imaging modality where data are research software 19,20, it is not straightforward to use any of typically acquired at sub-micrometer resolution. LM reconstruc- these existing work to tackle the above challenges. We introduce tion makes it possible to trace both long projections and the the TeraVR system addressing the above requirements. We terminal arborization of a brain cell. Recent extension of this demonstrate the applicability of TeraVR to challenging cases of approach based on expansion microscopy can help visualize whole mouse brain neuron reconstruction, achieving previously neurons at nanometer resolution using LM approaches10. unattainable accuracy and efficiency. It is widely recognized that manual and semi-automatic neu- ron-tracing methods are crucially required to produce full reconstructions, which can also serve as gold-standard datasets to Results develop fully automatic neuron-tracing methods9,11–14. Without TeraVR platform. We developed TeraVR (Fig. 1, Supplementary loss of generality below, we define any neuron-tracing method Note 1, and Supplementary Movies 1–11), an open-source VR that has a non-negligible human labor component as manual software package for the visualization and annotation of reconstruction, which clearly also includes many semi-automatic teravoxel-scale whole-brain imaging data (Fig. 1a). The software methods. This paper discusses a technology that makes such LM- uses the TeraFly16 module of Vaa3D (http://vaa3d.org) to manage oriented manual reconstruction more efficient and reliable than data input–output (I/O), thus TeraVR can streamline the data I/O existing approaches. This work was motivated by four difficulties and other real-time user interaction with teravoxel-scale image detailed below: (1) observability, (2) big data handling, (3) volumes, for example, an 18.4-teravoxel brain image in Fig. 1a. As interaction, and (4) validation. described below, TeraVR is much more than a simple extension First, a neuron can have a very complex 3-D shape that may of TeraFly. Indeed, it has a number of unique features designed contain hundreds or even thousands of fiber branches, especially for reconstruction of neuron morphology in whole-brain images, in dense arbors. Such a high degree of mutual occlusion makes it at different levels of details and at different local regions of hard to see how neurite fibers wire together. The observability is interest (ROI). further compromised by the uneven or weak axon labeling, To use TeraVR, a user wears a VR headset (bottom right relatively poor Z-resolution from imaging, and so on. Often, of Fig. 1a) and works within a virtual space defined for the neither the prevailing 2-D cross-sectional view (such as those brain image along with the neuron reconstruction and other widely used in EM-oriented and many LM software packages) location references on the image. TeraVR generates synchronized nor the typical 3-D intensity projection methods15 are sufficient real-time rendering streams for both left and right eyes (bottom to unambiguously delineate these complex wiring patterns, left of Fig. 1a), which simulate how a person perceives real-world let alone reconstruct them. objects and thus forms stereo vision. In this way, TeraVR Second, reconstructing the full morphology of a mammalian facilitates efficient immersive observation and annotation (Fig. 1b neuron relies on effectively managing and streaming huge whole- and Supplementary Movie 12) of very large-scale multi-dimen- brain imaging datasets. The volume of a typical mouse brain is sional imaging data, which can have multiple channels or from about 500 mm3, it is not uncommon that a neuron
Details
-
File Typepdf
-
Upload Time-
-
Content LanguagesEnglish
-
Upload UserAnonymous/Not logged-in
-
File Pages9 Page
-
File Size-